Thesis
An average US adult listens to digital audio for ~1.5 hours a day. Meanwhile, 47% of Americans pay for a digital audio subscription, and the share of digital listening taken up by spoken-word audio increased by 40% from 2014-2021. Adopting new voice-enabled products was accelerated by the COVID-19 pandemic and driving demand for natural language processing (NLP) powered understanding of the transcribed text. Audio represents vast untapped value within the enterprise – 80-90% of enterprise data is unstructured data such as audio, voice, and text.
Cheap and accurate speech recognition has increased the demand for audio content. In 2017, Google AI-powered speech recognition claimed 95% accuracy, the threshold for matching human-level accuracy. The same year, Google and AWS launched speech recognition APIs. Developing and deploying ML models for audio transcription takes time and resources most companies don’t have. The US transcription market is expected to reach $41.9 billion by 2030.
AssemblyAI offers APIs to transcribe and understand audio data. It converts audio files and live audio streams to text. It aims to allow users to do more with audio intelligence, like summarization, content moderation, topic detection, and more with its API. AssemblyAI also intends to build on the latest AI research to offer production-ready, scalable, and secure AI models through an API.
Founding Story
Dylan Fox (CEO) founded AssemblyAI in 2017 after having worked at Cisco for the previous 2 years. While at Cisco, Fox had been researching prototypes for machine learning in collaboration products and started looking at Automatic Speech Recognition APIs. Fox found that existing APIs were either inaccurate or inaccessible; for example, when Fox was trying to get access to an API for Nuance, a legacy incumbent in the speech recognition space, the company sent him a CD-ROM with trial software (Fox noted that he didn’t even have a CD-ROM drive on his laptop at the time). Fox also reached out to Google when it had just released its speech API and found that even with though he was representing Cisco, he couldn’t get to support, understand its roadmap, or figure out what aspects of the product might be deprecated in six months.
Fox also dove into ML for speech recognition and discovered that new ML approaches had unlocked accuracy breakthroughs. In 2015, Google announced the adoption of new deep neural networks for voice search, replacing the 30-year-old industry-standard Gaussian mixture model. This kicked off a period of accelerated innovation in the space. In 2017, Google and AWS launched speech recognition APIs and Microsoft announced it had achieved a 5.1% error rate with a speech recognition system used in Cortana, a presentational translator, and Microsoft’s Cognitive Services.
Meanwhile, developer-first companies like Twilio and Stripe were seeing traction with a focus on developer experience. Fox recognized that ML advances allowed new players to come in with a better product and that incumbent players offered a subpar developer experience, which led to the idea for AssemblyAI. He has stated that the “company’s goal is to research and deploy cutting-edge AI models for NLP and speech recognition, and expose those models to developers in very simple software development kits and APIs that are free and easy to integrate.”
Product
Core Transcription
Transcription is AssemblyAI’s foundational offering. Conformer-1 is AssemblyAI’s speech recognition model trained on 650K hours of audio data. It allows transcription of both pre-recorded audio and live audio streams. It supports the automatic detection and labeling of up to 10 unique speakers. Users can boost custom terms to increase their likelihood of being transcribed. It can also detect the number of speakers in an audio file. Each word in the transcribed text is automatically associated with its speaker. As of April 2023, AssemblyAI supports 12 languages (including accented English). AssemblyAI claims industry-leading accuracy and robustness on its core speech recognition model, citing 43% reduced errors on noisy data on average.
Audio Intelligence
AssemblyAI offers models to summarize speech, detect hateful content, and more. It has custom models for different use cases: informative, conversational, or catchy. Informative is best for a single speaker and conversational for two-person conversations. Different models support different summary lengths – for example, catchy can provide a headline or gist, which summarizes a single sentence or a couple of words.
AssemblyAI can identify logical “chapters” in the audio, segments them, and summarizes them into a “chapter.” Demo applications in this area include breaking down podcasts into bite-sized segments, making them more searchable and easier to sample.
