Thesis
Platform Shifts
Ever since the rise of the internet in the late 90s, there has been a major technology platform introduced in every subsequent decade. Each has given birth to a new category of companies. The rise of personal computers gave birth to Microsoft, the cloud led to Amazon Web Services, mobile drove Apple’s rebirth, and Facebook was born on the rising tide of social media. In 2022, technological breakthroughs taking place in artificial intelligence (AI) may be giving rise to the next major platform shift that will define the decade to come.
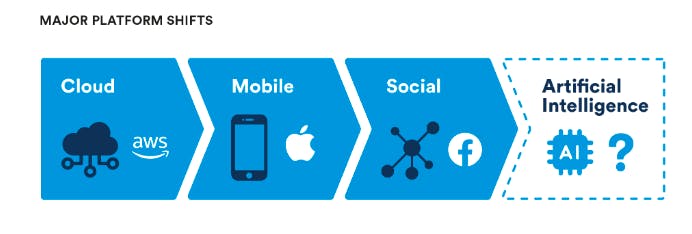
Source: Base10
Major breakthrough technologies like transformers and large language models (LLMs) beg the question of whether we’re on the precipice of a similarly monumental shift in technology. While unlocking Artificial General intelligence (AGI) would be almost certainly be a quantum leap eclipsing any previous technology trend, even the more limited recent breakthroughs in AI and LLMs could prove quite significant. At the least, it seems likely they will unlock at least as much value as the migration to the cloud did in the last decade.
More than any other company, OpenAI has been at the forefront of the recent AI breakthroughs. OpenAI provides foundational models that could serve as infrastructure powering a new generation of consumer and enterprise AI applications, just as Amazon’s AWS capitalized the shift to the cloud. AWS built significant moats by becoming an infrastructure layer, accruing first-mover advantages, and having access to capital to manage the high upfront costs of building an ecosystem that has given them a formidable lead within the cloud market.
Similarly, OpenAI has developed competitive moats that position them to capitalize on the large market opportunity presented by recent breakthroughs in AI. It has built the core infrastructure that powers a huge part of the entire generative AI ecosystem; an ecosystem that has given rise to over 353 companies (so far) in 2022. In addition, OpenAI’s partnership with Microsoft has provided access to capital, and data that has created a high barrier to entry for competitors. Finally, the company has accrued brand and ecosystem advantages by becoming one of the first movers to release its technology publicly.
Unlocking The Market Opportunity in AI
The market tailwinds for the AI industry are large, with some estimates projecting that the AI market could grow to $422 billion by 2028, representing a 39% CAGR, driven by the use of natural language processing (NLP) models to solve problems within different industry verticals. IDC expects the aggregate global data to grow from 33 zettabytes in 2018 to 175 zettabytes (or 175 trillion gigabytes) in 2025. Most of these datasets haven't been analyzed to generate insights because there is not enough capacity to effectively mine the zettabytes of data being created daily on the internet. More than 65% of companies reported revenue increases attributable to AI adoption, and productivity gains are expected to meaningfully increase due to generative AI.
OpenAI’s recent launch of ChatGPT has driven rapid consumer adoption due to its ability to answer questions with a high level of specificity, context, and personalized descriptions. Five days after launch, ChatGPT surpassed over 1 million users, growing even faster than previous blockbuster platforms like Instagram or Spotify. ChatGPT’s success has prompted conversations around the possibility of OpenAI disrupting Google’s $200 billion search business. As OpenAI adds more features over the coming years, and adoption grows rapidly, the ability to steal some of Google’s 250 million unique visitors per month doesn’t seem out of the realm of possibility.
OpenAI’s models have the ability to continually improve as they are fed more training data. These advanced foundational models have significantly higher processing power than competing models in the market. With future technologies like GPT-4 on the horizon, OpenAI could become even more dominant. What was once a non-profit could end up becoming one of the most important companies of the last 20 years, at minimum. DARPA, which gave birth to some of the most fundamental technology of the last 100 years, like satellites, GPS, the internet, and more, was once hailed as an organization “that shaped the modern world.” Could a research organization with the talent density of OpenAI achieve the same level of impact?
Founding Story
An Idea Developing
Sam Altman has told the story of founding OpenAI a number of times. He’s remarked that he’d always been fascinated by AI, even from his early days at Stanford. After dropping out of school, he went on to start a YC-backed location-based social network that failed. In 2012, he became a partner at YC. That same year happened to be a pretty interesting year for artificial intelligence, with breakthroughs in speech / image recognition, and neural networks.
In 2014, it was announced that Sam Altman would take over for Paul Graham as President of YC. During his time leading YC he rapidly expanded their programs, launching a growth fund, a research institution, and pushing YC to invest in more hard tech like neural networks and nuclear energy. Early in 2015, Altman felt not enough attention was being paid to artificial intelligence as a whole. Any work being done was commercial, but very little progress was made on foundational levels of the technology.
All-In
In December 2015, Altman left his role at YC and announced the formation of OpenAI as a non-profit research organization with himself and Elon Musk as co-chairs. They articulated their vision this way:
“Our goal is to advance digital intelligence in the way that is most likely to benefit humanity as a whole. We believe AI should be an extension of individual human wills and, in the spirit of liberty, as broadly and evenly distributed as possible.”
The list of people involved in the organizations was a who’s-who in the world of AI.
“OpenAI’s research director is Ilya Sutskever, one of the world's experts in machine learning. Our CTO is Greg Brockman, formerly the CTO of Stripe. The group’s other founding members are world-class research engineers and scientists: Trevor Blackwell, Vicki Cheung, Andrej Karpathy, Durk Kingma, John Schulman, Pamela Vagata, and Wojciech Zaremba. Pieter Abbeel, Yoshua Bengio, Alan Kay, Sergey Levine, and Vishal Sikka are advisors to the group.”
A combination of some of the leaders involved, some of the leaders of YC like Paul Graham, and corporate sponsors like AWS and Infosys together donated $1 billion to the organization. Although the technology was nascent, the vision was clear. It was established with the mission of ensuring artificial general intelligence (AGI) benefits humanity. The early vision was that OpenAI would openly collaborate with other institutions and researchers by making their patents and research open to the world.
Over time, OpenAI recognized some of the limitations of their existing model and changed its status from non-profit to "capped" for-profit in March 2019, with a 100x profit limitation across all investments. They explained their reasoning this way:
“We’ll need to invest billions of dollars in upcoming years into large-scale cloud compute, attracting and retaining talented people, and building AI supercomputers. We want to increase our ability to raise capital while still serving our mission, and no pre-existing legal structure we know of strikes the right balance. Our solution is to create OpenAI LP as a hybrid of a for-profit and nonprofit—which we are calling a “capped-profit” company.”
Talent Density
For the company to be able to accomplish its bold vision, it needed to attract talent at the forefront of such a nascent technology. Their early challenge was trying to attract world-class talent as a non-profit when companies like Google or Amazon had a significant war chest to dramatically overpay the best talent. Microsoft Research vice president Peter Lee made the battle for talent clear:
“The cost of a top AI researcher had eclipsed the cost of a top quarterback prospect in the NFL.”
While larger companies were offering researchers 2-3x their market rate for compensation, the team at OpenAI was taking a very different approach. They offered “the chance to explore research aimed solely at the future instead of products and quarterly earnings.” They were able to attract prominent individuals like Ilya Sutskever (a top researcher at Google in neural networks), Yoshua Bengio (founding father of deep learning) and Wojciech Zaremba (Polish mathematician focused on neural networks from Nvidia, Google Brain, and Facebook). Other key participants in OpenAI’s talent ecosystem include Pieter Abbeel, an AI and Robotics professor at UC Berkeley who led AI research at UC Berkeley and Andrej Karpathy, who was a senior director of AI at Tesla, where he led the team responsible for all neural networks on the Autopilot. His Ph.D. from Stanford in Deep Learning, Computer Vision, and Natural Language Processing was integral to the early research efforts on OpenAI.
In February 2018, Musk left the OpenAI non-profit board due to a conflict of interest with Tesla’s AI goals and disagreements about the organization’s direction, though he continues to donate to and advise the organization. Today, the company is run by Sam Altman who became OpenAI’s CEO in 2019 and as of May 2022, Greg Brockman is President, Brad Lightcap is COO, and Mira Murati is CTO. OpenAI employs over 450 people across its three main divisions:
Capabilities: Advancing what AI systems can do
Safety: Ensuring those systems are aligned with human values
Policy: Ensuring appropriate governance for the systems.
