Thesis
As artificial intelligence progresses, it is likely to transform many aspects of the economy. Through natural language processing, machines can comprehend and respond to human language. Computer vision enables machines to perceive and interact with the environment. Enterprise adoption of AI is a growing trend, with 63% of businesses expecting to accelerate AI investment in a market where enterprises spent $51 billion in 2022. The core of this transformation is using data to generate insights and make predictions. However, 87% of data science projects never make it into production. One of the most common factors in this failure is the need for more relevant data.
Data scientists and domain experts spend over 80% of their time assembling and managing training data. As the infrastructure and models for AI become more widely accessible, training data has become the critical determinant of whether an AI project succeeds or fails. Architectural advancements and computing innovations can only take companies so far if the training data remains a major hurdle. The challenge and opportunity lie in understanding, labeling, augmenting, and managing the data. The global data labeling solution and services market is expected to reach $38.1 billion by 2028.
Snorkel AI is the data platform for enterprise AI, enabling customers to build and iterate from unlabeled data sets to machine learning models deployed in production. Instead of relying on manual, hand-labeled data, users are able to programmatically label and manage data. This is done by writing functions to express rules that make it easy for subject-matter experts to map their expertise to ML solutions. Snorkel AI’s product Snorkel Flow trains, deploys, analyzes, and monitors models and workflows on top of this training data. Users can improve and adapt these models by editing programmatic training data.
Founding Story
Snorkel AI started as a research project in the Stanford AI Lab in 2015. The founders, Alexander Ratner (CEO), Chris Ré, Henry Ehrenberg, Braden Hancock, and Paroma Varma, set out to explore new interfaces for machine learning. As a part of the founding team, Ré brought a history of successful research spinouts to Snorkel AI. As an associate professor of Computer Science and MacArthur Genius award winner, he helped develop DeepDive, which became Lattice Data, a company acquired by Apple. Ré also founded SambaNova Systems, which reached a $5 billion valuation in 2021. Prior to founding Snorkel AI, the founding team spent over half a decade researching programmatic labeling, weak supervision, and other techniques to break through one of the biggest bottlenecks in AI: the lack of labeled training data. That research resulted in the Snorkel research project and 60+ peer-reviewed publications.
The Snorkel AI team found that AI practitioners and academics needed help with a critical requirement: assembling training data. They saw domain experts like radiologists tasked with labeling cases one by one, such as classifying X-rays, in an expensive and time-consuming process. The team developed a different approach – allowing users to label data programmatically. That led to a human-in-the-loop flow which enabled experts to supply higher-level rules on how they labeled data, as opposed to generating all the labels themselves. The team deployed early versions of the new approach in Gmail, Youtube, Apple, Intel, and the Departments of Justice and Defense. However, users were increasingly asking for help with the lifecycle of building, deploying, and managing ML systems. In 2019, the research team spun out of the AI Lab and created the company Snorkel AI to meet the demands of a data platform.
Product
Snorkel Flow
Snorkel AI’s core product is Snorkel Flow. It enables customers to build and iterate from unlabeled, unstructured datasets to deploy high-quality ML models between “10-100x” faster than other techniques. Users, instead of manually labeling data, can programmatically label data using “labeling functions,” which encode rules or heuristics that express the domain knowledge of subject matter experts. Snorkel Flow uses this programmatic training data to train, deploy, and monitor models. While similar to a traditional ML workflow, Snorkel’s focus on programmatic training data allows users to iterate on each step quickly and flexibly, enabling improvements within hours or days instead of weeks and months.
Snorkel Flow is powered by half a decade of research from the Snorkel Project, which demonstrated even noisy labeling functions could be aggregated to generate high-quality labels. This approach, called Weak Supervision, has the following benefits versus manual labeling:
Scalability: A labeling function can label millions of data points without additional human effort.
Adaptability: Product requirements change, new input data is added, and unexpected errors can appear. Manual labeling would require re-reviewing affected data points individually, multiplying the cost in time and money. With programmatic labeling, users can add or modify a targeted number of labeling functions and re-execute.
Explainability / Governability: Manual labeling makes it difficult to audit why a label was provided, affecting quality control, safety, and compliance. Programmatic labeling ties each label back to a function so that functions can be removed or modified if bias is detected.
Snorkel Flow makes ML model development more similar to software development than it otherwise would be. Software developers reuse and modify code and build on existing frameworks. Snorkel Flow provides users with an easy way to apply existing knowledge bases, legacy systems, and domain knowledge to bootstrap training data. Software developers iterate rapidly based on product feedback. Similarly, Snorkel Flow makes it easy to understand gaps or errors with current ML models and allows users to regenerate labels faster.

Source: Snorkel AI
Data Labeling
Instead of asking domain experts to provide individual labels, users can distill their intuition as labeling functions that are applied at scale. Snorkel Flow can capture various signal sources for labeling, including keywords, patterns, database lookups, and external models. Labeling functions (LFs) let users encode domain knowledge without worrying about every edge case. Snorkel Flow reconciles and applies imprecise and conflicting labeling functions to auto-label training datasets. Users can write labeling functions in no-code UI or using the SDK.