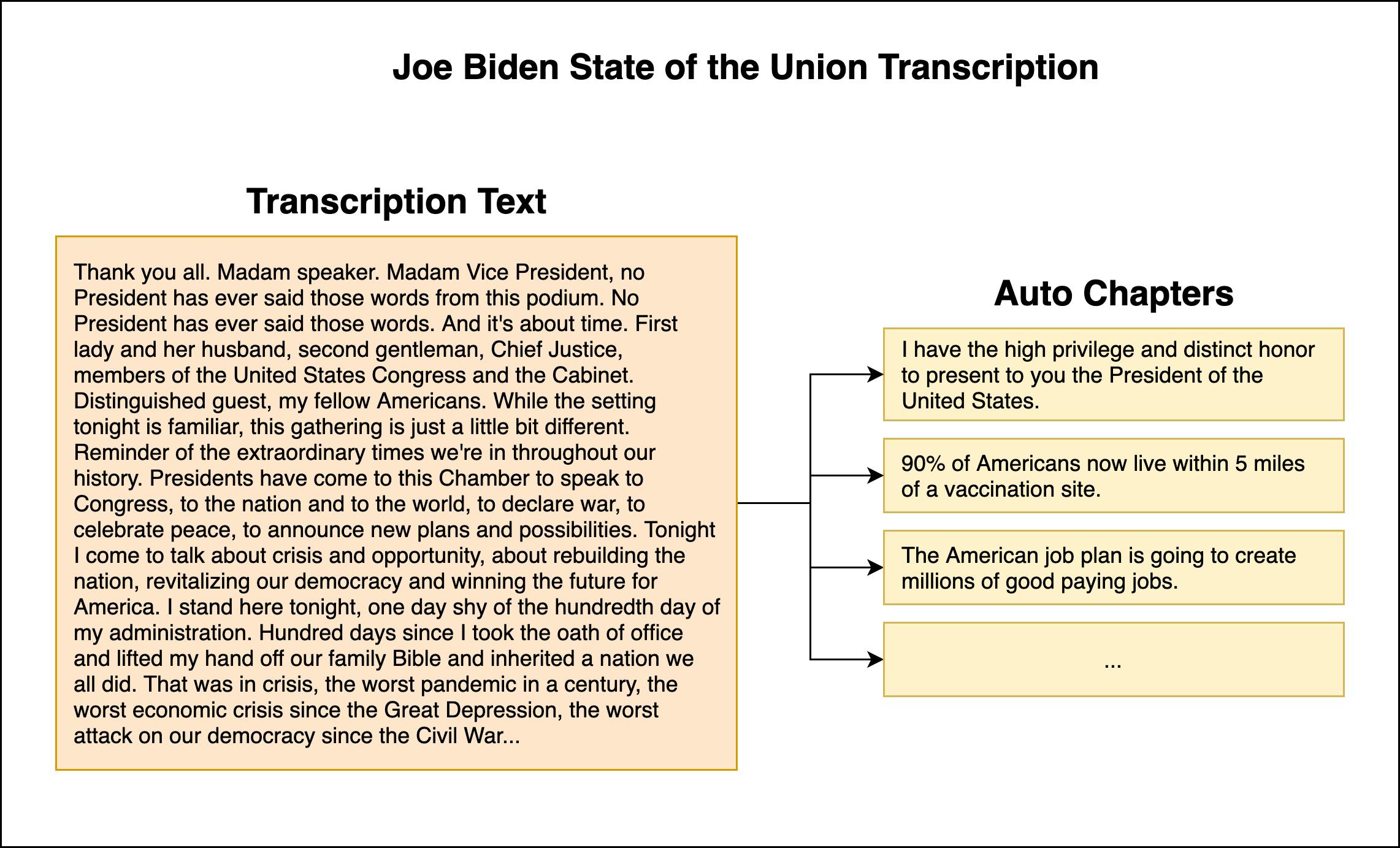
Source: AssemblyAI
PII Redaction increases security and reduces the risks organizations face. AssemblyAI offers 15+ redaction policies. For example, it can automatically redact things like medical conditions, date of birth, credit card, religion, and political affiliation. AssemblyAI provides an example of an audio clip that is automatically redacted with this feature in the following image.