Products
History of OpenAI’s Projects
After launching in 2016, the company kept experimenting with different projects and applications. Some of their products included applying neural networks toward robotics or developing games that could beat humans. Most of these were early experiments and research into the fundamentals of Artificial Intelligence. However, a major breakthrough came about two years after launching.
In June 2018, a paper was published where researchers at OpenAI had unlocked some key developments in language models titled “Improving Language Understanding with Unsupervised Learning.” This was the first time that researchers demonstrated the possibility of improving language models by combining transformer models and unsupervised pre-training. This gave birth to what most people now know as GPT (Generative Pre-Trained Transformers).
Foundational Models & APIs
OpenAI is primarily focused on advancing research and building foundational models in reinforcement learning, natural language processing, robotics, generative language, and multimodal models. At the core of OpenAI’s product is providing an extensible API (Application Programming Interface) that provides access to all of their models. Although OpenAI has a variety of research and project initiatives within its pipeline, it’s core products (or research models) can broadly be categorized into: (1) GPT (generative pre-trained transformers) and (2) DALL-E (large models for images).
GPT
GPT, at its core, is a neural network machine learning model trained using data on the internet to generate any type of text (similar to the synaptic function of a brain). GPTs are unsupervised language models that require a relatively small amount of input text to generate large volumes of specific machine-generated text. The first iteration of GPT was GPT-2 which was released in 2019. At the time, it had over 1.5 billion parameters and achieved a high level of accuracy after only being trained on 8 million documents across the web and Reddit.
GPT-3
GPT-3 was initially released to the research community in September 2020, before it was more broadly released to the public through an API in March 2021. What made GPT-3 such a meaningful release was the fact that GPT-3’s language was trained on over 175 billion parameters, 10x the previous models of 1.5 billion and its performance had zero-shot and few-shot learning compared to 7 out of 8 tested results for GPT-2’s model. The progress between GPT-2 and GPT-3 occurred in less than nine months, demonstrating the rapid pace of innovation within the industry.
GPT-3 enabled people to generate or analyze language content without writing any code, and spend less time and resources retraining a model. Users only needed to show the model one or two examples of the output they expect, and it starts to perform the task on new input with meaningful accuracy. For example, a college student used GPT-3 to write fake blog posts that went viral, and most people did not realize that the blog was AI-generated. Developers use GPT-3 for various applications such as email composition, text summary, website design, and software code generation. For example, GPT-3’s mode called ‘summarize for a 2nd grader’ translates legal terms of startup term sheets, or the EULA terms for the video game Far Cry, into plain English that is easy to read. The full paper behind GPT-3 can be found here.
As of March 2021, GPT-3 was powering over 300 applications, generating over 4.5 billion words per day, and ten-of thousands of developers around the globe were building on the platform.
ChatGPT
ChatGPT (Chat Generative Pre-Trained Transformer) is a chatbot built on top of OpenAI’s GPT-3 model. Notably, its key difference is that ChatGPT is fine-tuned from a model in the GPT-3.5 series. This new generation of models was trained on a blend of text and code (articles, books, Reddit comments, human conversations etc.) before Q4 2021.
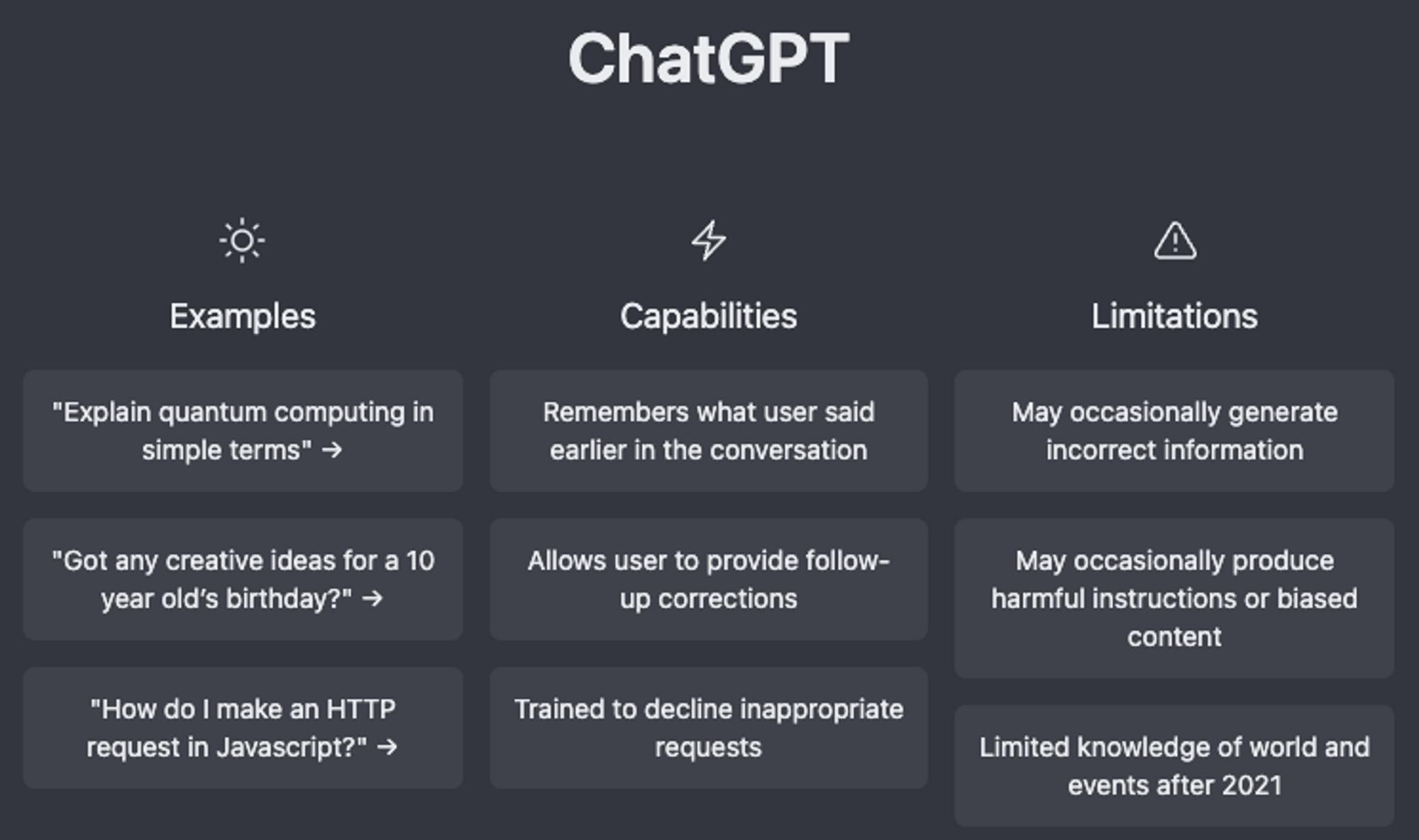
Source: OpenAI
OpenAI launched a research preview in November 2022. The model was trained using reinforcement learning from human feedback (RLHF). Essentially, reinforcement learning in AI means training a machine learning model to become better at making decisions. It involves feeding the model with input data and prompting it to give an output. If it gets the output wrong, a human keeps making adjustments until it gets the right answers. OpenAI trained the model using supervised fine-tuning. Human AI trainers provided conversations in which they played both sides—the user and an AI assistant. The trainers were given machine model-written suggestions to help them compose their responses. This entire process was repeated multiple times.

Source: OpenAI
ChatGPT has seen significant growth in usage, reaching over 1 million users in five days, often having to limit visits to the site given the volume of demand. Some estimates put the cost to operate ChatGPT at $1 - 3 million per day. Despite its success, the product still has some drawbacks and critics. One of its key drawbacks is that it writes in such a confident way, but in many cases, its answers are inaccurate or factually wrong. The model makes guesses or assumptions about a user’s intent behind their questions. The nature of prompts or questions asked of the model are sensitive. Users have to be able to craft questions in such a way for the model to provide a good response. Ultimately, OpenAI has been clear that this is a more conservative model designed to get users’ feedback and learn about its strength and weaknesses.