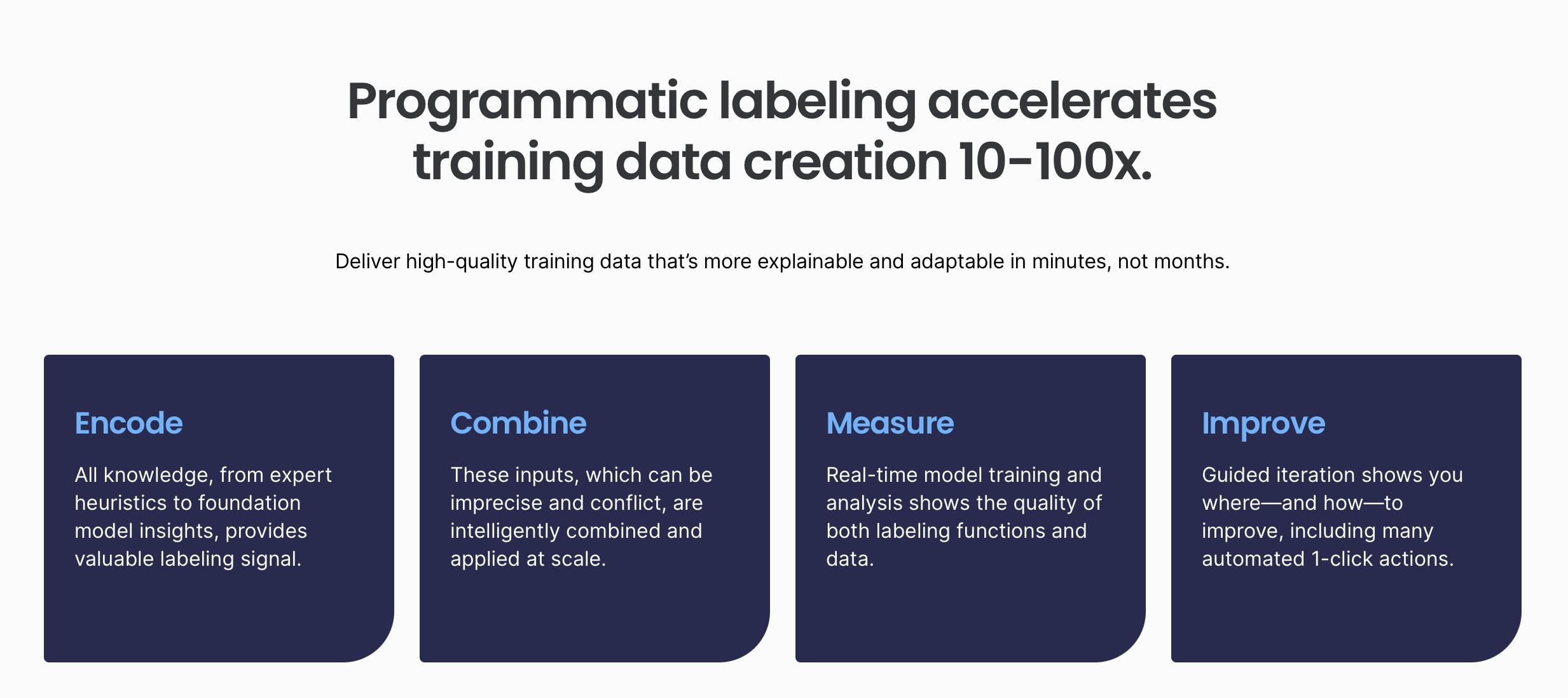
Source: Snorkel AI
Model Training & Analysis
As users label their data, Snorkel Flow automatically retrains models to provide real-time analysis of the model and the training data quality. It also auto-generates suggestions. Users can train model architectures without code or use the Python SDK to train custom models on their infrastructure and return predictions to Snorkel Flow for analysis. In Snorkel Flow, users have the flexibility to build more than a model — they can also combine pre- and post-processing operators, models, and business logic, as well as gain visibility into each step of the pipeline to optimize end-to-end application quality.