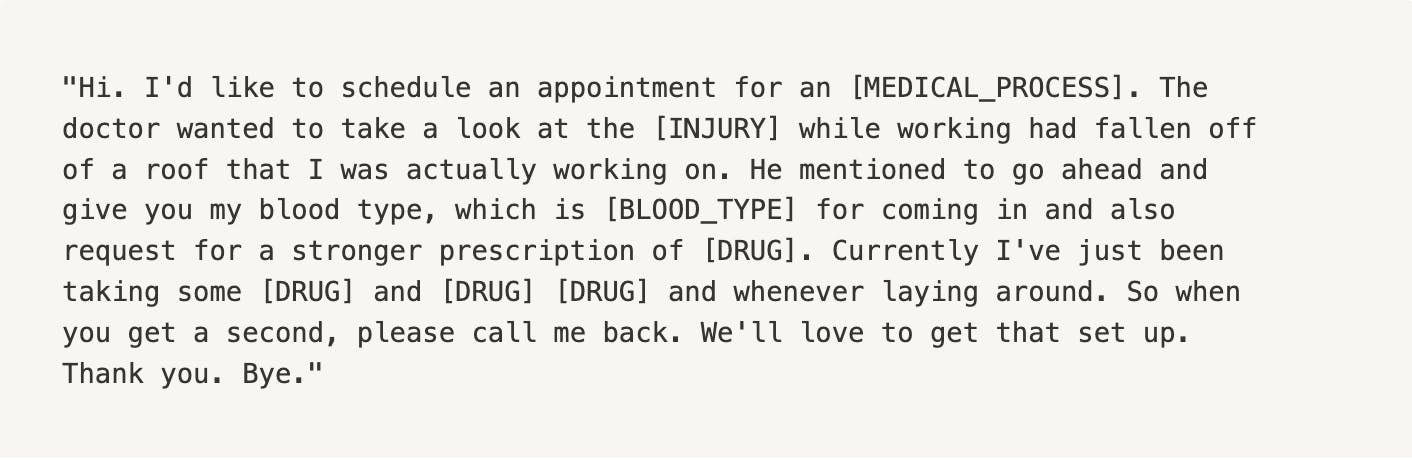
Source: AssemblyAI
Users can also detect and pinpoint when sensitive issues may be discussed, such as crime, drugs, health issues, etc. AssemblyAI can detect the sentiment of sentences in audio files. Users can identify entities in audio files, such as names, email addresses, dates, and locations.
Market
Customer
AssemblyAI’s developer-first approach meant many initial customers were startups and SMBs adopting voice features. However, AssemblyAI has a foothold in the enterprise, with customers like WSJ, NBC Universal, and Spotify. AssemblyAI is investing in its upmarket motion, evidenced by the announcement of the Enterprise offering on September 2022. AssemblyAI is used by Veed's automatic captioning system, Spotify's advertising platform, and CallRail's call tracking platform. AssemblyAI has healthcare-specific features allowing it to identify medical processes, conditions, blood types, drugs, and injuries and redact them from transcripts. Its advanced Topic Detection targets customers that handle podcasts, videos, and other media, where understanding the topics can help with advertising, recommendations, and search.
Accuracy is the most important factor in choosing an ASR (Automatic Speech Recognition) provider, but it can vary widely depending on the customer's use case. For example, TED talks are relatively easy, whereas Zoom meetings where there are multiple speakers potentially talking over each other with varying audio quality are more difficult. For ASR customers, accuracy is the most important factor in choosing a provider. However, while off-the-shelf ASR models are hitting 90+% accuracy in easier use cases, improving models for complex use cases remains a challenge and provides significant value. AssemblyAI aims to distinguish itself in difficult cases, claiming 43% fewer errors on noisy data.