OpenAI Codex
OpenAI Codex emerged from the GPT-3 model. OpenAI’s Codex is a neural net model trained to write and generate code. OpenAI Codex was trained on 54 million GitHub repositories and 159 GB of Python files. Codex is the product that powers GitHub’s Copilot, offering auto-completion for software developers. OpenAI claims the largest Codex model it developed, which has 12 billion parameters, can solve 28.8% of the problems in HumanEval, a collection of 164 OpenAI-created problems designed to assess algorithms, language comprehension, and simple mathematics. OpenAI claims that Codex is even more advanced than GPT-3:
“It has a memory of 14KB for Python code, compared to GPT-3 which has only 4KB—so it can take into account over 3x as much contextual information while performing any task. GPT-3’s main skill is generating natural language in response to a natural language prompt, meaning the only way it affects the world is through the mind of the reader. OpenAI Codex has much of the natural language understanding of GPT-3, but it produces working code—meaning you can issue commands in English to any piece of software with an API. OpenAI Codex empowers computers to better understand people’s intent, which can empower everyone to do more with computers. Once a programmer knows what to build, the act of writing code can be thought of as (1) breaking a problem down into simpler problems, and (2) mapping those simple problems to existing code (libraries, APIs, or functions) that already exist. The latter activity is probably the least fun part of programming (and the highest barrier to entry), and it’s where OpenAI Codex excels most.”
Codex can translate natural language to code and is used to render web pages, launch web servers, and send emails. Real world examples of what can be done using Codex include creating Mailchimp interface capabilities with three lines of instructions, or creating a user interface in JavaScript. It can also generate data science code and charts using Python’s matplotlib library.
Codex has some meaningful implications for the future of software development. We covered some of these in our report on the “Evolution of DevOps,” where we discussed the implications of Copilot in significantly optimize developer productivity. Companies like Replit are also creating similar products like Ghostwriter, a product that was explored in a recent Contrary Research event.
DALL-E
DALL-E is a transformer-based model that creates images. It was first launched in January 2021. DALL-E uses a 12 billion parameter version of GPT-3 to interpret natural language inputs. DALL-E is a network trained on 400 million pairs of images and text. DALL-E was announced in conjunction with CLIP (Contrastive Language-Image Pre-Training). CLIP is an open source diffusion model conditioned on CLIP image embeddings. These models efficiently learn visual concepts from natural language supervision. OpenAI built CLIP to help assist in ranking DALL-E’s output when the latter model was trained. The core focus for developers and researchers has been optimized for DALL-E.
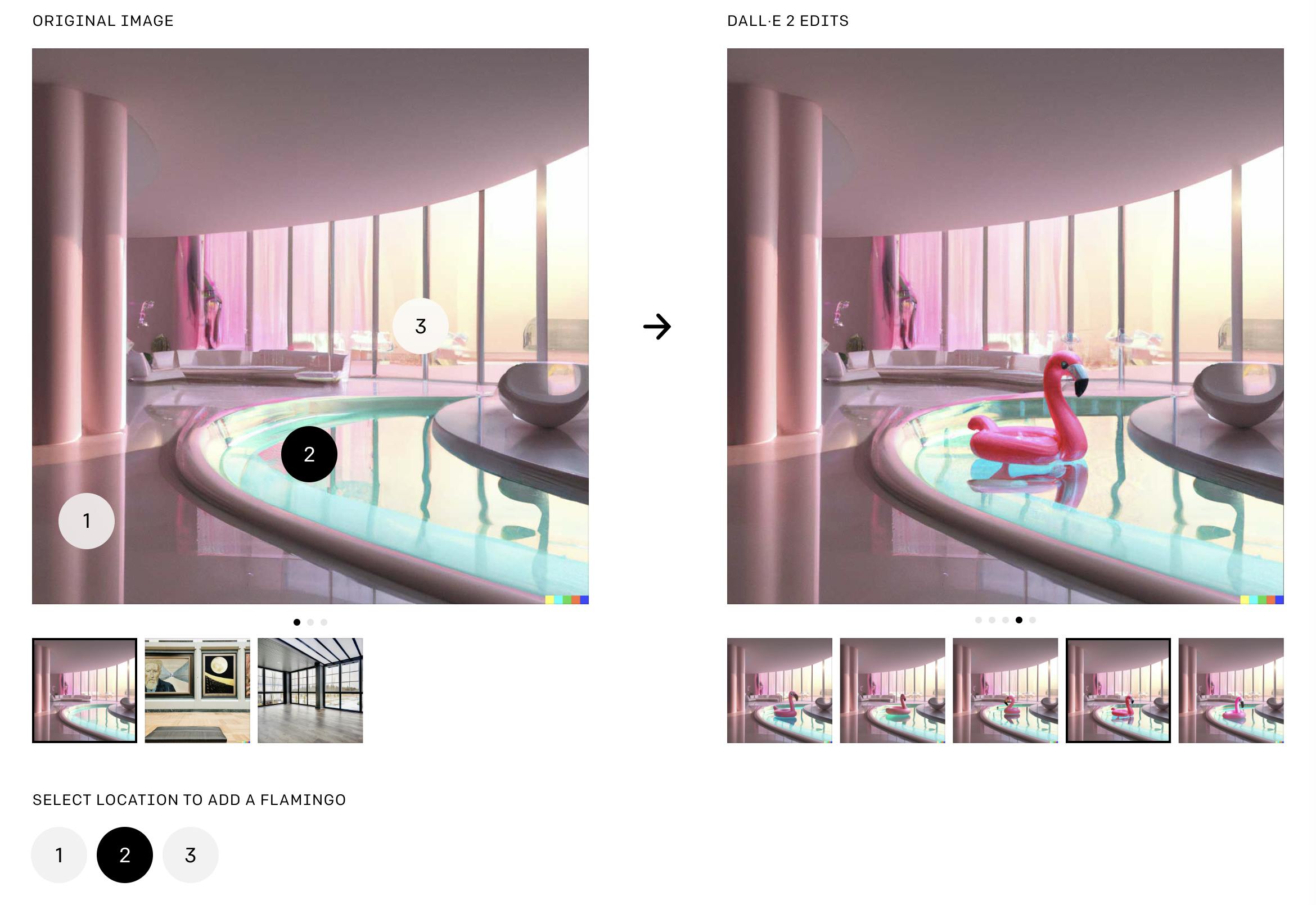
Source: OpenAI
In April 2022, OpenAI announced DALL-E 2, a new AI system for creating realistic images and art from descriptive English prompts or words. Use cases for DALL-E 2 include combining or editing existing images and creating different variations of existing images. In the future, more AI models trained by OpenAI will be provided through the API, such as DALL-E 2.
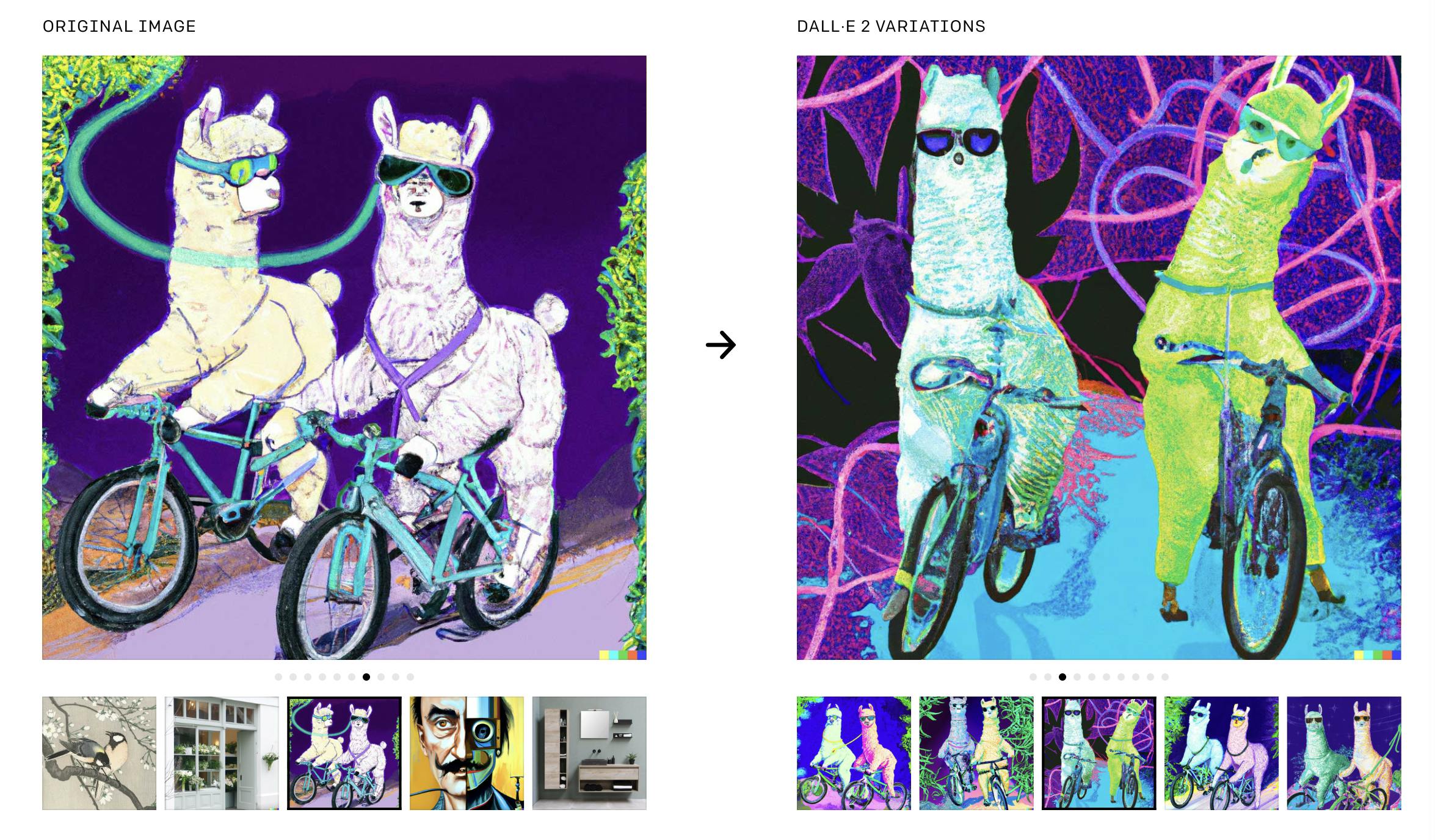
Source: OpenAI
OpenAI Portfolio of Other Projects
There are other products, models, and applications offered by OpenAI that are worthy of highlighting. Many of these are not core focus areas at the moment, but over time may evolve into similarly impactful products:
OpenAI Whisper: OpenAI open-sourced Whisper, which is an English-language speech recognition model. Whisper can be used to build applications that support the transcription of multiple languages into English or vice-versa.