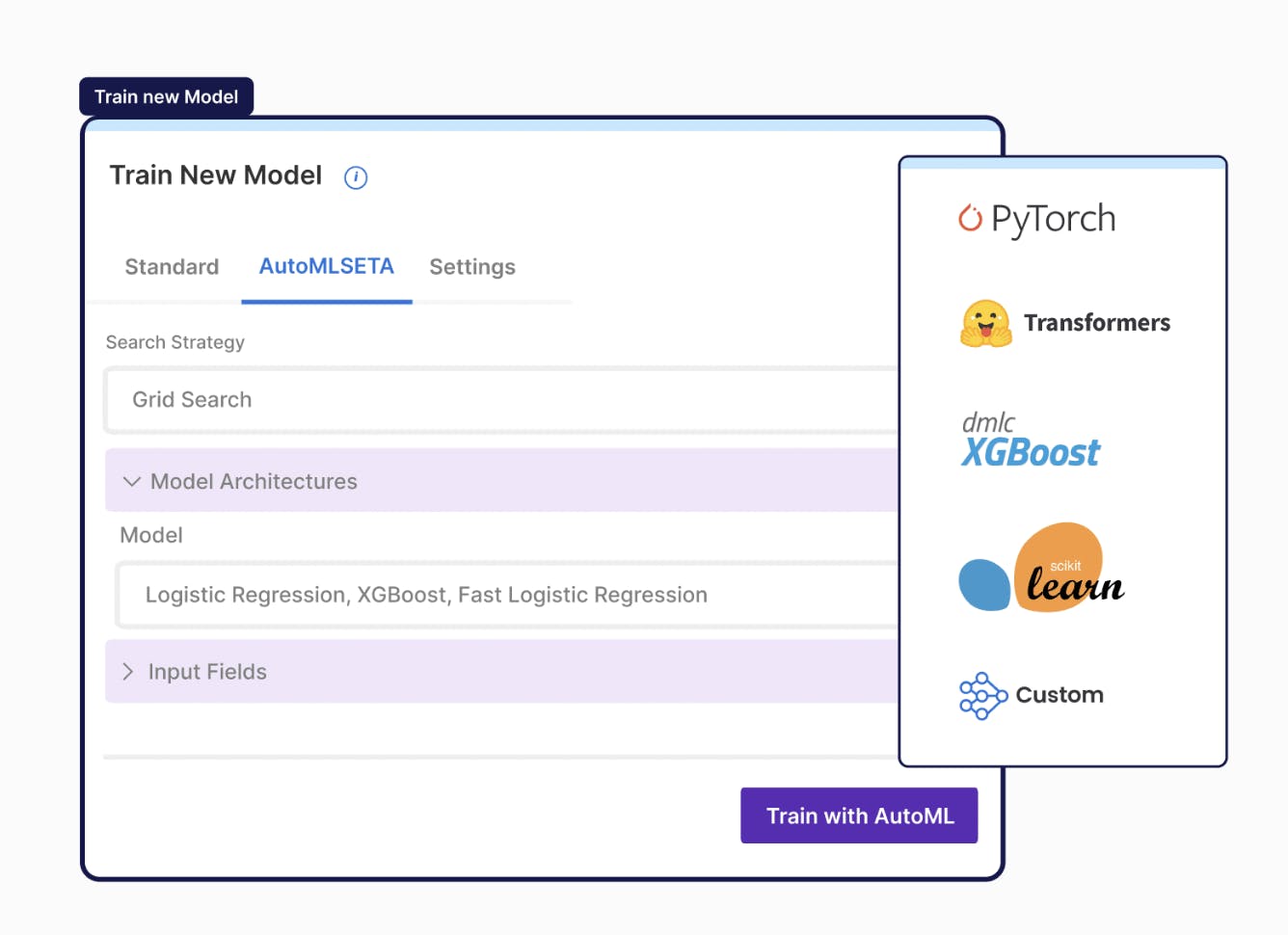
Source: Snorkel AI
Enterprise
Snorkel Flow works with a user’s existing ML stack of enterprises in a secure manner. Users can build a data-centric workflow over their infrastructure, data sources, modeling libraries, serving environments, and more.
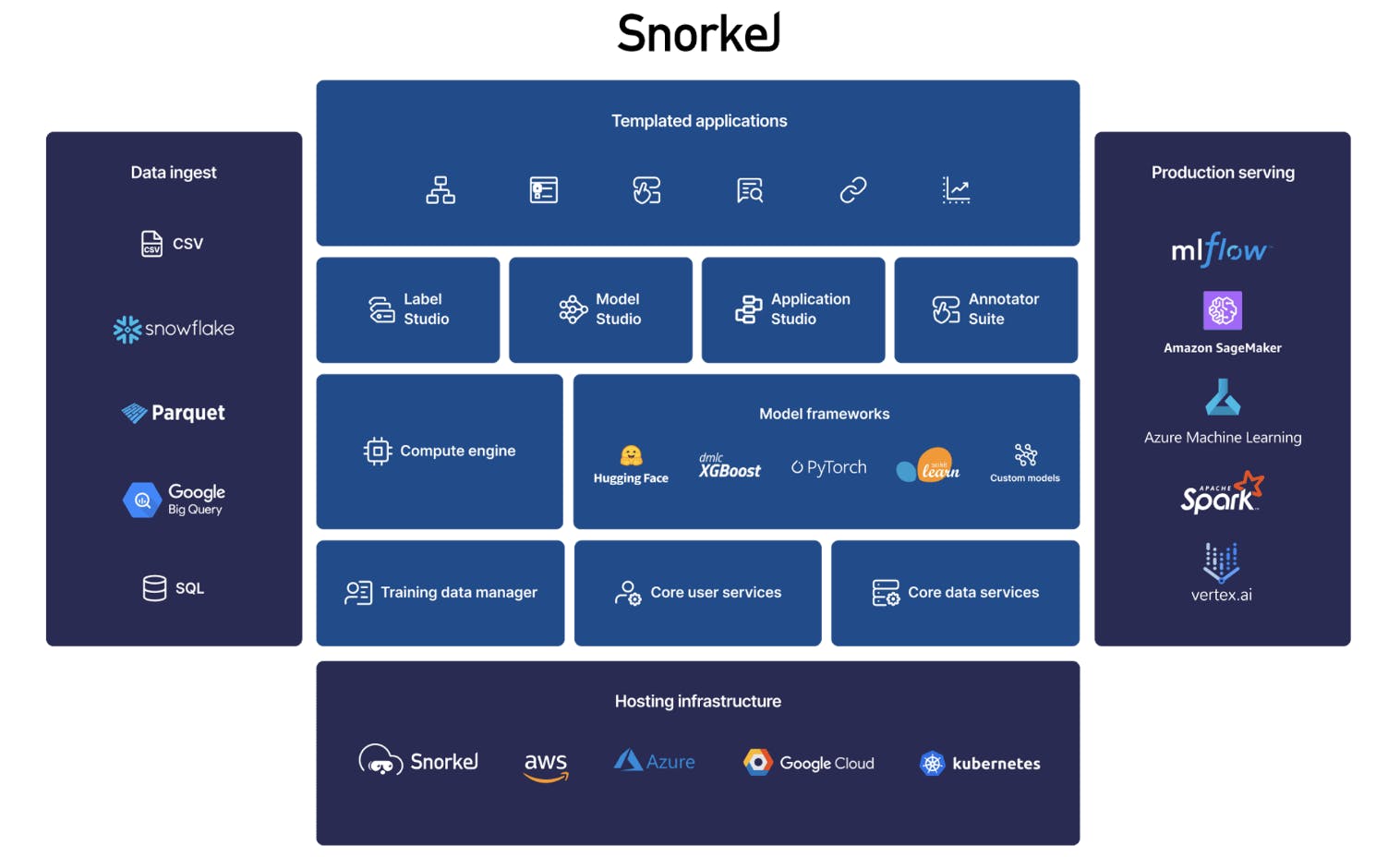
Source: Snorkel AI
Foundation Models
Snorkel Flow’s Foundation Model Development aims to overcome adaptation and deployment challenges currently blocking them from adopting foundation models to accelerate AI development. It gives enterprises the tools they need to put foundation models to use for complex, performance-critical use cases and create large, domain-specific training datasets to fine-tune and adapt foundation models for enterprise use cases with production-grade accuracy.
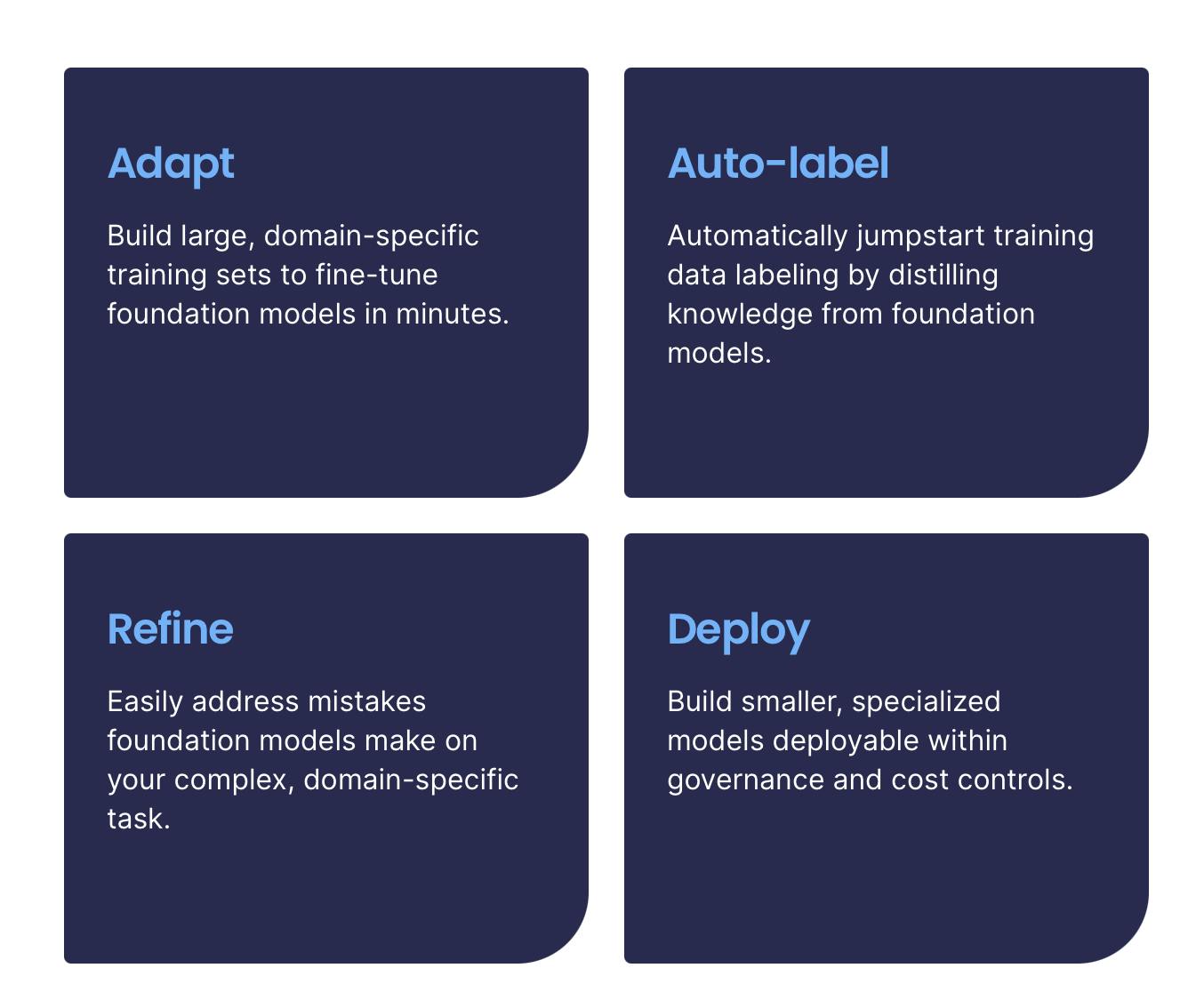
Source: Snorkel AI
Market
Customer
Snorkel AI’s customer profile is typically large enterprises deploying AI applications. It is used across banking, healthcare, the public sector, and insurance industries. It has many use cases, including document classification, information extraction, sentiment analysis, conversational AI, and named entity recognition. Its customer base includes Apple, Intel, Tide, Uber, LinkedIn, BNY Mellon, Stanford Medicine, Google, consulting firms, and more.
However, the company targets use cases where Snorkel AI can provide the biggest improvement over existing approaches – typically where data is unstructured and labeling is expensive and difficult. An estimated 80% of enterprise data is unstructured, such as text, video, image, network logs, PDFs, websites, etc. Snorkel AI tames this “messy long-tail data” by helping users generate 100K “pretty good labels” vs. 100 “perfect” labels. Snorkel AI thus unlocks new, data-hungry models, often showing