Customers of ASR also want to go beyond transcription and understand transcribed audio through NLP. Whereas with other providers customers may have to stitch together an audio to text to NLP workflow, AssemblyAI has a tightly integrated Audio Intelligence offering. For example, AssemblyAI announced healthcare-specific features allowing it to identify medical processes, medical conditions, blood types, drugs, and injuries — and redact them from transcripts.
However, it isn’t easy to generalize NLP across use cases and specialized verticals often require customized solutions. A 2021 survey found that the top users of NLP are health care, technology, education, and financial services. The most important NLP features to these users included named entity recognition and document classification, which vary widely from vertical to vertical.
Market Size
The text-to-speech market was valued at $2.8 billion in 2021 and is estimated to reach $12.5 billion by 2031. However, AssemblyAI’s Audio Intelligence more broadly targets the NLP market, estimated at $26.4 billion in 2022 and projected to grow to $161.8 billion by 2029, representing a CAGR of 18.1%. Cheap, accurate speech recognition has also unlocked demand for existing voice audio. A wide range of industries are turning to AI-powered solutions to make audio legible to machines, spanning call centers, financial services, legal, telecom, education, healthcare, and more. Within the enterprise, audio represents a vast amount of untapped value – roughly 80-90% of enterprise data is unstructured data such as audio, voice, and text. AssemblyAI’s core transcription service targets the speech and voice recognition market, which was valued at $13.8 billion in 2021 billion in 2023 and is projected to grow to $48.1 billion by 2030, representing a CAGR of 14.9%.
Competition
New players
Deepgram: Founded in 2015, Deepgram provides developers with a Speech-to-Text API. It has raised $86 million in funding. As of November 2022, Deepgram had over 300 customers and more than 15K users. In contrast to AssemblyAI’s developer-first, API-only approach, Deepgram started focusing on the enterprise, building solutions that customers can deploy on-prem. A marquee customer is NASA, where Deepgram built Space to Ground communications models. Deepgram is pushing into the startup and SMB market, announcing a $10 million startup program on August 3, 2021, along with a revamped developer experience including improved documentation, SDKs, and developer consoles. Deepgram claims faster processing speeds than AssemblyAI and greater multi-channel support. Deepgram competes head-to-head with AssemblyAI, offering an API for Automatic Speech Recognition and Conversational Intelligence.
Speechmatics: Founded in 2006 and based in the UK, Speechmatics is one of the earliest startups in Automatic Speech Recognition. Speechmatics founder Dr. Tony Robinson conducted AI Research at Cambridge before spinning out Speechmatics. It has raised $72 million. As of June 2022, it had 170 customers. Speechmatics aims to differentiate itself through its support of a broader range of languages and accents. For example, as of April 2023, AssemblyAI supports 12 languages whereas Speechmatics supports 34 languages. CEO Katy Wigdahl states “what we have done is gather millions of hours of data in our effort to tackle AI bias. Our goal is to understand any and every voice, in multiple languages.”
Incumbents
Nuance and its flagship Dragon speech recognition product is the dominant incumbent provider in the space, with its speech recognition service having been confirmed to be powering Apple’s Siri service starting in 2013. Dragon, developed in 1997, was acquired by Nuance, and Nuance expanded Dragon from desktop software to targeted Medical and Legal solutions. Microsoft acquired Nuance in 2021 for $16 billion and continues to invest in Nuance’s products in healthcare. For example, on March 20, 2023, Microsoft announced Dragon Ambient Experience Express, a clinical notes application powered by GPT-4.
IBM Watson Speech-to-Text, Google Speech-to-Text, AWS Transcribe, and Azure Cognitive Services Speech-to-Text are other large providers in this space. Large cloud providers typically offer more languages – for example, Google offers 125 languages and vertical-specific solutions, such as AWS Transcribe Medical. However, large providers typically lack customization and are slow to address bias in their models. As a Principal Director of Data, AI, & IOT Solution Architect at Microsoft noted, “With Microsoft or any big cloud providers if you're not a big enterprise Fortune 50 company that is paying millions of dollars or hundreds of thousands of dollars every week, you don't have access to the engineering group to be able to do all of this.”