OpenAI's MuseNet: This is a deep neural net trained on GPT-2 to predict subsequent musical notes in music files. It can generate musical compositions with 10 different instruments.
OpenAI Gym: This is a python-based programming environment created for developing and testing learning agents. These are suited to help people advance reinforcement learning techniques.
OpenAI Debate game: OpenAI’s goal of trying to develop explainable AI that can learn to audit AI decisions and detect lies.
OpenAI RoboSumo: Robotics designed to simulate human actions like tackling or kicking and being adaptable to changing conditions.
OpenAI Dactyl: A human-like robotic hand that is capable of manipulating physical objects. Its most popular application was when a Dactyl robot was able to solve a Rubrik’s cube in 60% of the time it takes the average person.
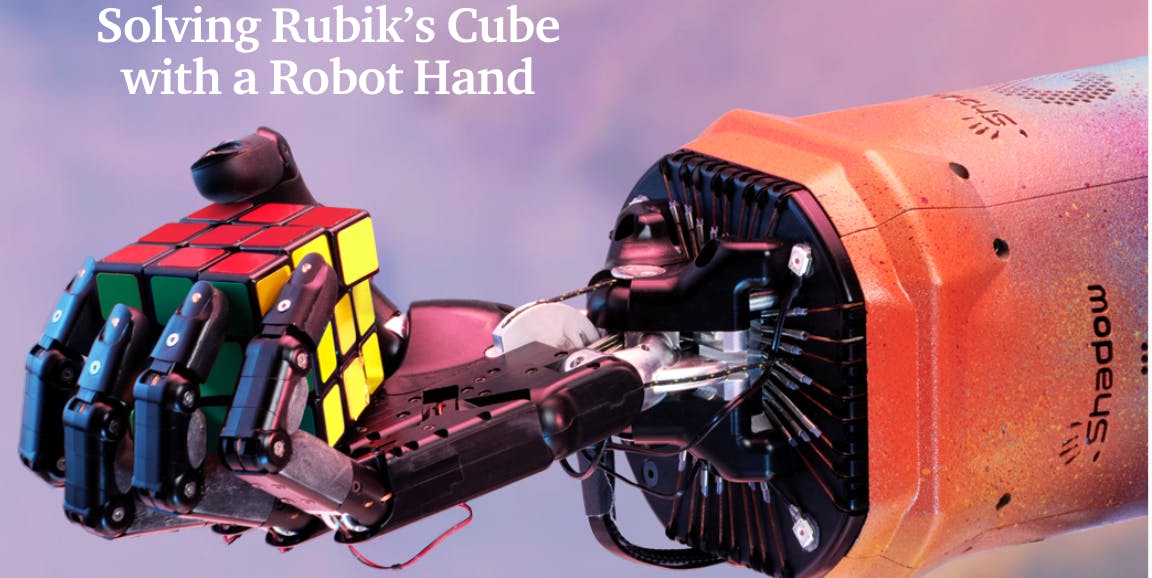
Source: OpenAI
Market
Customer
There is wide applicability and access to everything that OpenAI has built. OpenAI’s product can be used across consumers, startups, and enterprises. The majority of breakthroughs and applications in AI haven’t been made public given the sensitivity of the underlying data and models. OpenAI has been explicit about making its research and models publicly available through user interfaces. The sophisticated models behind DALL-E and ChatGPT are easily accessible and useable. One way of framing this evolution comes from Justin Gage at Amplify, in his article titled “ChatGPT, and why are these new models so good”
“AI has gone from a by-researchers-for-researchers (and practitioners) discipline to a by-researchers-for-the-public-discipline.”
OpenAI is typically offering API-driven products, meaning it mainly target developers or researchers at smaller companies and startups who either don’t have the resources to train a large AI model, or don’t have established AI capabilities. These developers would otherwise take off-the-shelf open-source AI models from Github. Training ML models is hard and expensive if people don’t have data or computing power. The biggest success story of a customer building on top of OpenAI is Jasper, a text generator for marketing, copywriting and sales built on a model from GPT-3. There are several other generative AI companies that rely upon OpenAI’s transformer models.
Beyond the core developer or startup profile, it’s been increasingly clear that the average non-technical user will be a key consumer of OpenAI’s products. Educators, researchers, non-profit organizations, healthcare institutions, and many others will rely on OpenAI’s models either for basic day-to-day tasks or for models for specific industry verticals.
Market Size
The interwoven aspects of the technology in the AI market can make it difficult to determine the size of the opportunity. One framework for doing so is looking at the demand for the core technology itself, and demand to embed the product in consumer and enterprise applications.
Core Technology
The market for the core NLP technology is projected to grow at a CAGR of 25.7%, from $11.6 billion in 2020 to $49.4 billion by 2027. This growth is primarily driven by the demand for technologies that can analyze text and generate insights from them. Sectors with massive sets of data, like healthcare, can use these models to analyze patient data across millions of individuals.
Governments, healthcare, and other large sectors that affect the lives of millions of people can leverage tools from OpenAI to drive better outcomes. This category closely aligns with OpenAI’s mission.
OpenAI has also created interfaces with prompts that have made it easy for non-technical users to utilize them. The web app has large implications for the future of education that some prominent authors and educators, like Daniel Herman, have written about in pieces like “The End of High-School English.” Paul Krugman wrote an OpEd talking about the implications of ChatGPT coming for skilled jobs. All of this suggests that once OpenAI starts to monetize and releases the full version that is plugged into the internet more broadly, ChatGPT alone could easily spread to hundreds of millions of users globally.
Powering Generative AI Economy
Large language models, such as OpenAI’s GPT-3, are also expected to become platforms providing developers with the building blocks to create their own enterprise and consumer applications. Nvidia CEO, Jensen Huang, shares this sentiment:
“In the future, you’re going to see large language models essentially becoming a platform themselves that would be running 24/7, hosting a whole bunch of applications.”
OpenAI already powers thousands of commercial applications, including AI copywriting, semantic search, and customer support. There are currently over 353 companiesbuilding generative AI that have raised over $7.2 billion in funding during 2022. Viable uses GPT-3 to analyze customer feedback and generate summaries and insights on behalf of its clients. Duolingo uses it to provide French grammar corrections. Copywriting tool, Copy.ai, is also powered by GPT-3. As the demand for these models continues to grow, and the computational costs decrease over time, OpenAI could find itself powering an entire economy similar to how Amazon’s AWS controls a great part of applications in our economy today.
