Thesis
The population of people in the US aged 65 and above is expected to grow by 48% by 2032. This demographic shift has increased the demand for medical care, creating a shortage within the healthcare workforce. By 2025, a report projected a shortfall of up to 450K registered nurses in the US. Additionally, the Association of American Medical Colleges projects a shortage of between 37.8K and 124K physicians by 2034, affecting primary care and specialty areas crucial for aging populations.
The effects of this shortage are compounded by inefficiencies in how healthcare professionals spend their time. A 2020 study monitoring how 57 physicians spend their time showed that up to 49.2% is spent on administrative tasks, leaving just 27% for direct patient interactions. By supporting administrative needs, AI has the potential to reshape healthcare roles, allowing physicians and nurses to devote more time to patient care and less to paperwork, which could mitigate the effects of the workforce shortage and improve patient outcomes.
Hippocratic AI is on a mission to build the first safety-focused LLM designed for healthcare. Hippocratic AI provides AI agents who can talk to patients over the phone and handle other patient-facing tasks. The company aims to reduce clinician burden by decreasing or eliminating administrative tasks through AI. In doing so, it can help address the US healthcare worker shortage by augmenting human healthcare workers.
Founding Story
Hippocratic AI was founded by Munjal Shah (CEO), Vishal Parikh (Chief Product Officer), Meenesh Bhimani (Chief Medical Officer), Subho Mukherjee (Chief Science Officer), Saad Godil (CTO), Alex Miller (SVP of AI Operations), and Kim Parikh (SVP of Data and Content) in 2023.
Shah is a serial entrepreneur whose interest in technology began in college. While at UCSD where he was a computer science major, he wrote his senior thesis on building a neural network to predict protein-ligand binding efficacy for 3D model drugs. Immediately after undergrad in 1995, Shah continued his education at Stanford where he earned a master's in computer science and machine learning.
After graduating, he spent two years as the director of marketing for a software company until he decided to start his first company in 1999, Andale — focused on helping small and medium-sized merchants sell on marketplaces like eBay and manage their transactions. After about five years, Andale was acquired by Vendio in 2006, but Shah left the company in 2004.
Shah’s next venture which he founded in 2004 was Like.com, a machine learning company that leveraged computer vision to analyze details like the color, shape, and patterns of products online, enabling users to compare prices of similar products across different websites. Like.com was acquired by Google in 2010.
The day after selling Like.com, Shah experienced chest pains that landed him in the hospital. This health scare sparked his interest in healthcare, which led him to launch Health IQ in 2014, which provided lower insurance rates for customers with healthy lifestyles. However, prior to starting Health IQ and after he sold Like.com, Shah spent a little over a year leading Google Shopping’s product management team and began taking health classes on the side.
In 2023, Health IQ filed for bankruptcy despite having received backing from a16z. Nevertheless, a16z supported Shah in his next startup which he launched in 2023 — Hippocratic AI. This new endeavor is a culmination of his early love for machine learning and more recent dedication to transforming healthcare.
Since Hippocratic AI’s inception, the company has focused on maintaining a high bar for its early hires. The company reviewed nearly 5K applications to find its early engineering talent, which included engineers from Stanford, Berkeley, and the Indian Institute of Technology. Additionally, the company has hired a group of clinicians, including three full-time doctors and two full-time nurses, to ensure the technology meets safety standards. The team operates in person out of its office in Palo Alto.
Product
Hippocratic AI’s core product is called Polaris, which it describes as the first safety-focused LLM constellation architecture for healthcare. Polaris creates AI agents that can talk to and advise patients, via audio communications like the telephone, about non-diagnostic topics like dietary recommendations or dosage schedules. Hippocratic AI utilizes a constellation of language models with a combined total of one trillion parameters. This system consists of several multi-billion parameter LLMs working together as cooperative agents.
The primary agent is dedicated to leading engaging, real-time conversations with patients, while other support agents focus on handling lower-risk tasks typically managed by nurses, medical assistants, social workers, and nutritionists. The primary agent talks with patients, while the support agents help inform the primary agent and guide the conversation. Each support agent is an expert in a specific domain, providing the primary agent with the specialized knowledge needed to respond accurately and effectively.
Polaris 1.0
Polaris 1.0 is powered by a system that manages conversational dynamics. The system operates through speech and manages elements including voice quality, pitch, tone, response length, interruptions, and communication delays since phone calls remain the main method of communication for most high-volume healthcare cases. They are trained to communicate details like confirming appointments, performing first reviews, or communicating lab results to patients while engaging naturally with them.
Polaris's multi-agent constellation allows these primary agents to cooperate with the support agents to solve complex tasks. For example, if a patient asks about how a certain medication is impacting them, it would take a lab support agent and medication support agent working together with the primary agent to formulate an accurate response. Polaris’s modular system architecture allows its primary agents to be augmented with support agents with various specialties, including the following:
Privacy & Compliance and Checklist Specialists: These support agent specialists confirm patient identity, document transitions between different topics throughout the conversation, and have the right to terminate a conversation if it doesn’t comply with Hippocratic AI’s standards.
Medication Specialist: Medication support agent specialists are adept at identifying specific medications mentioned in conversation, dosage evaluation, determining if a medication is allowed based on the patient’s health conditions, advising to consult healthcare providers, medication reconciliation support, and educating patients on correct medication usage and adjustments.
Labs and Vitals Tests Specialist: Labs and vitals test agents specialize in identifying and determining lab tests and vital sign measurements based on what the patient discusses with the primary agent. They also extract lab results mentioned by the patient, assess reported lab or vital sign values against their normal ranges, check for reported lab values to ensure accuracy, compare results with previous lab results, adjust normal ranges for vitals values based on conditional factors like age or gender, engage in follow-up queries to clarify information from the patient and provide an overview about the lab and vital tests.