This lack of fine-tuning may also cause problems with bias. For example, a 2019 study by Stanford tested speech-to-text offerings from Amazon, Apple, Google, IBM, and Microsoft and found discrepant average error rates among different ethnicities. The developer experience is also worse, typically requiring users to stitch together multiple services to leverage NLP-powered solutions like Speaker Diarization (separating individual speakers) and Named Entity Recognition. In contrast, startups such as Deepgram and AssemblyAI unify these services in a single interface.
Open Source / In-House
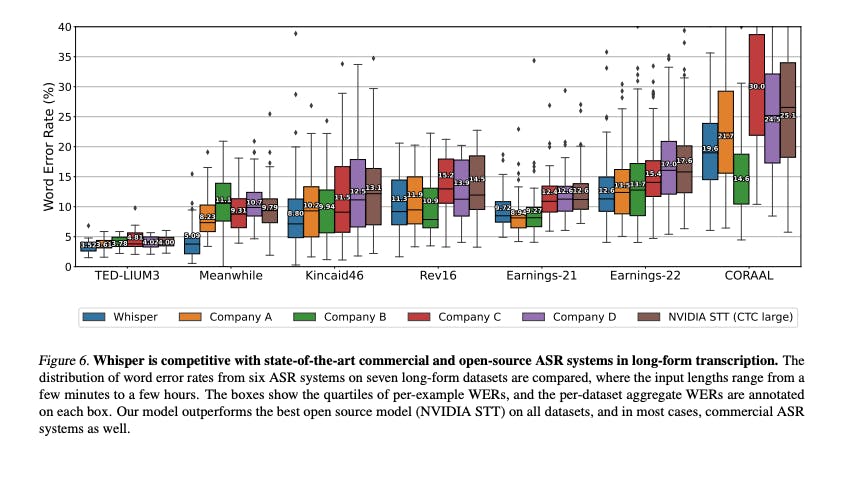
Source: OpenAI
Open-source models are competitive with existing commercial solutions on accuracy. For example, a creator media startup, Captions, chose OpenAI’s Whisper over Google’s Speech-to-Text Solution. However, latency and scaling remain challenging with open-source model hosting. Training and deploying an ML model requires specialized infrastructure and expertise. For companies with engineering resources, open-source models can jump-start proprietary models. The open nature of ML research also means that many architecture breakthroughs, like Google’s Transformer and Facebook Wave2Vec, are published openly and can direct internal research efforts. A machine learning engineer using Deepgram at Observe.ai, an AI contact user solution, said that it was considering building its own speech recognition model with a team of 7 engineers after spending north of $1 million with Deepgram.
Business Model
AssemblyAI offers a usage-based pricing model, charging per second for Core Transcription and Audio Intelligence. As of April 2023, AssemblyAI charges $0.00025 per second for Core Transcription and $0.000583 per second for Audio Intelligence. AssemblyAI charges custom pricing for enterprise features.