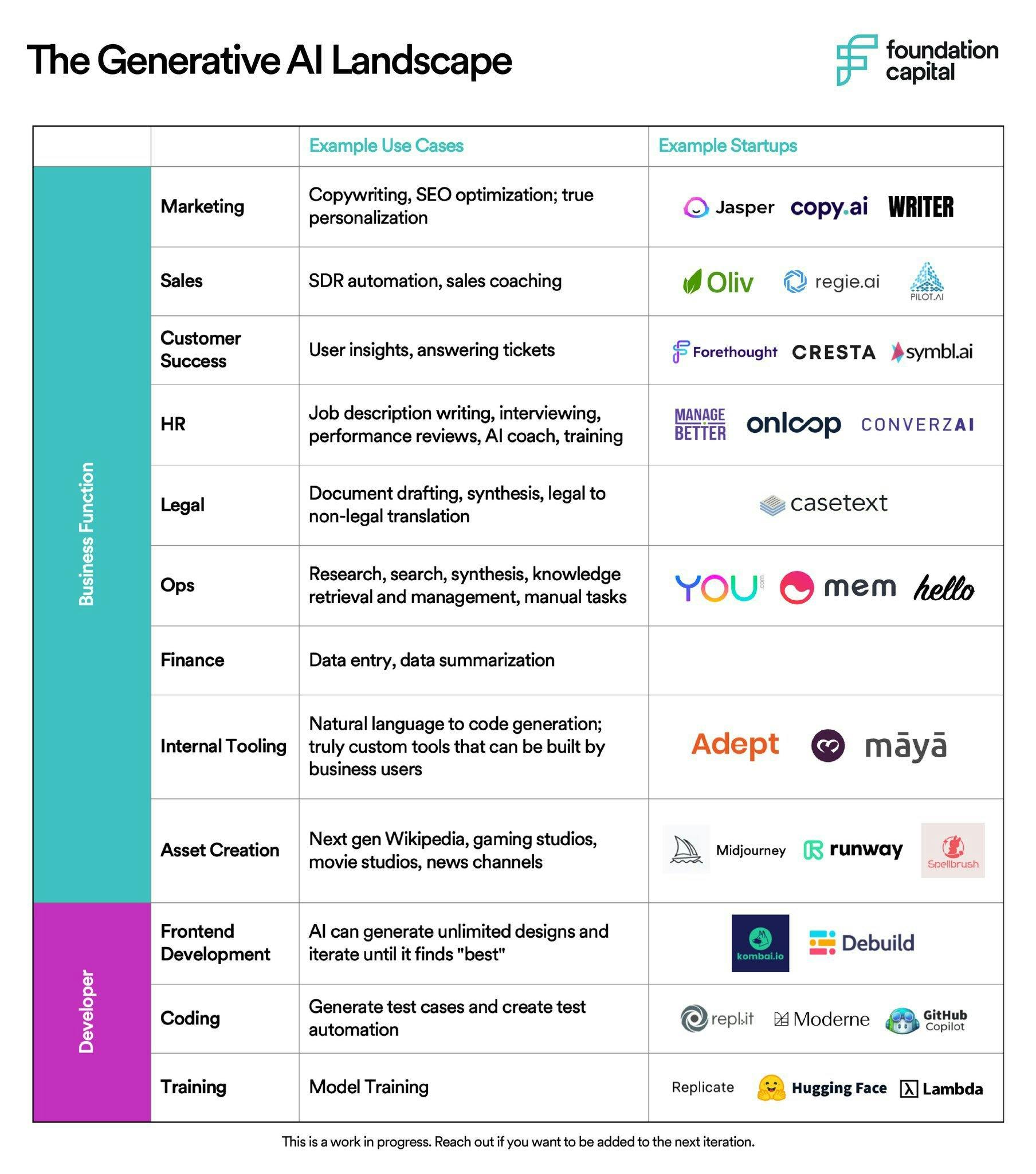
Source: Foundational Capital
Competition
AIphabet’s DeepMind
OpenAI most directly competes with other well-funded AI research labs, such as Alphabet’s DeepMind and Meta AI Research, all of which have released large scale language models similar to performance in GPT-3. However, OpenAI also competes with companies selling commercial NLP APIs, such as Google Cloud Natural Language AI and IBM Watson.
DeepMind is Google’s AI research laboratory based in London. It was acquired by Alphabet for $500 million in 2014. DeepMind has been one of the leading companies at the forefront of building neural networks, which are lagging behind many of the LLMs today. DeepMind famously created a neural network that learned how to play video games in a fashion similar to that of humans. This happened when Google’s AlphaGo beat a former Go world champion. DeepMind publicly launched its single visual language model (VLM) named Flamingo which could accurately describe a picture of something with just a few training images.
Commercial Competition
Beyond the AI research organizations in large tech companies like Alphabet, OpenAI competes with other companies, such as Cohere, AI21 Labs, Hugging Face and Stability AI.
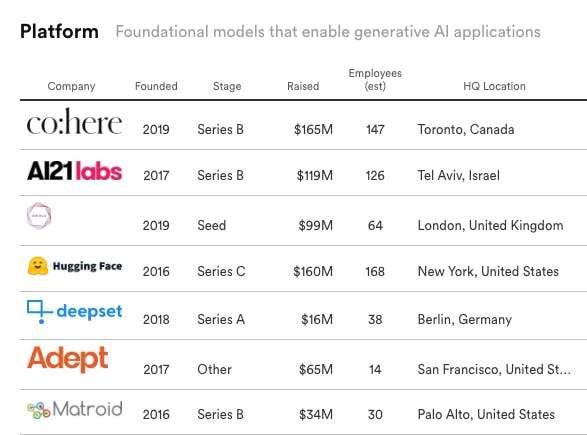
Source: Base10
Cohere & AI21
As of September 2022, Cohere and AI21 Labs offer more or less the same features and capabilities as OpenAI, with comparable model performance and accuracy. Cohere is available on the Google Cloud and AWS marketplaces. AI21 Labs is not integrated with cloud server providers. However, it built its own consumer-friendly products, including Wordtune, which helps edit people’s grammar as they type. For more information on Cohere, read the full Contrary Research memo.
Hugging Face
Companies like Hugging Face (the “Github of Machine Learning”) indirectly compete with OpenAI. Hugging Face is a platform for developers to build and collaborate on top of pre-trained models easily (many of which include NLP models similar to OpenAI’s GPT-3). Additionally, it provides hosted AI services and a community-driven portal for AI tools and data sets. Hugging Face already contains some alternative models to GPT-3 with similar performance and accuracy, and their models can be deployed on Microsoft Azure. For more information on Hugging Face, read the full Contrary Research memo.
Stable Diffusion & Stability AI
Stable diffusion is a large language model specifically built for text-to-images. It is used to generate detailed images based on a text description. Its core differentiation is that it can run on most consumer hardware equipped with a typical GPU. This marked a departure from previous proprietary text-to-image models such as DALL-E and Midjourney, which costs $10 per month for 200 images which are primarily accessible on the cloud.
Another core differentiator of stable diffusion and Stability AI have is open source, large language models. This means that to utilize them, users need to put in more effort relative to leveraging OpenAI, and it requires signing up on Hugging Face, which offers access to the Stable Diffusion code itself. OpenAI only makes its APIs available publicly, however, StabilityAI makes its APIs available, but publicly releases all the code as well.
The Evolution of The Business Model
Non-Profit Research Organization
OpenAI was originally founded with the focus of being a non-profit research organization that advanced Artificial General Intelligence (AGI) research for the benefit of humanity. In its non-profit structure, all funds either earned or donated must be used for pursuing the group’s objectives and paying its operating costs.
However, as costs skyrocketed to run the GPUs and compute necessary, OpenAI needed to find ways to continue funding its operations. As Sam Altman explains, the amount received by donors wasn't enough to manage the necessary infrastructure. The company also reached out to governmental bodies that didn't want to commit to the capital necessary to fund their initiatives. It was at this point they decided to create OpenAI LP and a new “capped-profit” company that made it possible for them to increase the amount of compute and access to talent. Altman elaborated on the capped profit model, and how it sets a cap on the profits that investors and shareholders can make from the company. It also gives a significant amount of power to the OpenAI non-profit board, which now governs the OpenAI LP organization.
Microsoft Partnership
In July 2019, Microsoft began a partnership with OpenAI. As a part of the partnership, OpenAI agreed to have its services run on Microsoft Azure, and in exchange, Microsoft became OpenAI’s preferred partner for commercializing its AI technologies. Microsoft built a custom supercomputer for OpenAI to train GPT-3. As a result of their partnership with Microsoft, OpenAI will their services to run on Microsoft Azure, and Microsoft will become OpenAI’s preferred partner for commercializing new AI technologies.
Traction
OpenAI mostly generates revenue by providing access to its API for a fee. The business and pricing are structured across its image models (DALL-E) and language models (GPT). Across those, customers can purchase more fine-tuned custom models. Additionally, there are embedded models which allow for building advanced search and topic modeling. OpenAI generates an average of 4.5 billion words per day. API calls are priced per 1,000 tokens, which is approximately equal to 750 words. Depending on the model and pricing tier used, the API generates between $4.8K - $360K in revenue per day or $1.7 - $131.4 million per year.