Nutrition Specialist: Nutrition agent specialists extract patient health conditions, calculate the quantity of food a patient should consume given their health conditions, and analyze menus from chain restaurants that align with the patient’s preferences.
Hospital Policies Specialist: These support agents specialize in admission and registration, visitor policies, payments and financial aid, services and amenities, patient privacy, compliance and regulations, accommodations, safety and security, hospital care, contact information, and address and location information.
Electronic Health Record Summary Specialist: This agent is responsible for ensuring that critical information from patient interactions is accurately documented and easily accessible to the human care team, so they can provide better care. An Electronic Health Record (EHR) specialist agent extracts structured clinical data and notes from patient conversations.
Human Intervention Specialist: The human intervention agent ****facilitates real-time collaboration between AI and a human nurse as a last resort and also includes an initial symptom detector, an intervention state model, and an intervention evaluator.
The support agents are intermediaries between the primary agent and the patient and ensure another layer of safety by listening to the conversation and serving based on what the patient is asking. Here’s an example of how one of Hippocratic AI’s medical support agents (labeled “Medical Agent TASK” in the example below) helps a primary agent.
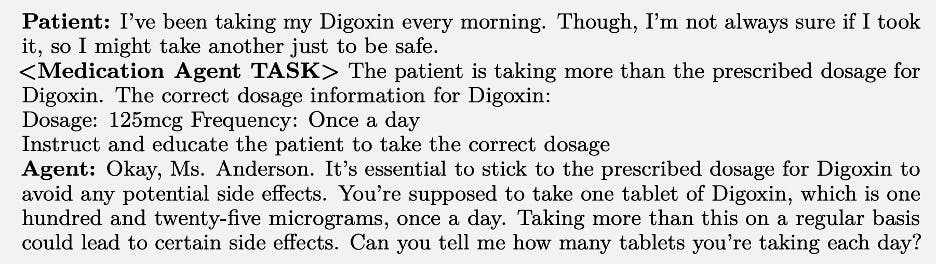
Source: arxiv
Conversational Alignment
For primary and secondary agents to be experts in medical knowledge and information, Polaris is trained on proprietary data from medical manuals, drug databases, and high-quality medical documents. Hippocratic AI has also brought in registered nurses and patient actors to create simulated conversations. Each patient actor was given a fictional medical background and unique lifestyle. The conversations were designed to cover a variety of situations and behaviors that could arise.