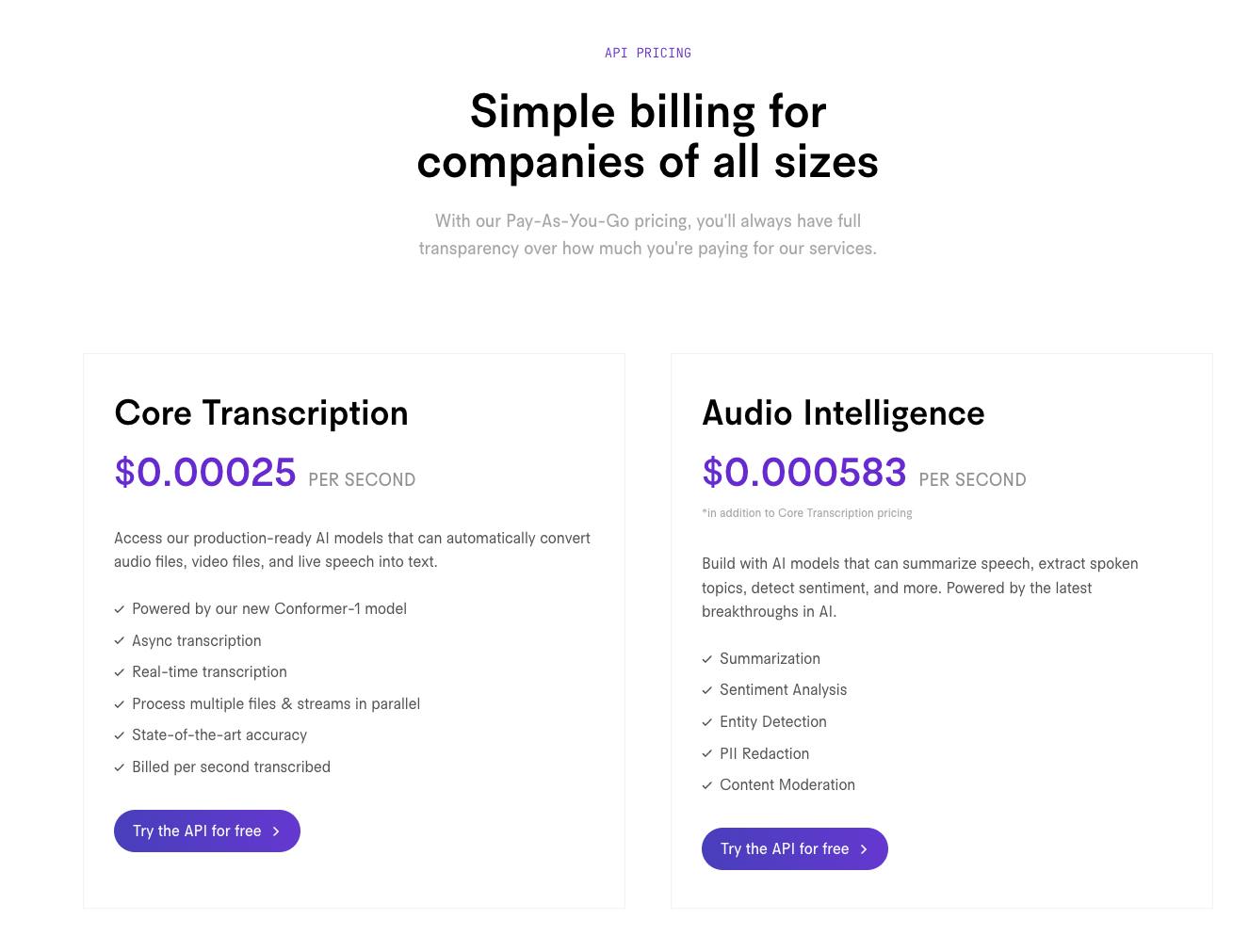
Source: AssemblyAI
Traction
From the start of 2022 to July 2022, AssemblyAI’s revenue and user base multiplied 3x. It reportedly has “hundreds” of paying customers and more than 10K users. AssemblyAI also has been recognized as a high performer and momentum leader on G2 in the Voice Recognition software category four times (Summer 2022, Spring 2022, Winter 2022, and Winter 2021. AssemblyAI has a rating of 9.9 for Ease of Use (industry average 8.3) and 9.5 for Quality of Support (industry average 8.3).
Valuation
In July 2022, AssemblyAI raised a $30 million Series B at an undisclosed valuation. The round was led by Insight Partners, with Accel and Y Combinator participation. Accel previously led the $28 million Series A round, in March 2022. Its total funding raised is $63.1 million.