Large training data sets can be difficult and expensive to label and relabel as requirements change. Simple problems such as “find the stop sign” can be outsourced once, but Snorkel AI gains an edge when data is private, requires domain expertise, or changes often. Use cases include classifying private legal documents or extracting information from custom financial contracts in finance, insurance, healthcare, and government industries. Snorkel AI also fits cases where model objectives constantly change, such as network security and monitoring.
Market Size
Snorkel AI’s core product, programmatic labeling, targets the data labeling market. This was estimated to be worth $5 billion in 2022 and was projected to grow to $22 billion by 2027. Meanwhile, the global enterprise artificial intelligence market was valued at $11.4 billion in 2021 and is expected to grow at a CAGR of 34.6% from 2022 to 2030. As an increasing number of organizations embrace AI, the need for Snorkel AI’s product is likely to increase because labeling training data is one of the integral steps in training a machine learning model.
Competition
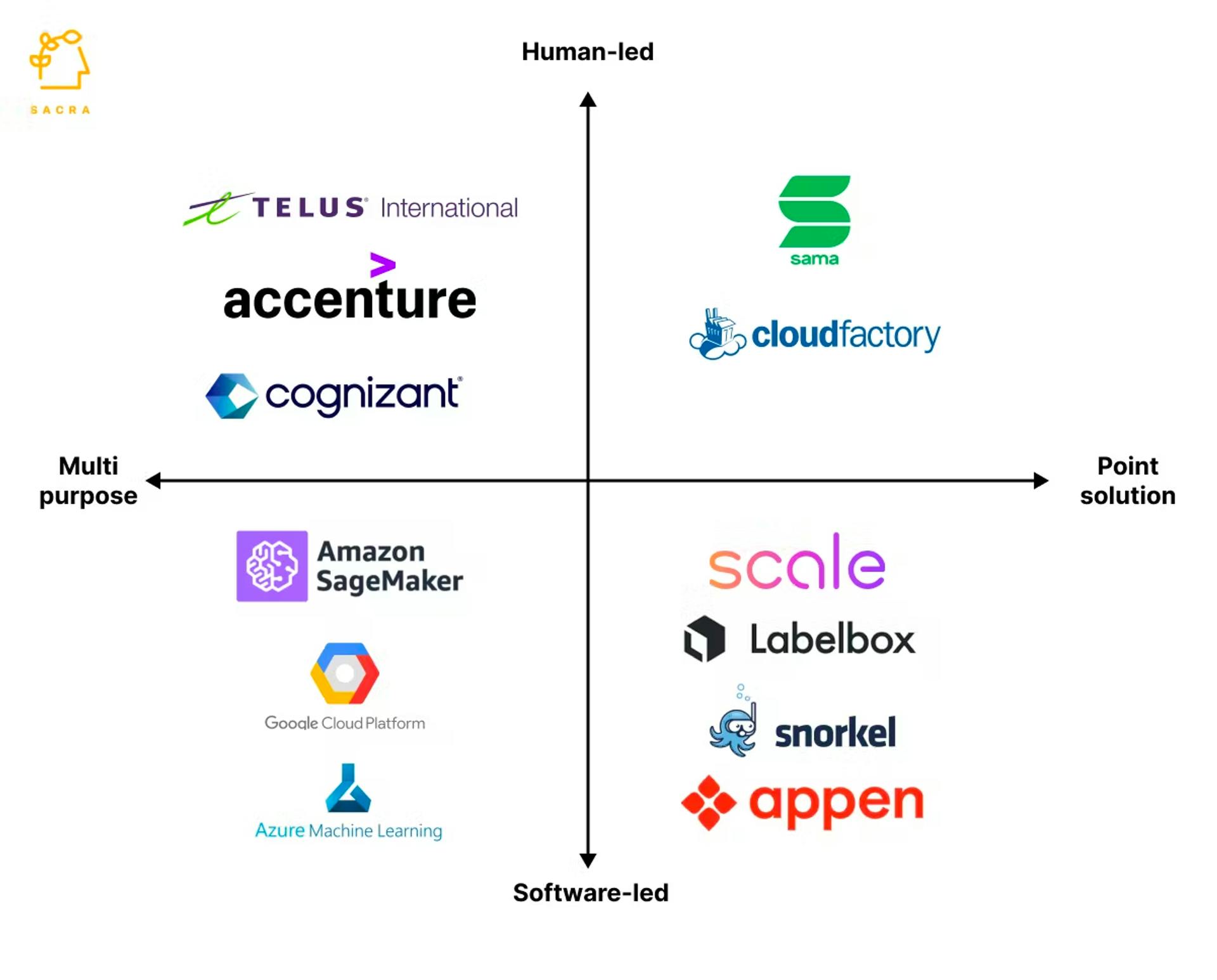
Source: Sacra