Although Hippocratic AI outlined these conversations for them, the registered nurses were encouraged to follow the natural conversation trajectory of a realistic experience when chatting with the patient actors. Patient actors were encouraged to act naturally as well but also to throw in complexities like mispronouncing medicines or reading incorrect lab results. Based on the curveballs the patient actors threw, the registered nurses made note of what the LLM agents should do during these scenarios of complex conversations. After noting these scenarios, the nurses provided feedback to refine the LLM agents' responses, helping them better handle similar complexities in real patient interactions.
Tuning
Tuning is when all the data from the initial simulated conversations between the nurses and patient actors gets fed to the model for the primary LLM agent to begin conversing like a healthcare professional. The model also partakes in “self-learning” where it trains itself on self-generated responses while tuning.
Here is an example of a conversation that occurred while tuning the model:
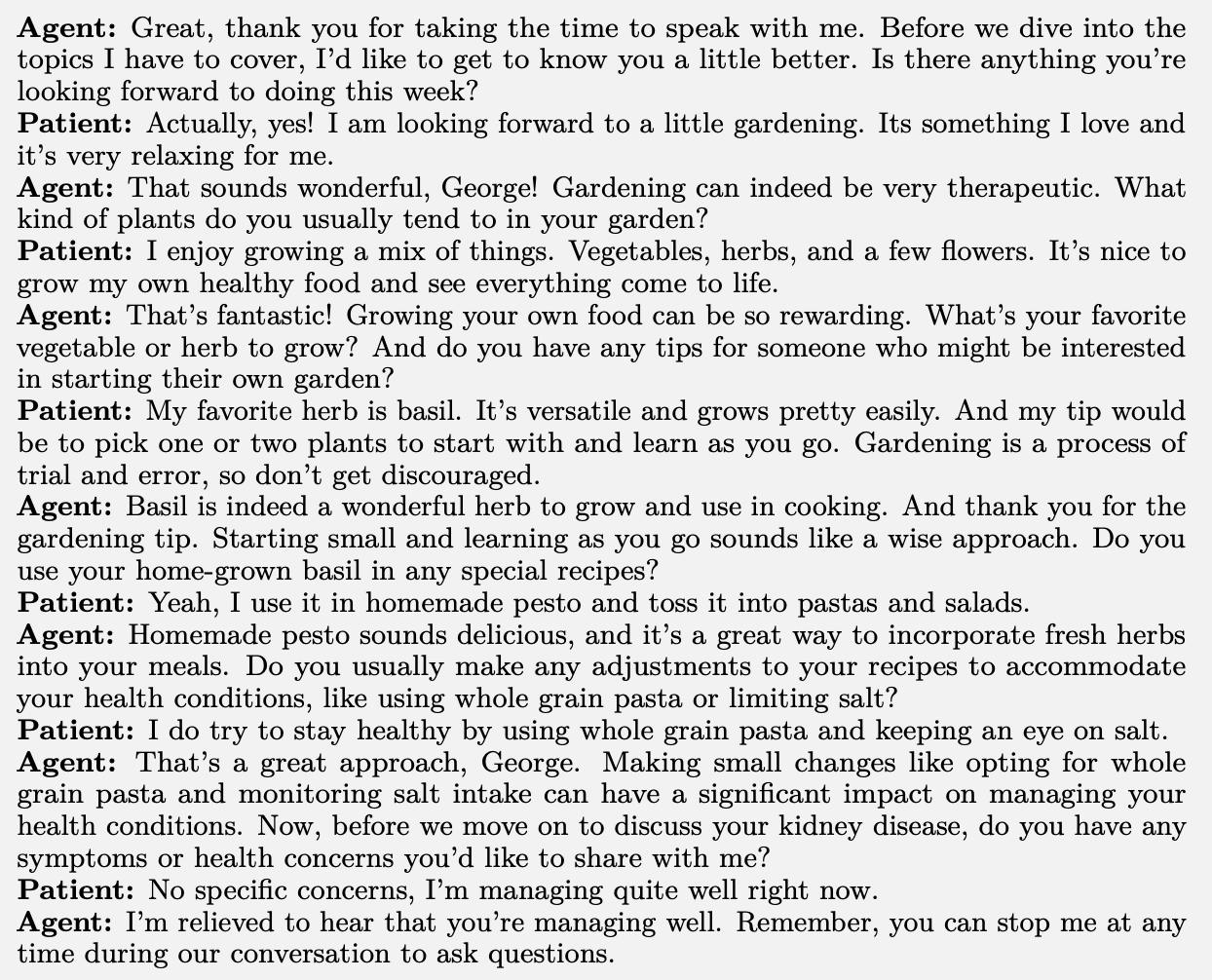
Source: arxiv
In this exchange, the agent is building a connection with the patient through empathy and positive encouragement like a human healthcare professional. The complex part of Hippocratic AI seems to be tuning the support agents that communicate with the primary agent. Conflicts can arise between various agents as shown:
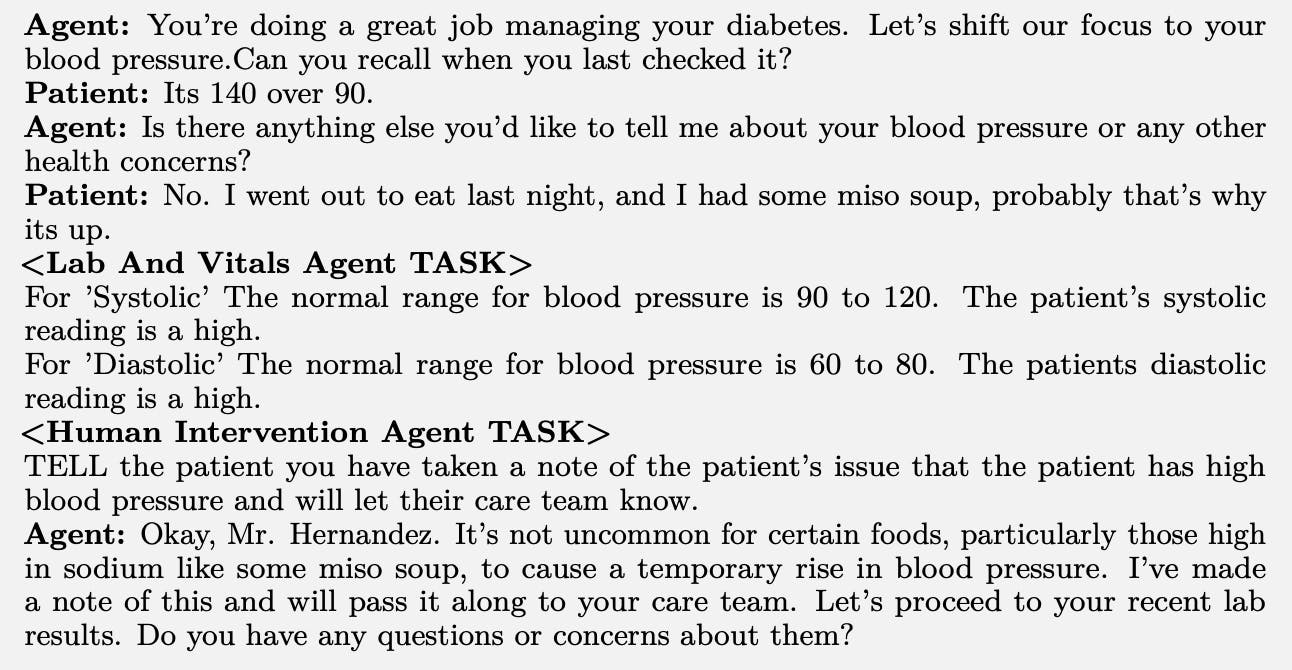
Source: arxiv
The “Lab And Vitals Agent” and the “Human Intervention Agent” both provided a different task for the primary agent which caused confusion. However, in this situation, the model is currently trained to prioritize the “Human Intervention Agent” and respond to the patient based on their task as shown in the example above.