While AssemblyAI had “barely dipped” into its Series A funding, its fast-follow funding was intended to accelerate its AI development and establish AssemblyAI as a dominant AI provider. As CEO Dylan Fox stated during the Series B announcement: “We plan to 3x our AI research team over the next six months and invest millions of dollars into GPU hardware to train larger and more complex AI models that will push the envelope.”
Key Opportunities
Accelerating ML Research Driving Product Innovation
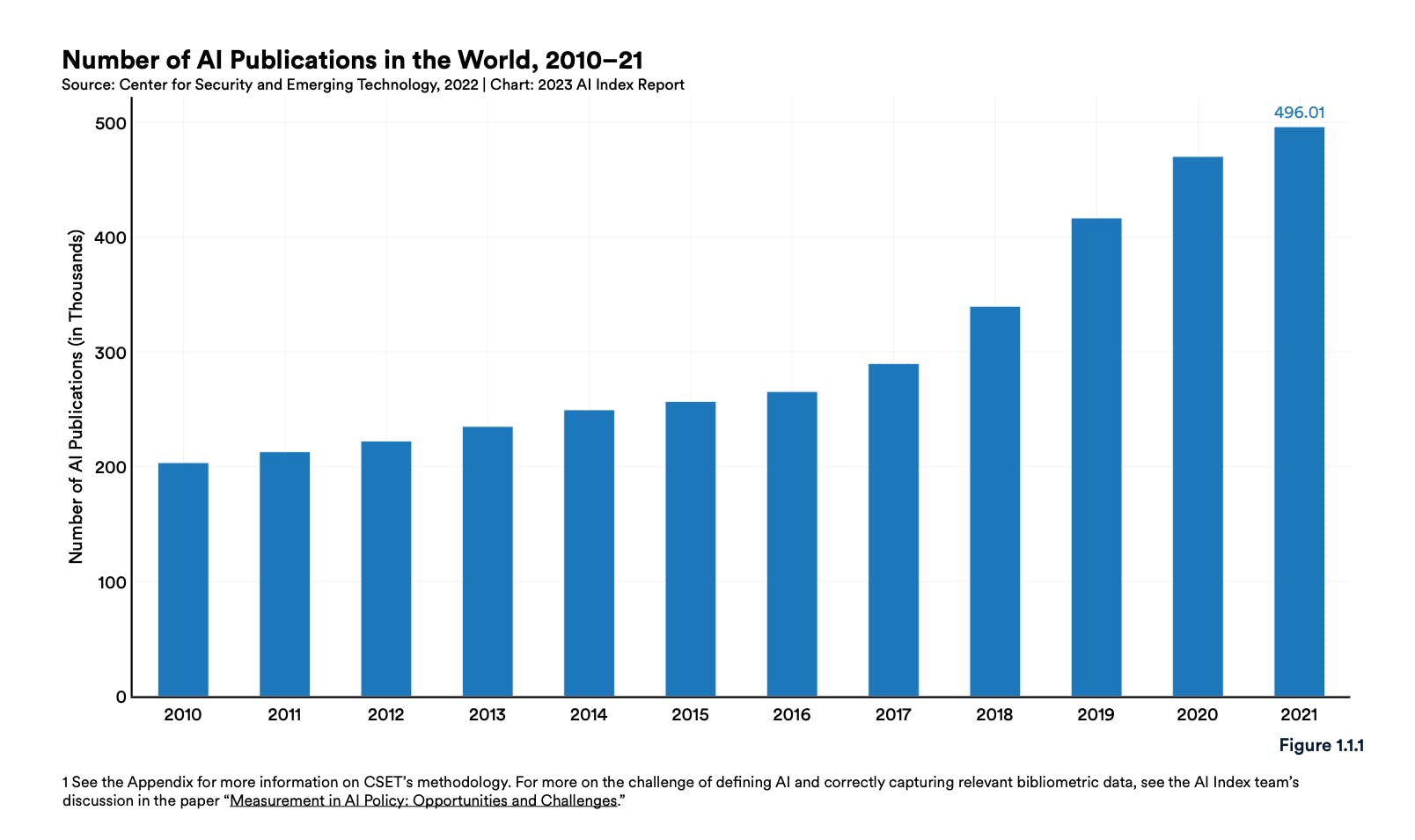
Source: Stanford
The LLM arms race and accelerating AI research more broadly fuels AssemblyAI’s product innovation. Teams like AssemblyAI can incorporate and commercialize the latest research. As Dylan Fox, CEO of AssemblyAI, has noted:
“Many of our customers choose to integrate with our API for that very reason - our speed and rate of improvement. It’s not out of the norm for our team to push model updates on a weekly basis.”