Labelbox: Labelbox, founded in 2018, is a data training platform that creates and manages labeled data for machine learning applications. Like Snorke Al, 80% of Labelbox’s business in 2021 came from enterprises and Labelbox’s software platform targets enterprises that aim to facilitate the training data iteration loop that improves ML performance. However, LabelBox approaches the labeling problem from a different angle as it was one of the first to offer model-assisted labeling automation. Rather than subject matter experts writing their own labeling functions, LabelBox offers proprietary ML-powered pre-labeling. LabelBox also offers robust tooling around managing widely distributed annotation teams, including a new product, Boost, that offers outsourced labeling expertise. LabelBox’s founders also claim they do not compete with Scale AI or Snorkel AI but are primarily replacing Amazon’s SageMaker and in-house tools. It raised a $110 million Series D in January 2022 at a $1 billion dollar valuation. It operates across retail, manufacturing, entertainment, insurance, and healthcare. Its customer base includes P&G, Burberry, Dialpad, and Ancestry.
Scale AI: Scale AI, founded in 2016, is another data platform for AI, providing training data for machine learning teams. While Scale uses outsourced labor in flagship products such as Scale Rapid, Scale has also expanded to directly target in-house data labeling teams with products such as Scale Studio. Scale AI leverages proprietary ML models to help pre-label data, which could eliminate the need for in-house labeling. As Snorkel AI builds its end-to-end platform, it competes with Scale AI's all-in-one ML platform. It has raised $602 million in funding and was last valued at over $7 billion. It is used across autonomous vehicles, AR/VR, robotics, LLMs, etc. Its customer base includes Flexport, Nuro, OpenSea, and more.