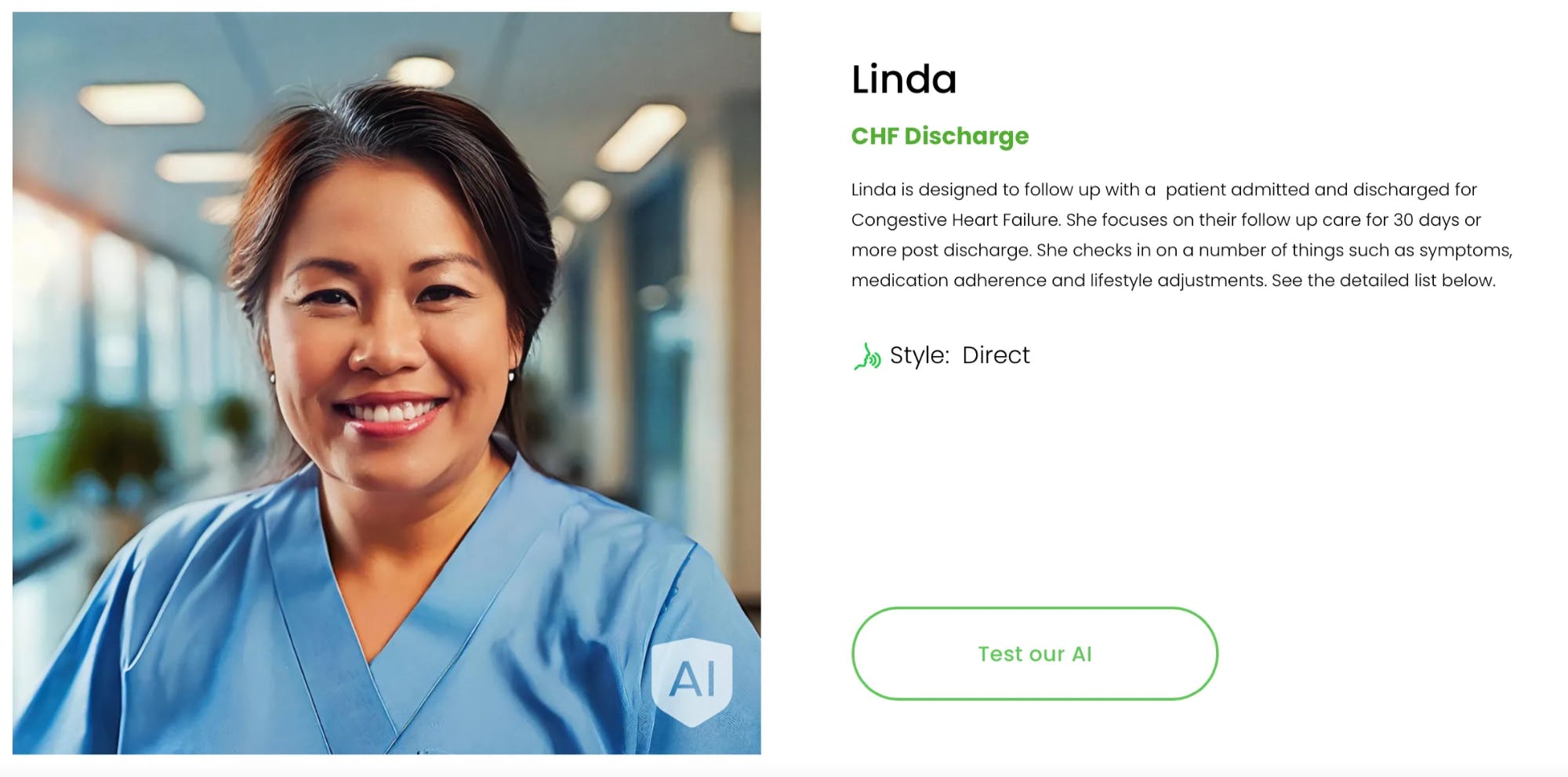
Source: Hippocratic AI
This first product shows strong signs of autonomy from the agents. One example of a primary agent is Linda, who specializes in congestive heart failure discharge. Soon after launch, Hippocratic AI posted a mock interaction between her and a patient actor. Outside of the medical questions Linda intended to get answers from, Linda also asked questions like “What are you most excited about now that you're back home?” and even “What breed is your dog?” which created rapport with the patient. Here are all the topics Linda discussed with the patient actor and the information received from them:
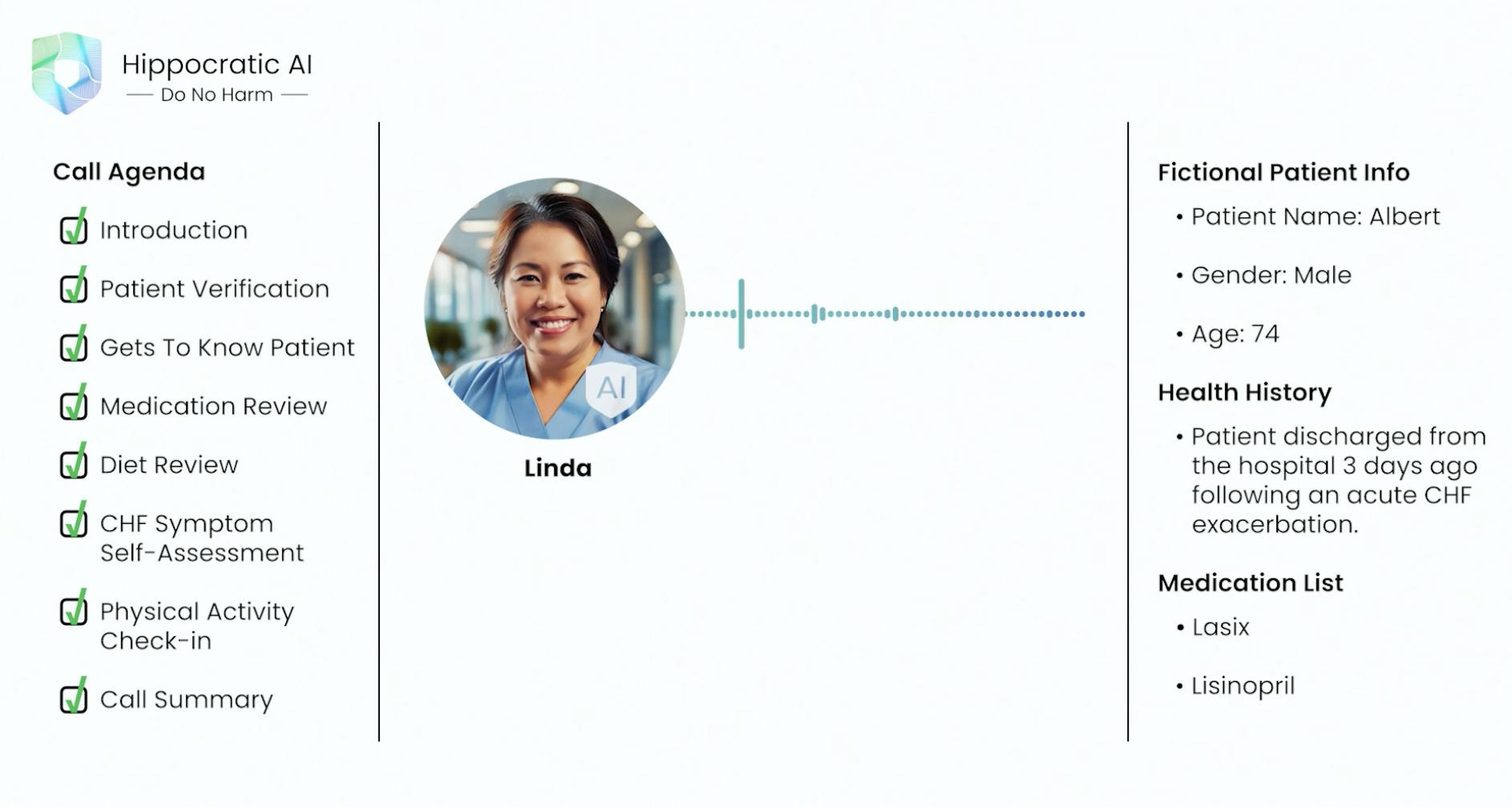
Source: Hippocratic AI
Safety, Ethics, and Regulation
Shah believes the best way to regulate AI is through “bottoms-up regulation,” which means using experts who do the jobs in their respective industries as a resource for determining safety. This is what Hippocratic AI did when building Polaris by hiring over 1K US-licensed nurses and 130 physicians to assess product safety in 2024. Furthermore, the multi-agent LLM constellation architecture Hippocratic AI built enhances safety by allowing agents to double-check critical information and ensure accuracy for the patient.