Across the business, it offers usage-based pricing in a pay-as-you-go model. Most of OpenAI’s revenue is generated based on those APIs used to power GPT-based applications. However, it remains unclear how they will monetize ChatGPT. Sam Altman has stated that they will have to charge at some point. There are some sources estimate that OpenAI recently presented a plan to potential investors indicating its expectation of $200 million in revenue in 2023, and $1 billion by 2024.
On the cost side, it's been well-documented that operating the models behind DALL-E and GPT can be incredibly expensive. GPT-3 took months to train and cost around $12 million. Additionally, OpenAI spent additional resources fine-tuning and improving its model. Other costs are associated with training large AI models like cloud computing costs, data storage, model inference, and having humans in the loop.
Sam Altman has publicly indicated that these models can cost single-digit cents per chat (which means somewhere around $0.02-$0.08). An estimate from Verge suggests that it probably costs close to $3 million a day to run ChatGPT. Given that DeepMind, another AI research lab, reported paying more than $483 million to its 700 employees in 2018, we can estimate that OpenAI probably pays out several hundred million dollars to its 400+ employees yearly. While most of these numbers are estimates, any costs will likely keep increasing as more users leverage the platform. A key question becomes, if OpenAI decides to charge based on usage-based pricing or subscription based on its cost structure.
In terms of user traction, OpenAI’s user base has primarily grown through ChatGPT.
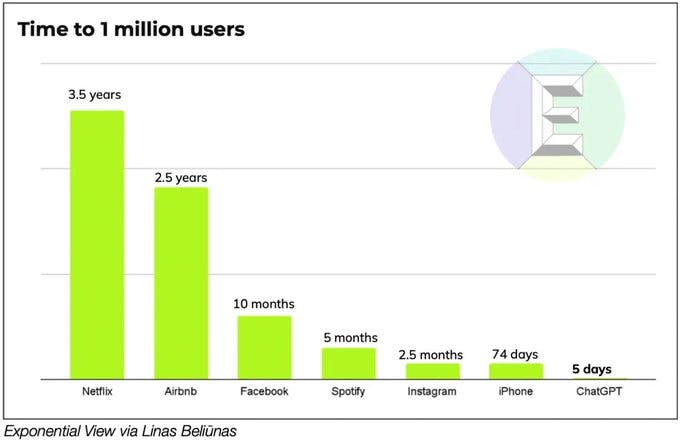
Source: Collaborative Fund
Platforms like Spotify have over 433 million users as of Q2 2022 or Instagram has over 2 billion active users a month. Many of these platforms grew with the help of advertisement or paid marketing. However, the adoption of ChatGPT has largely been driven by word of mouth.
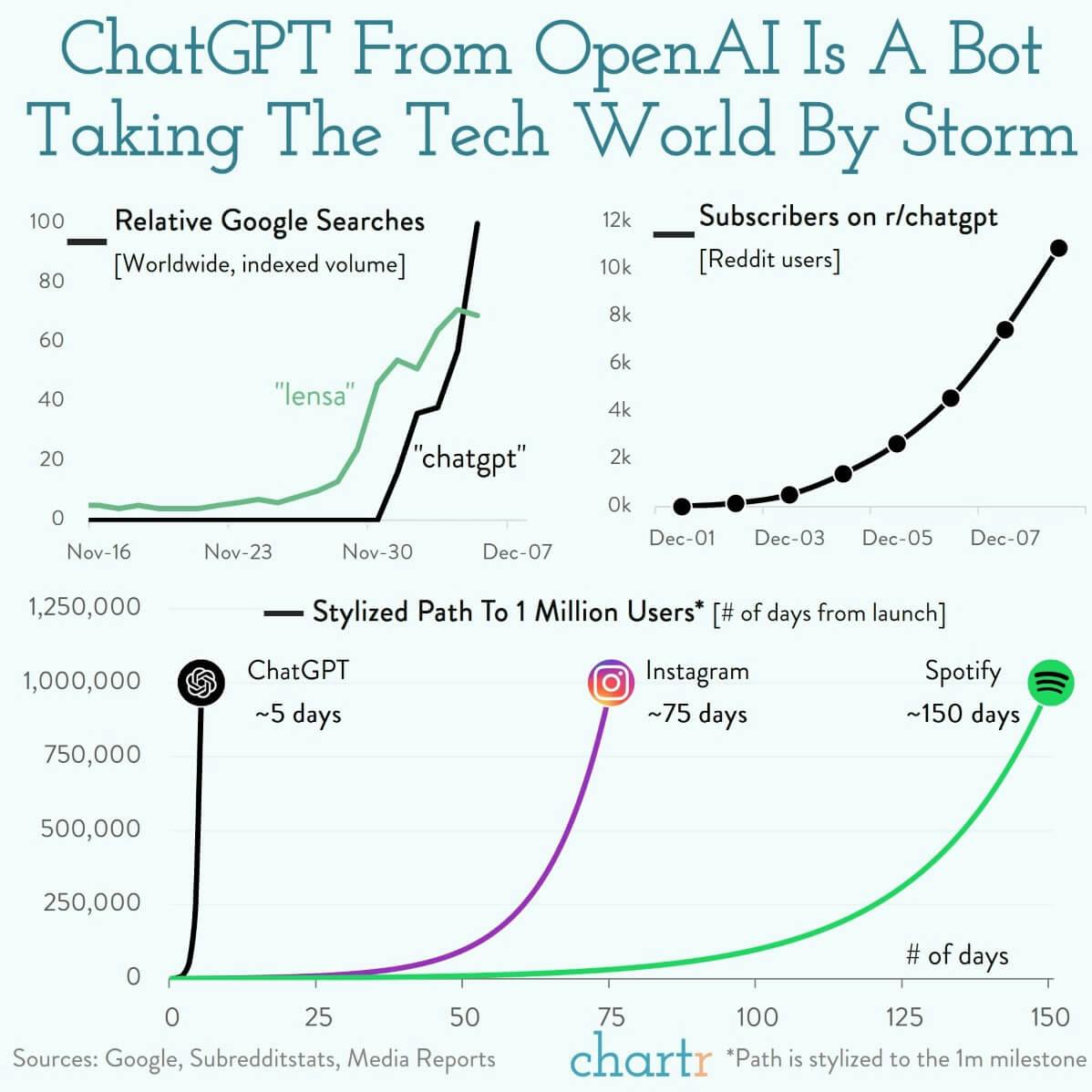
Source: Chatr
According to the OpenAI API website, companies like IBM, Salesforce, Cisco, and Intel use their API. GPT-3 has been used in companies such as Keeper Tax, Viable, Duolingo, Fable Studio, and Algolia. Codex has been used in Github Copilot. As of March 2021, nine months since the launch of the OpenAI API, more than 300 applications are using GPT-3, and tens of thousands of developers globally are building on OpenAI’s platform. Once ChatGPT becomes fully released, that usage will likely continue to increase.