Surge: Surge is another data labeling platform founded in 2020. It has raised $25 million in funding. It is used for financial categorization, content moderation, customer support, and more. Its customers include Twitter, Amazon, NYU, and Twitch.
IT services giants like Accenture and Cognizant also offer fully outsourced data-labeling services and managed data-labeling providers such as Sama and Cloudfactory require minimal internal resources. Cloud providers such as AWS and GCP, where enterprises often host their ML models, could expand their labeling offerings, for example, through Amazon’s Mechanical Turk. AWS launched Amazon SageMaker Ground Truth in 2018 as an automated data labeling service.
Business Model
Snorkel AI has minimal public information on pricing and likely has custom enterprise contracts. As a ceiling for pricing, Snorkel AI claims it drives “seven-to-eight figure ROIs,” including a large biotech firm that saved an estimated $10 million on unstructured data extraction.
Traction
Snorkel AI announced triple-digit customer growth in 2022, having acquired notable customers including 5 out of the top 10 US banks, government agencies, and Fortune 500 companies across financial services, insurance, pharma and healthcare, manufacturing, and retail industries. Earlier versions of Snorkel AI’s core technology were deployed in production at the most sophisticated technology companies, such as Apple and Google. Its customer base includes Intel, Tide, Uber, LinkedIn, BNY Mellon, Stanford Medicine, Google, and consulting firms.