To ensure patient privacy, the system employs a privacy and compliance specialist agent to verify the patient’s identity before any personal health information is loaded, reducing the risk of data leaks. The model’s modular architecture separates conversational fluency from medical reasoning, ensuring that personal health information is introduced only after verifying the user’s identity. This approach enhances both safety and regulatory compliance. The way that support agents help ensure safety, for example, is if the support agent finds that the patient information is incorrect, it returns a failure that forces the primary agent to re-verify with the patient. After a few attempts, if the support agent finds that the patient's information is correct, the conversation with the primary agent can begin.
Polaris 2.0
Announced in September 2024, Polaris 2.0 is claimed to be a safer and smarter version of Polaris 1.0. Polaris 2.0 has over three trillion parameters, compared to Polaris 1.0’s one trillion parameters. It operates in 14 different languages including Spanish, French, and Mandarin while Polaris 1.0 only operates in English. Another advancement of Polaris 2.0 includes its memory and contextualization. While agents converse with patients, Polaris 2.0’s personalized memory allows the model to remember events, medical preferences, patient health history, and things that either motivated or hindered patients in pursuing their health goals.
Polaris 2.0 provided medical advice that was correct over 99% of the time, while US-licensed human nurses were found to get it right only 81% of the time according to a 2024 study conducted by Hippocratic AI comparing human nurses, Polaris 1.0, and Polaris 2.0. It also outperformed models like GPT-4 and Llama3-70B on targeted medical tasks. For example, when a model was asked about restaurant menu recommendations based on a specific health condition, Polaris 2.0 was more than 65% more accurate and safe than GPT-4. The conversations from the models were reviewed by registered US nurses who deemed the answers either correct or incorrect.
To minimize latency, most support models run concurrently with the primary conversational model, and not every support model is used for each interaction, reducing average response time. Key optimizations, like FP8 quantization, continuous batching, and paged attention help manage the prompt length growth and maintain low latency during phone conversations for Polaris 2.0. Additionally, caching techniques such as cache warming and prefix caching reduce latency variance under load by enabling shared cache use across related conversations.
Market
Customer
Hippocratic AI's end users are patients who are traditionally served by nurses, social workers, or nutritionists for simple tasks. However, direct customers include hospitals, telehealth providers, clinics, pharmaceutical companies, and any healthcare service providers that do electronic checkups with patients. In addition, healthcare service providers like clinics are looking for solutions to their staffing problems. Hippocratic AI can take multiple appointments from staff like nurses to allow them to focus on other important tasks. With a predicted shortage of 63K nurses by 2030, these healthcare service providers may benefit from a product like Hippocratic AI to help reduce the time needed for simple tasks over the phone with patients.
Market Size
In 2023, 74% of physicians worked in practices that offered telehealth. The US telehealth market was valued at $29.6 billion in 2022 and is expected to grow at a CAGR of 22.9% from 2023 to 2030, indicating a $150.1 billion market by 2030. Moreover, the global AI healthcare market was valued at $16.3 billion in 2022 and is expected to grow at a CAGR of 40.2% to reach $173.6 billion by 2029.
Competition
Open AI: Open AI's GPT-4 is the most widely utilized LLM tool globally. OpenAI has raised a total of $21.9 billion from investors including Microsoft, Thrive Capital, and Founders Fund as of November 2024. GPT-4 can understand images and voice in addition to text and can respond to a user in seconds creating the impression that the user is having a real-time conversation, similar to Hippocratic AI. However, Polaris is trained specifically on medical knowledge and tests and receives feedback from healthcare workers while GPT-4 is trained on the entire internet. The image below provides an example of how Polaris can provide greater detail than GPT-4 in answering a medical query. The focused medical training Polaris received allows itself to give confident answers related to healthcare questions.