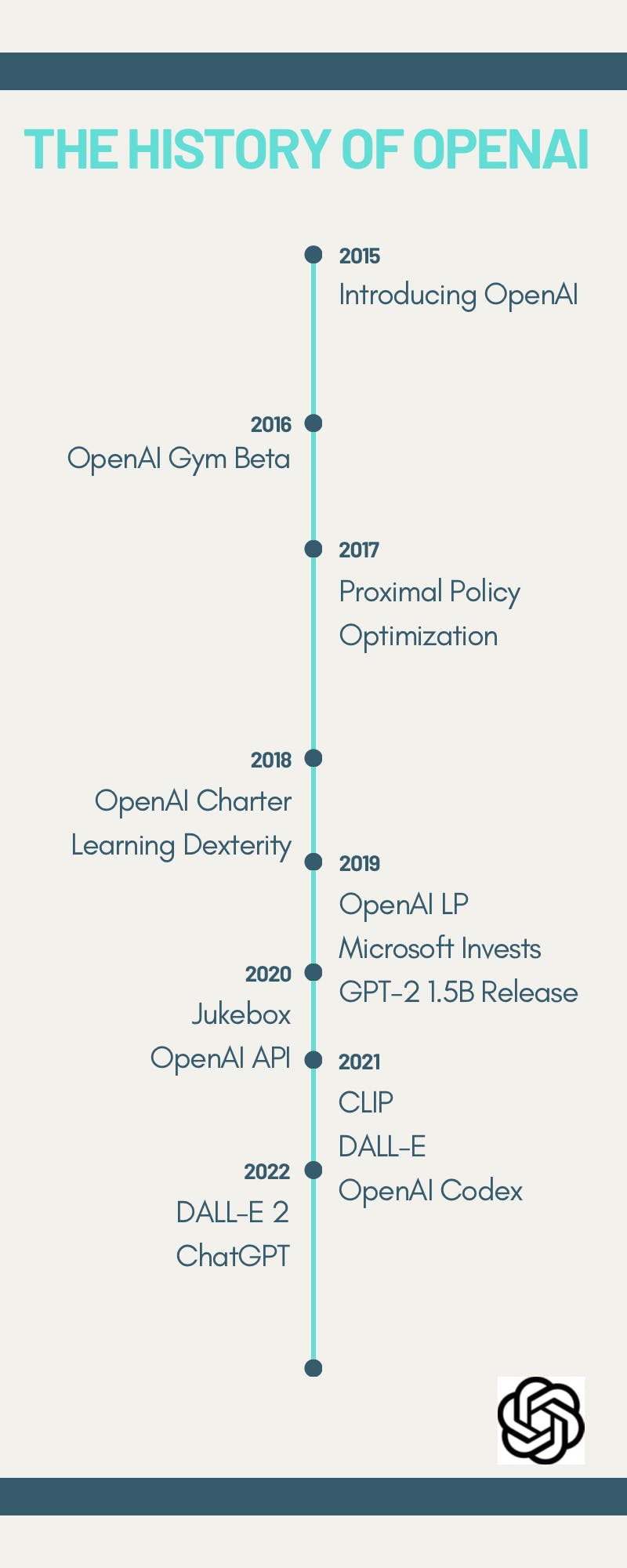
Source: OpenAI
Key Opportunities
Monetization of User Base
Tens of thousands of developers use OpenAI’s API to build or integrate new applications into their existing products. ChatGPT has drawn millions of users. However, OpenAI’s monetization efforts are still in the early days. The company has co-developed an enterprise-grade solution called Azure OpenAI Service with Microsoft. The product provides developers access to OpenAI’s popular models, such as GPT-3 and Codex, through the Azure cloud platform. However, Azure OpenAI was only available in limited access preview as of May 2022, and customers who wanted to use it had to apply for access.
The company has also made DALL-E 2, its text-to-image generating model, available to 1.5 million users who are now using it to create 2 million images per day. They also introduced a credit-based price structure for the product. Customers will get 50 free credits (the equivalent of 200 images) for the first month and 15 free credits per month afterwards. They will then have to pay $15 for every additional 115 credits. It is still beta stage and is not yet widely available as of July 2022.
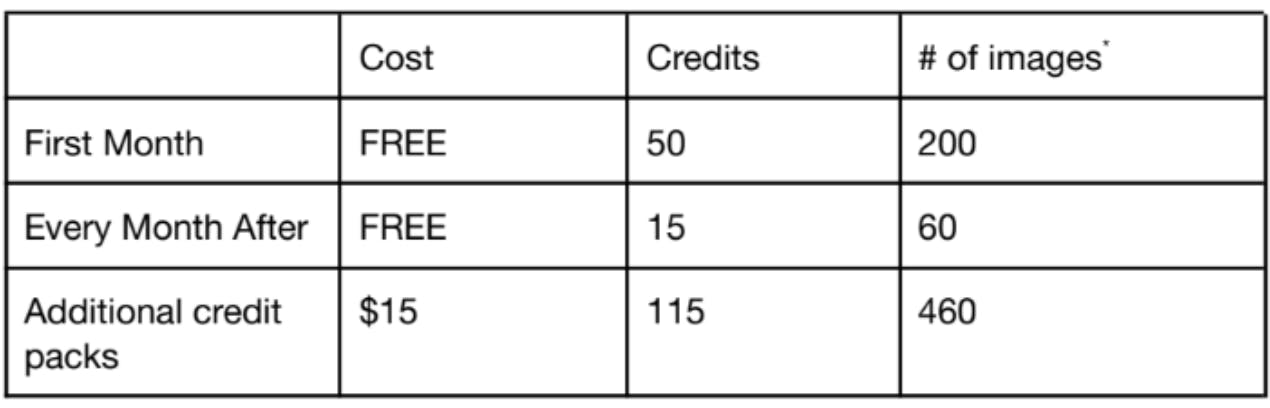
Source: Techcrunch
OpenAI has the opportunity to develop a go-to-market strategy tailored for consumers, startups, and large enterprises drowning in large unstructured datasets. Many of these companies would be eligible to use OpenAI’s models like GPT-3, Codex, and DALL-E 2 to write, code, design, and run data-driven decision-making.
Becoming The Next Search Engine
ChatGPT has the opportunity to take a cut of the search engine market. While Google would be a difficult search platform to replace, both platforms could be used complimentary of each other. Google will likely always play a role in finding information that has a high level of accuracy, authoritative queries, and information that requires citation of key sources. However, OpenAI’s ChatGPT would be a better fit for creative endeavors. It could be the platform for detailed responses, comprehensive essays, code snippets, and things that require less factual information such as the use cases outlined here. OpenAI could choose to monetize via subscribers (Netflix) or advertising (Google). In either case, the market opportunity to become a core search engine for hundreds of millions of users as the technology improves is exponential.
Data Growth & AI Adoption
IDC expects the aggregate global data to grow from 33 Zettabytes in 2018 to 175 Zettabytes (or 175 trillion gigabytes) in 2025. Experts estimate that 80% to 90% of the world’s data is unstructured, whether text, images, video, or audio-based datasets. Retail, pharma, and finance organizations are increasingly harnessing text analytics and NLP models to extract insights from their big data and applying them in use cases such as customer experience management, drug discovery, and investing, to name a few.
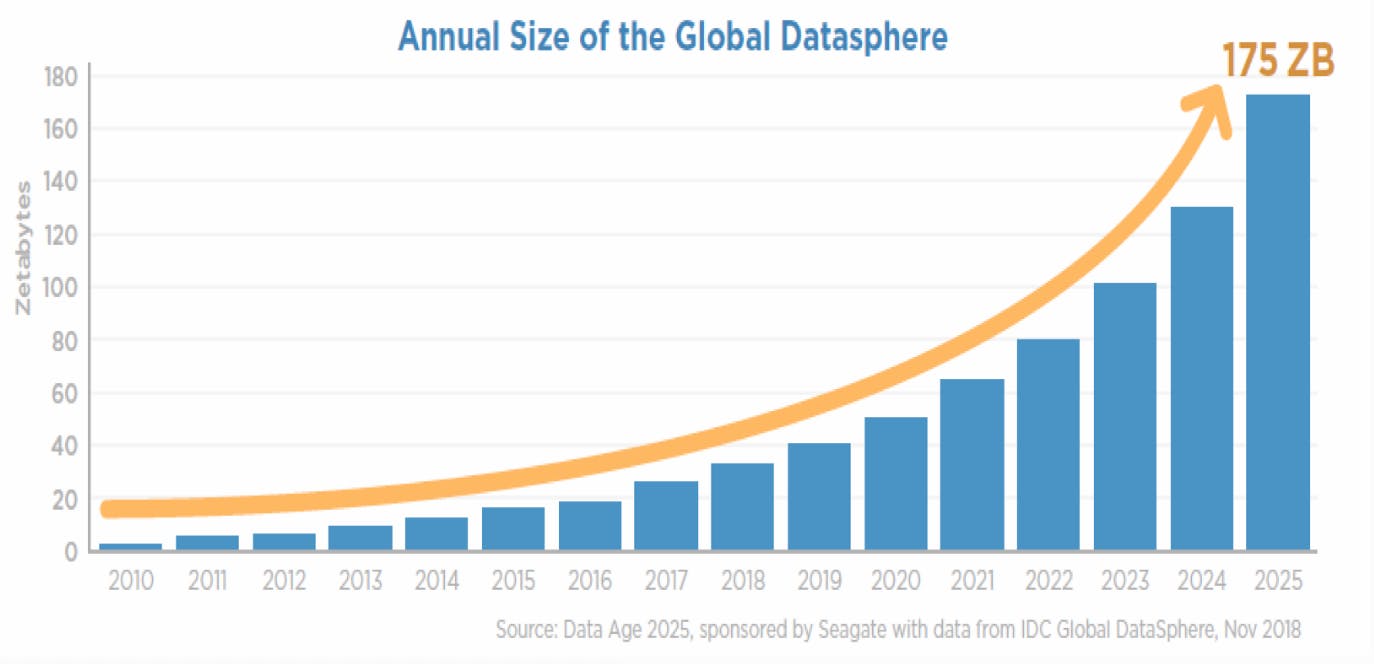
Source: IScope
56% of organizations globally adopted AI in 2021, up 6% year-over-year. The increase in adoption was mainly driven by ease of access and notable financial gains via AI implementation. More than 65% of companies reported revenue increases attributable to AI adoption. OpenAI plays an essential role in AI research and is positioned to receive a significant windfall for this trend as organizations tap into their big data to generate insights and increase their AI budgets.