In 2022, Snorkel AI Research published over 30 papers at NeurIPS, ICLR, ACL, and more with academic partners at Brown University, Stanford University, the University of Washington, and the University of Wisconsin-Madison. It also deepened partnerships across the enterprise AI ecosystem, including Microsoft Azure AI Services, Google BigQuery, Snowflake, Aimpoint Digital, and more. Snorkel AI also hosts an annual “Future of Data-Centric AI” conference, which claimed in 2022 to be “the first and the largest event to bring together thought leaders from Fortune 500 enterprises, AI/ML startups, and academia to explore data-centric AI approaches that make AI practical.”
Valuation
On August 9, 2021, Snorkel AI announced an $85 million Series C round at a $1 billion valuation. This round was co-led by new investor Addition and previous investor Blackrock. Previous investors Greylock, Lightspeed Venture Partners, Nepenthe Capital, and Walden also participated. The Series C round brought Snorkel AI’s total funding to $135 million.
Key Opportunities
Expanding Industry Verticals and Use Cases
Snorkel AI markets vertical-specific solutions for banking, healthcare, government, insurance, and telecom. These solutions include pre-built templates for common use cases, simplifying sales and accelerating time to value. As more organizations try to leverage unstructured data, Snorkel AI's opportunities expand. Snorkel AI markets case studies of different industries, such as Big 4 consulting firms analyzing news articles, Georgetown researchers analyzing policy recommendations, Schlumberger analyzing oil well reports, and Pixability labeling YouTube videos.
AI advances also allow Snorkel AI to expand into new use cases. For example, as NLP models became more powerful, Snorkel AI expanded from earlier applications such as Document Classification and Named Entity Recognition to areas such as Conversational AI. Comcast used Snorkel AI to create applications powering voice and conversational interfaces, leveraging advanced NLP models such as BERT, and are now exploring large language models.
Foundation Models
Snorkel AI has rapidly incorporated foundation models into its product and marketing, announcing Data-Centric Foundation Model Development in November 2022 and hosting a Foundation Model Summit in January 2023. Enterprises often have to fine-tune foundation models to meet the bar for production-quality predictive AI. Even if this accuracy is achieved, deploying foundation models includes its challenges, including inference costs, latency, governability, and model risk management.
By incorporating foundation models as part of the Snorkel AI process, enterprises can fine-tune and train a smaller, specialist model that’s easier to manage and deploy. As a VP of a publicly traded financial services company has noted:
“We will not take blindly whatever tool gives us because we can be fined billions of dollars. We cannot just say the tool told us to do it. That's why model extendibility, stability bias, and fairness are very important to us. We are willing to sacrifice model performance, accuracy recall, or r-square, whatever is the relevant KPI.”
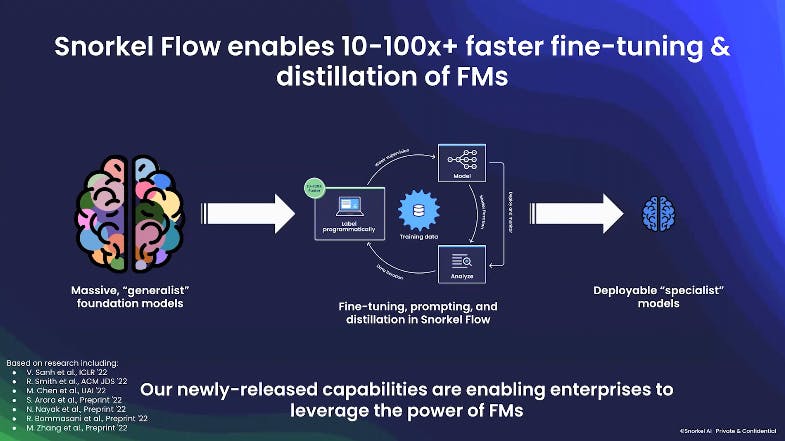
Source: Snorkel AI
Key Risks
Challenges Expanding a Specialized Platform
Expanding usage of its platform may also prove challenging for Snorkel AI. Snorkel AI gains an advantage when the job must be done internally and involves internal domain experts. Simple data labeling, like labeling pedestrians in an image, can be outsourced effectively. Enterprises may not need many customized internal models – as a Snorkel AI customer at a publicly traded financial services notes:
“I envision the next five years, there will be… more need for a tool like Snorkel AI, but it's not a thing that we will double our uses because we have a good base. It's not like we're starting with the first model; then we'll produce ten models next year.”
Snorkel AI’s platform approach requires investing in onboarding and training users. Landing new contracts requires an expansive go-to-market motion. Snorkel actively hires Solution Engineers that to support production implementations in Snorkel Flow.
Specialized AI-enabled products may gain traction before Snorkel AI can establish a foothold. For example, AI-enabled Customer Service has multiple players who can fine-tune AI for each customer, such as Cresta and Forethought. Some of the top use cases for AI are relatively stable, potentially giving other horizontal players a stronger right to win. Enterprises may find their need for internally developed and managed solutions less painful as specialized products gain traction.