LLM improvements unlock new capabilities for NLP, which directly helps AssemblyAI improve and expand its value-added audio intelligence product. Accelerating AI research may also help AssemblyAI improve its core transcription service. For example, AssemblyAI’s latest automatic speech recognition model, Conformer-1, adopted a new neural net architecture, conformers, first published by Google Brain in 2020. Conformers evolved from the transformer architecture, first published in 2017, that powers ChatGPT.

Source: The Economist
Growing Usage of Voice-Enabled Products
Innovation in voice-enabled products is driving usage. AssemblyAI, with its developer focus and classic product-led growth, is positioned to capture new voice products and grow with existing customers. For example, CallRail, a startup that delivers inbound call analytics to SMBs, partnered with AssemblyAI and “improved its call transcription accuracy by up to 23% and doubled the number of customers using its Conversation Intelligence product.” The key buyer for AssemblyAI is its end-users – developers and product teams. AssemblyAI invests heavily in developer relations to grow end-user advocacy and inspire new use cases. AsssemblyAI’s research team runs a YouTube channel on AI with 22.8K followers and 1.2 million views, and in 2022 AssemblyAI published over 110 articles on AssemblyAI’s blog, including tutorials, AI breakdowns, announcements, and more.
Key Risks
High Research Costs and Risks
Developing models for audio is more challenging than text, given its high dimensionality. AssemblyAI is investing heavily in AI research and development, with CEO Dylan Fox stating in July 2022 that he plans to “3x our AI research team over the next six months and invest millions of dollars into GPU hardware to train larger and more complex AI models that will push the envelope.” Costs may continue to rise as models become larger and data requirements grow. As CEO Dylan Fox noted, the long training times and experimentation inherent in developing ML models meant that for the first two years, AssemblyAI’s main focus was R&D.
Challenges Pushing Upmarket
Pushing upmarket may require a different sales motion to execute successfully. AssemblyAI is taking steps in this direction with its enterprise announcements in September 2022, but it remains to be seen if it can execute successfully. As of April 2023, AssemblyAI doesn’t offer on-prem solutions, a common requirement for buyers handling sensitive data such as government or healthcare.
Enterprise buyers in automatic speech recognition also have high customization and accuracy requirements. Furthermore, vertical-specific ASR solutions are highly competitive and have established players bundling in ASR to existing product suites. For example, AssemblyAI announced medical-specific features in May 2021. However, with its complicated terminology and sensitive data, healthcare has players such as Microsoft acquired Nuance, and Amazon Alexa. As part of its $28.3 billion acquisition of EHR system Cerner, Oracle announced that it plans to add a “hands-free voice interface to secure cloud applications.” Overcoming such incumbents could prove challenging.
Summary
Voice-enabled features are rapidly becoming consumer and enterprise products, driving demand for speech transcription. However, existing automatic speech recognition and NLP services from cloud providers like Nuance and IBM can be difficult to use and fail to support smaller customers.
AssemblyAI aims to offer a Stripe or Twilio-like API for transcription and value-added NLP features such as topic detection. It has seen early traction, tripling its revenue and user base from the start of 2022 through July 2022. To keep up with the accelerating rate of AI progress, AssemblyAI invests millions in research and training models. AssemblyAI will need to adjust as it expands its product offering and push upmarket.