Key Risks
Open Source Alternatives
There are several open source alternatives to GPT-3, such as GPT-J released in August 2021. GPT-J generally performs better than the smaller versions of OpenAI’s GPT-3 models, but is still slightly worse than the best performing GPT-3 model. For developers who can trade off accuracy for cost, they may choose open-source models over the smaller OpenAI GPT-3 models, resulting in less revenue for OpenAI. Alternatively, stable diffusion and Stability AI have proven to be effective solutions for text-to-image models. It is possible that people prefer to use these open-source versions. There are note worthy companies, like Midjourney, which is an independent research lab that produces its proprietary AI models for images in partnership with Discord.
A number of large partnerships and investments have started to lay the landscape for various solutions in the space.
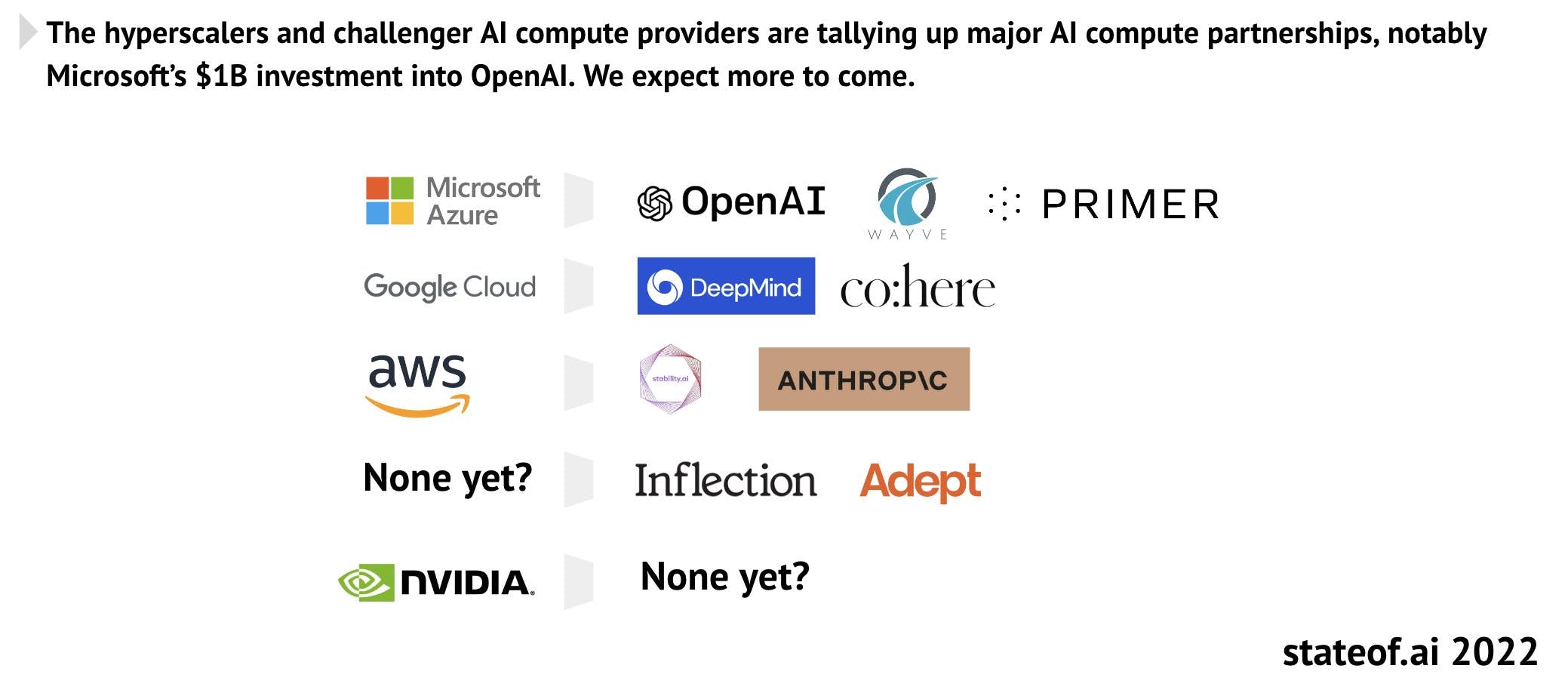
Source: State of AI Report
Model Bias
OpenAI models may generate outputs deemed untruthful, toxic, or reflect harmful sentiments. Training data usually contains many examples of bias, disinformation, and prejudice. The OpenAI team said in a paper describing the technology that “internet-trained models have internet-scale biases.” OpenAI released InstructGPT in January 2022, which is more equipped to follow user intentions than GPT-3, while making them more accurate and less toxic. OpenAI has also committed to researching trust and safety to reduce the risk that it’s models will generate racist or sexist responses, or generate disinformation. OpenAI models may be too general and require fine-tuning for specific business use cases. If businesses do not fine-tune models from OpenAI, and instead use them right out of the box, the ML model is likely not accurate enough for their use case.
Profit Incentive
There is also the risk that partnerships, such as Microsoft, will steer OpenAI’s research away from AGI and toward products with short-term business value. Microsoft may eventually consider acquiring OpenAI, similar to how Google acquired DeepMind in 2014. A commercial acquisition could distract the company from optimizing for human welfare, or openly sharing all its technology. A commercial acquisition could have a similar outcome to DeepMind, which forced the company to split its resources between AGI research and an “applied AI” division that works on creating profitable products. This means that OpenAI may end up devoting fewer resources to AI research, instead focusing on creating commercial products and being unable to reach its mission of creating AGI.
Business Model Risks
Due to the significant compute and data costs that are required to support millions of daily or monthly active user queries for multiple OpenAI products present business model risk. If progress on the quality of GPUs isn’t adequate in driving down this cost of scale, it could be difficult for OpenAI to create a viable business. To effectively monetize and perform research into large AI models, OpenAI needs to scale. According to OpenAI’s blog:
“[OpenAI will] need to invest billions of dollars in upcoming years into large-scale cloud compute, attracting and retaining talented people, and building AI supercomputers.”
Microsoft’s VP of Azure, Eric Boyd, said the following about resource optimization for the OpenAI model:
“There [are] still open questions about whether such a large and complex model could be deployed cost-effectively at scale to meet real-world business needs.”
There is a risk that these costs will outweigh the company’s revenue and funding particularly due to its capped profit structure of the business. If investors feel like profits are limited, they may be less interested in funding the company.
Building In-House Solutions
While OpenAI has been successful in offering its platform as a foundation for other solutions, like Jasper for copywriting, there is still a risk of these platforms opting to build their own models and AI expertise in-house as opposed to paying for OpenAI’s products. The more companies feel in-house operations and capabilities are critical to their long-term success, the more difficult it is for OpenAI to make the case for having its solutions embedded into other applications.
Valuation
In March 2019, OpenAI changed its company structure from a non-profit to a “capped-profit” company called OpenAI LP. Different sources estimate that the business is valued at around $20 billion. Other sources have indicated OpenAI is considering a tender valuing the company at a $50 billion valuation. As mentioned, the structure of the company ensures that economic returns for investors and employees are capped, with the cap negotiated in advance on a per-limited partner basis. Any excess returns go to the OpenAI non-profit. Returns for their first round of investors are capped at 100x their investment, and they expect the multiple to be lower for future rounds as they make more progress.
Summary
OpenAI has so far pushed the frontiers of AI research in its mission to achieve digital intelligence in an optimal way for humanity. The company has made significant progress within 7 years of starting a company that was solely meant to be a non-profit research group. GPT-3, ChatGPT, and DALL-E 2 have become notable examples of OpenAI pushing the limits of what’s possible with AI.
OpenAI has been significantly conservative with the breadth and depth of the technologies it has released to the public, highlighting that the company would rather be cautious and move slowly rather than risk causing negative outcomes from the misuse of their AI. This may also be signaling that we may not be seeing the full capability of everything OpenAI is building.
Overall, OpenAI is well-positioned to become one of the most important companies over the next 10 years as AI becomes an increasingly important part of everyone’s lives. The company will play a key role in driving research forward on AGI, driving productivity for consumers, and powering enterprise applications. The OpenAI story is just getting started.