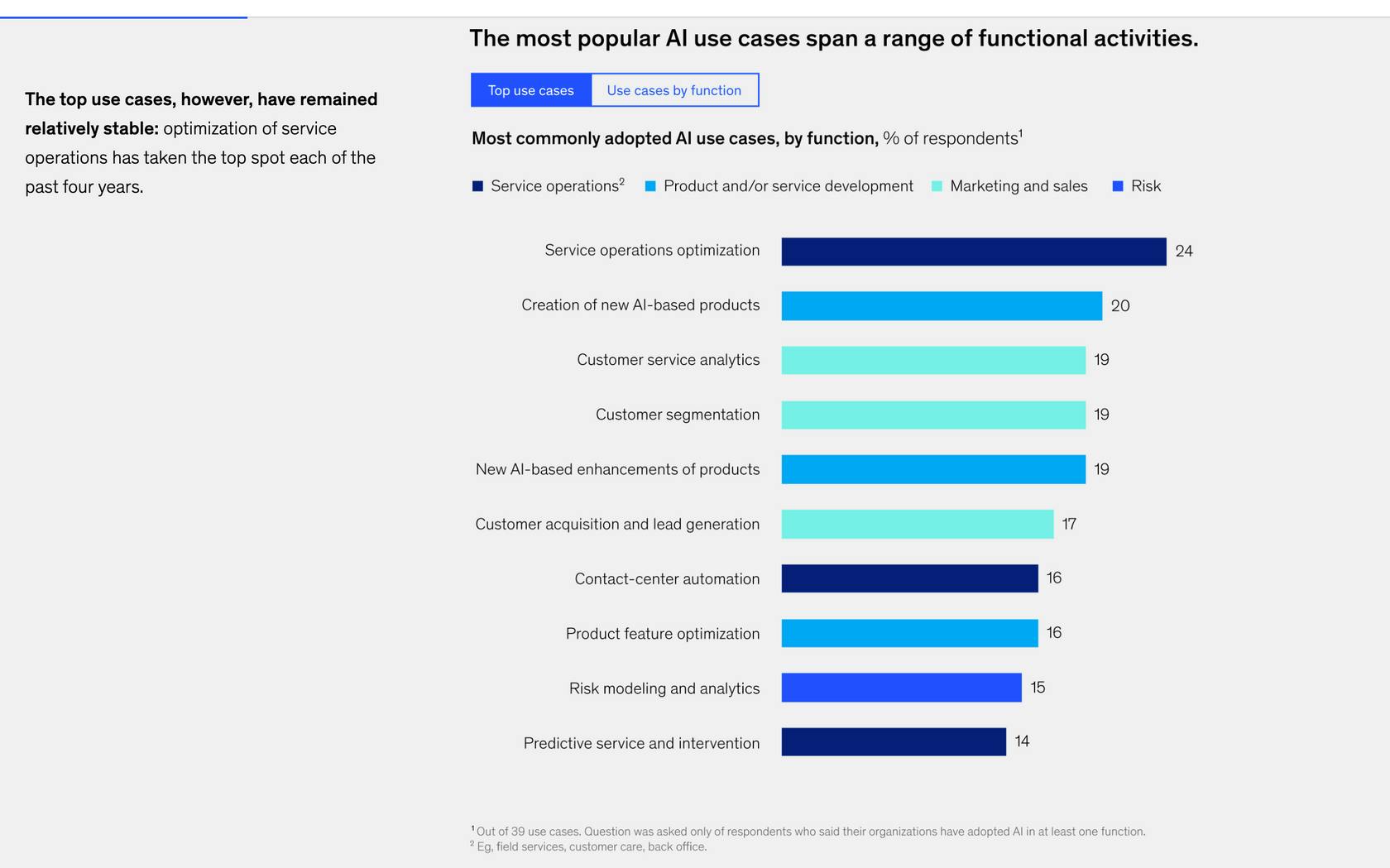
Source: McKinsey
Increased Competition
The core market for data labeling has new entrants, such as Kili in Europe and Heartex which aim to provide an open-source horizontal data labeling platform. While new horizontal players don’t have the latest and greatest weak supervision models for labeling data, down-market solutions may be good enough.
In certain use cases and verticals, such as image labeling, incumbents like Scale AI are strong players. One Snorkel AI customer noted that Snorkel AI was strong at text but at the “prototyping stages” for images. New entrants such as Encord focusing on medical images could also gain traction.
Summary
As AI becomes more advanced, enterprises are looking for customized, fine-tuned AI models for their specific use cases. However, most enterprise AI projects fail due to a critical bottleneck: assembling training data. Snorkel AI’s programmatic labeling approach tackles the toughest data labeling challenges, such as when data is private, requires domain expertise, changes frequently, or faces shifting product requirements. While data labeling is an increasingly contested space, Snorkel AI’s focus on the flexibility and speed of programmatic labeling enables it to stand out. As an end-to-end platform and workflow, Snorkel AI enables enterprises to unlock organizational knowledge and iterate on AI applications at software speed. However, its platform approach makes GTM and expansion harder, as Snorkel AI has to find and support fragmented internal use cases.