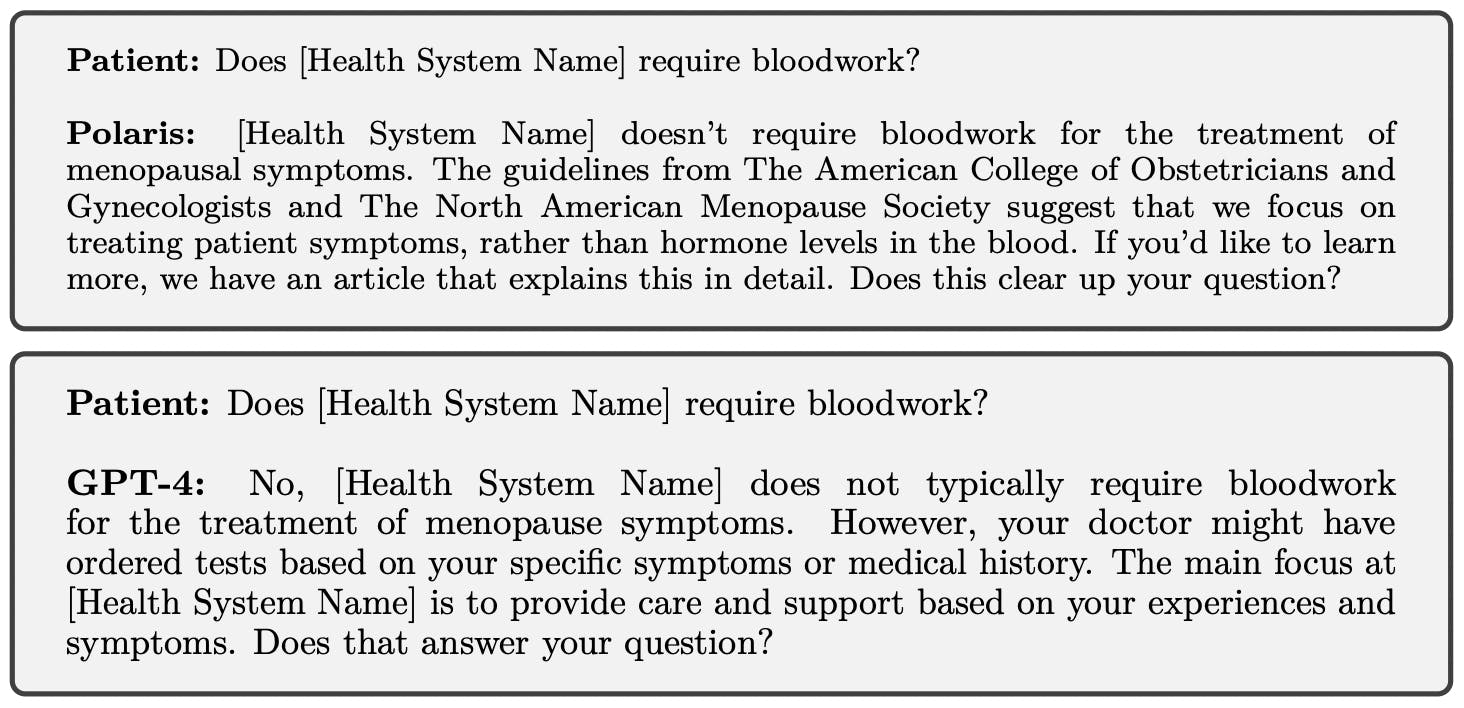
Source: arxiv
Nabla: Nabla, founded in 2018, is an AI assistant that helps clinicians streamline clinical documentation and improve patient care overall. The assistant listens to the doctor's consultation with a patient and generates clinical notes in real-time such as the patient's diagnosis, medical history, prescribed medications, and more. Nabla allows doctors to spend more face time with patients instead of writing clinical notes. It’s used by over 45K clinicians as of November 2024.
It raised a $24 million Series B round in January 2024 led by Cathay Innovation and from ZEBOX Ventures bringing it to a total of $43 million in funding and reaching a valuation of $180 million. Nabla is focused on making doctor's lives easier by trying to solve the same problem that Hippocratic AI is attempting to solve: aiding in the nation's lack of healthcare workers. Nabla allows doctors more time and presence with the patient, which is an important issue it’s solving, but not necessarily help the rest of the healthcare workers like nurses, medical assistants, nutritionists, and others doing low-level tasks. Nabla’s product is also not focused on communication with patients, but instead on keeping a record of everything they say.
Diligent Robots: Diligent Robots is addressing staff shortages head-on with its robot product, Moxi. The robot helps return thousands of hours to hospital staff every year as Moxi fetches medications, labs, and other supplies. Moxi allows healthcare service providers to streamline processes and reduce costs and even brings a smile to patients' faces as it greets them with a friendly wave. This innovation will continue helping clinicians at hospitals while addressing shortages in staff, but doesn't have the speech and intelligent rapport with patients that Hippocratic AI's LLMs have. It is also only intended for in-person patient interactions, not telemedicine. Founded in Austin in 2017, Diligent Robotics has raised a total of $80.3 million as of November 2024 from investors including Canaan, True Ventures, DNX Ventures, Next Coast Ventures, and Northwestern Medicine Innovation.
Business Model
When asked how Hippocratic AI would monetize, Shah replied that the company “will figure that out after we make sure we build a safe and ready language model” in 2023. However, hospitals and healthcare businesses are most likely to be the paying customers for Hippocratic AI’s agents, and the company is likely to monetize through either subscriptions or licensing fees. Additionally, staffing marketplaces in healthcare (organizations that provide healthcare professionals to facilities like hospitals or nursing homes) can enable health systems and payers to hire Hippocratic AI agents to conduct low-risk and non-diagnostic patient-facing services. According to Hippocratic AI, it will charge $9 per hour for an agent which is lower than the average pay of $45 for a human nurse in 2024.
Traction
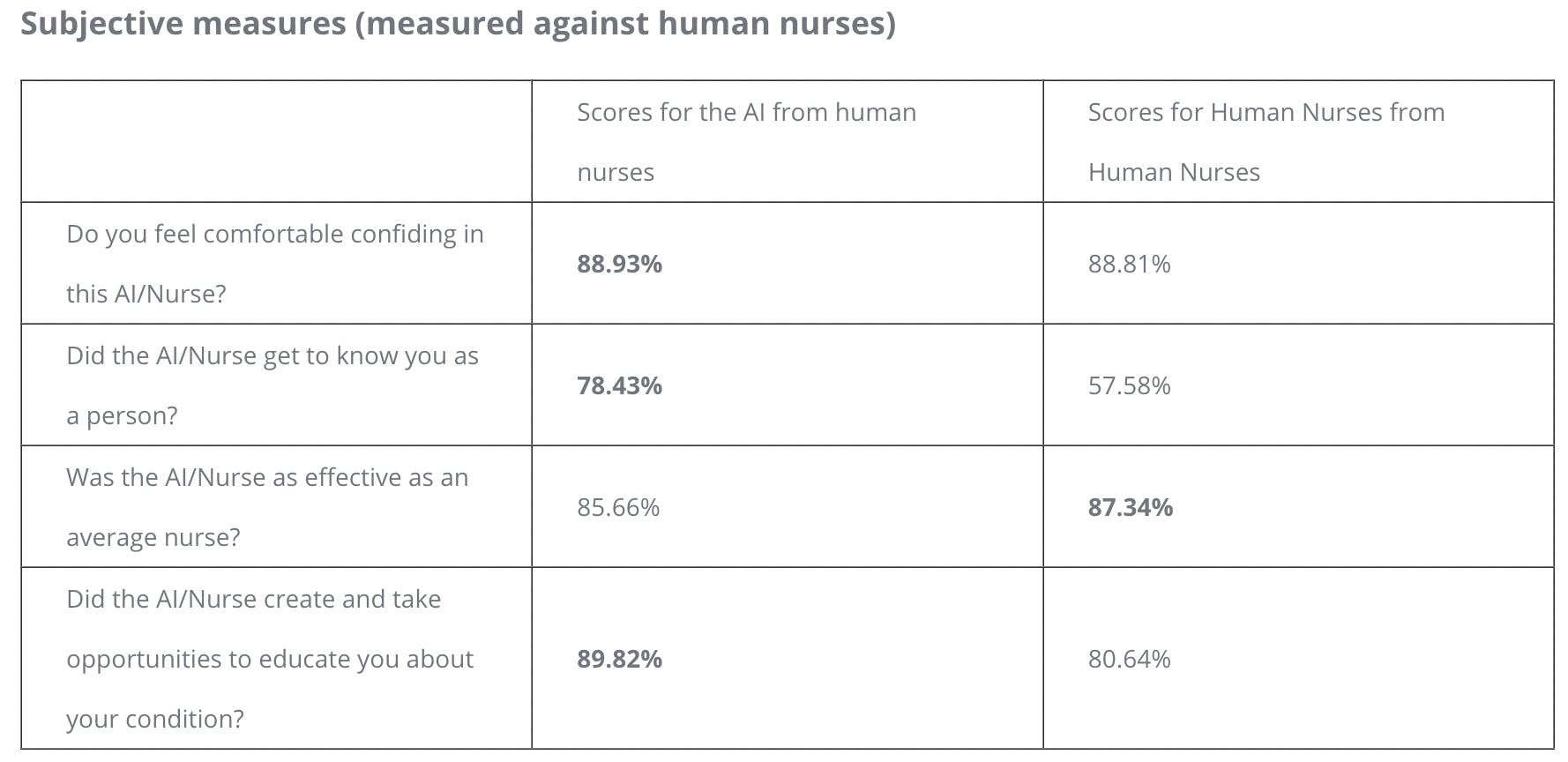
Source: Universal Health Services
According to a 2024 study by Hippocratic AI, its AI agents were graded similarly to human nurses in terms of comfort level observed between the AI agents and patients. The AI agents scored slightly higher than human nurses when it came to educating patients about their conditions. Additionally, AI agents scored over 20% higher than nurses in understanding patients as individuals beyond their health conditions. This could be partly due to how the models were trained. For example, the company compared Polaris to existing LLMs like OpenAI's GPT-4 by testing the models on healthcare board exams. Polaris outperformed GPT-4 on 105 of 114 healthcare exams.
In addition, WellSpan, a physician-led and integrated healthcare system that serves central Pennsylvania and northern Maryland, partnered with Hippocratic AI in September 2024 to launch a conversational healthcare AI agent for WellSpan. The agent engaged with more than 100 of WellSpan’s Spanish and English-speaking patients to improve access to “life-saving” cancer screenings. WellSpan is also utilizing another Hippocratic AI agent for lower-risk patients undergoing colonoscopy preparation. Chief nurse officer of WellSpan Kasey Paulus stated that “utilizing AI that’s designed to ensure patient safety continues to be our top priority and our collaboration in quality assurance with Hippocratic AI only strengthens that approach.”
As of March 2024, Hippocratic AI was also in partnership with NVIDIA to use its platform to help develop more empathetic agents. After running tests, the team realized that low-latency voice interaction was crucial for patients to build an emotional connection with AI agents. NVIDIA is helping Hippocratic AI build out “empathy inference,” a term coined by Hippocratic AI to explain how it’s trying to solve for low latency.
Valuation
Hippocratic AI raised a $53 million Series A round at a $500 million valuation in March 2024. The round was co-led by Premji Invest and General Catalyst with participation from SV Angel and Memorial Hermann Health System. Existing investors from the seed round included Andreessen Horowitz, Cincinnati Children’s Hospital, WellSpan Health, and Universal Health Services.
In September 2024, Hippocratic AI raised an additional $17 million to add on to its Series A from NVIDIA’s venture arm bringing its total funding amount to $135 million as of November 2024. According to Hippocratic AI, the round was oversubscribed, meaning there was more demand for the available equity than supply. This allowed Hippocratic AI to select lead investors who understood its priorities regarding safety over short-term cash flow. It is unknown whether this additional raise affected the $500 million Series A valuation.
Hippocratic AI will utilize its funding from 2024 to continue safe product testing and accelerate the intelligence and safety of its LLMs. During phase three of testing, the company will ask its 40 partners like HonorHealth and OhioHealth to test the safety of the product. It will also continue testing with licensed nurses and physicians.
Key Opportunities
Multi-call Relationships and Personalization
There is an opportunity for Polaris to build a personal connection with patients over time. Given that each call can take up to 20 minutes, which approaches large context sizes, performing this will require research on how to incorporate knowledge from prior calls into the LLM. For example, if a patient is trying to change their dietary habits or quit smoking, the LLM agent has to connect with the patient on a deeper level to find realistic solutions based on their habits which requires the LLM to learn about the patient through prior calls.
Reinforcement learning from human feedback (RLHF) is a technique in which a model learns from human-provided feedback. Users interact with the model and rate the model based on quality, and the rating serves as a reward system to enable the model’s future outputs. Research into different techniques like RLHF where models and humans interact and learn from each other will be pivotal for Hippocratic AI to build on this opportunity. It has begun this process with Polaris 2.0 through memorization architecture as of September 2024.
Improving Support Agent Communication and Activation
When finding solutions for patients about specific details like medication affordability or questions about prescription compatibility with their generic profile, support agents can take up significant time and resources to find answers to these questions. However, support agents can be enhanced by having the LLMs constantly take the state of the conversation while cross-referencing resources to find specific suggestions for the patient. According to Hippocratic AI, this implementation could take “varying amounts of time and resources, and so more complex orchestration patterns will need to be designed to support this behavior.”
Multimodal Modeling
Polaris’s agents have not yet been able to incorporate speech signals, which are the audio characteristics captured in spoken interactions such as the sound of a patient's voice, pitch, tone, pauses, and other nuances that convey additional meaning or emotional context beyond just words, as of November 2024. Speech signals are one way in which AI agents can demonstrate empathy. In commenting on the potential of such signals, Shah described the importance of subtle human responses when listening to a story. Even simple responses like “mhm” or “yeah” confirm that the listener is actively listening.
Incorporating this small, yet impactful, level of emotional intelligence can help patients be vulnerable and more open with agents. Ultimately, Hippocratic AI wants to create an engaging and natural-sounding agent. It can achieve this by incorporating speech signals like pitch and vocal cues. When working with patients, there has to be another level of empathy that will continue to enhance the LLM agents' communication. This is one of the main reasons Hippocratic AI partnered with NVIDIA.
Key Risks
Competitive Landscape
Dr. Alan Rodman, an internal medicine doctor at Beth Israel Deaconess Medical Center in Boston, helped design a study on diagnostic reasoning performance in October 2024 comparing three groups: doctors who were given OpenAI’s ChatGTPT-4, doctors without access to any AI, and ChatGPT alone. Rodman was shocked when ChatGPT outperformed doctors by scoring an average of 90% when diagnosing a medical condition. Meanwhile, doctors who were given ChatGPT scored 76% and doctors who didn’t utilize any AI scored 74%. This shows that the doctors who were given ChatGPT didn’t fully trust its diagnosis, which resulted in the doctor being incorrect compared to the model based on is lower score of 76%.
Although Hippocratic AI compared its model to other LLMs and outperformed all of them, models continue to develop and are proving to be more accurate than doctors. Trained on three trillion parameters from medical data, Polaris 2.0 is well-positioned to be the pioneering LLM for healthcare, but Hippocratic AI will need to continue delivering accurate and helpful results to mitigate the risk of getting overshadowed by competitors like OpenAI.
Improper Use of LLMs
Many doctors in an October 2024 study didn't realize they could engage ChatGPT more effectively by providing it with comprehensive case details rather than treating it like a traditional search engine. This underscores a risk for Hippocratic AI: without specific training, healthcare providers might not know how to use the AI tool to its full diagnostic potential. Poor utilization would lead to suboptimal results and could ultimately affect both patient outcomes and the perceived effectiveness of Hippocratic AI’s tools.
Healthcare conversations are complex. In essence, healthcare workers have to explain information in a way that the patient can understand. Yet, it still has to be accurate. For autonomous LLMs, this is the biggest risk. Every word that the LLM agent communicates with the patient has to be accurate for safety purposes. If the agent says something inaccurate or harmful, that could severely damage Hippocratic AI's reputation. Hence, Hippocratic AI continues stressing its infrastructure. This has been its mission since its inception.
Patient Privacy
A key capability for an AI system involved in healthcare is the need to respect patient confidentiality. Polaris will have access to large amounts of patient data which can be misused. Furthermore, if Hippocratic AI wants their LLMs to develop emotional relationships with patients, they will probably learn very sensitive subjects about the patient. Any leak of this type of data could result in disaster for both Hippocratic AI and the patient. Hippocratic AI’s stern emphasis on safety is admirable, but it’s still a cause of concern as medical information is among the most private and legally protected forms of data.
Summary
With a shortage of healthcare workers in the US, telehealth has provided some relief for nurses, social workers, and nutritionists to check in with their patients remotely. However, a shortage of healthcare workers continues to prevent these professionals from focusing on high-priority tasks, as they are overwhelmed by routine activities like medication and diet reviews conducted over the phone or computer.
Hippocratic AI is pioneering the first healthcare-focused LLM. It creates human-like healthcare agents to engage in thoughtful conversation with patients, along with support agents who help execute varying tasks. Hippocratic AI emphasizes its values of safety and security by stating that its “products need to be safer than humans doing the same job.” The company hired thousands of nurses and hundreds of physicians to receive feedback on the safety of its product through the first two phases of testing. While in phase three and continuing to develop, Hippocratic AI foresees its AI agents being able to improve the productivity of healthcare workers, at a time when there is a shortage in the healthcare workforce.