Thesis
Since the release of Google’s Transformer model in 2017, which introduced a new method for artificial intelligence development, advanced technologies within AI have progressed rapidly. From 2018 to 2020, parameter counts in emerging large language models (LLMs) increased 1K-fold, resulting in an average 4.1x increase in training compute use every year as larger, more compute-intensive models were released. Among frontier models, the increase in training compute has been even greater. OpenAI’s GPT-2, released in November 2019, was trained with 4.3 million petaFLOPS of compute. Less than six months later, GPT-3 was developed using 314 million petaFLOPS of training compute. By 2023, GPT-4 required 21 billion petaFLOPS of training compute, while Google’s release of Gemini Ultra in late 2023 required 50 billion petaFLOPS of training compute. Over this four-year period, frontier models have increased their annual training compute usage by 2.9K times.
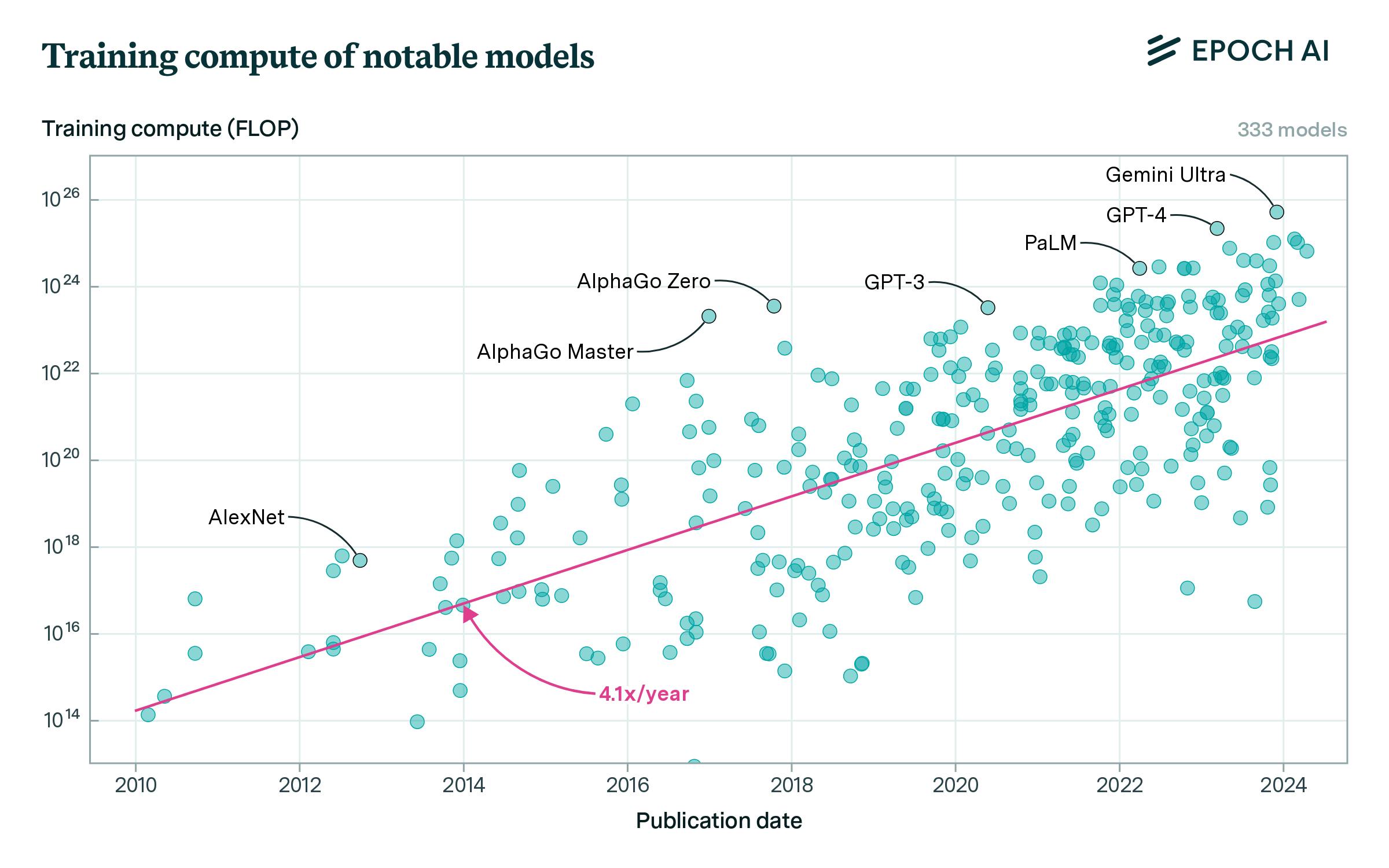
Source: Epoch AI
This surge of progress in AI technologies and the growth of larger models has been driven by the rapid adoption of AI by both consumers and businesses. It took Netflix 3.5 years to reach 1 million users. Facebook took 10 months. For OpenAI’s ChatGPT, it took five days to reach 1 million users and two months to reach 100 million monthly active users. By March 2024, 72% of organizations had adopted AI, up from 20% in 2017. Demand for AI technologies continues to grow, with the global AI market expanding at a 36.6% CAGR as education, cybersecurity, energy, gaming, automotive, healthcare, retail, finance, manufacturing, consulting, and other industry verticals continue developing and incorporating AI-powered services. However, training new AI models is complex, slow, and expensive. For example, training GPT-4 required 25K Nvidia A100 GPUs for roughly three months, costing $78 million. As models grow exponentially, companies worldwide are seeking software and hardware solutions to enable faster, simpler, or cheaper AI training.
Cerebras Systems is an AI chip manufacturer that builds what the company claims to be “the world’s largest chip”. Its flagship product, the Cerebras Wafer-Scale Engine (WSE), is described by the company as “the fastest AI processor on Earth” which “surpasses all other processors in AI-optimized cores, memory speed, and on-chip fabric bandwidth.” This chip, the size of a dinner plate, offers improved training times and simplified AI training methods at lower prices than Nvidia’s industry-standard GPU chips. Cerebras offers both on-premise deployment and cloud use of its computing systems for companies worldwide seeking to train AI models.
Founding Story
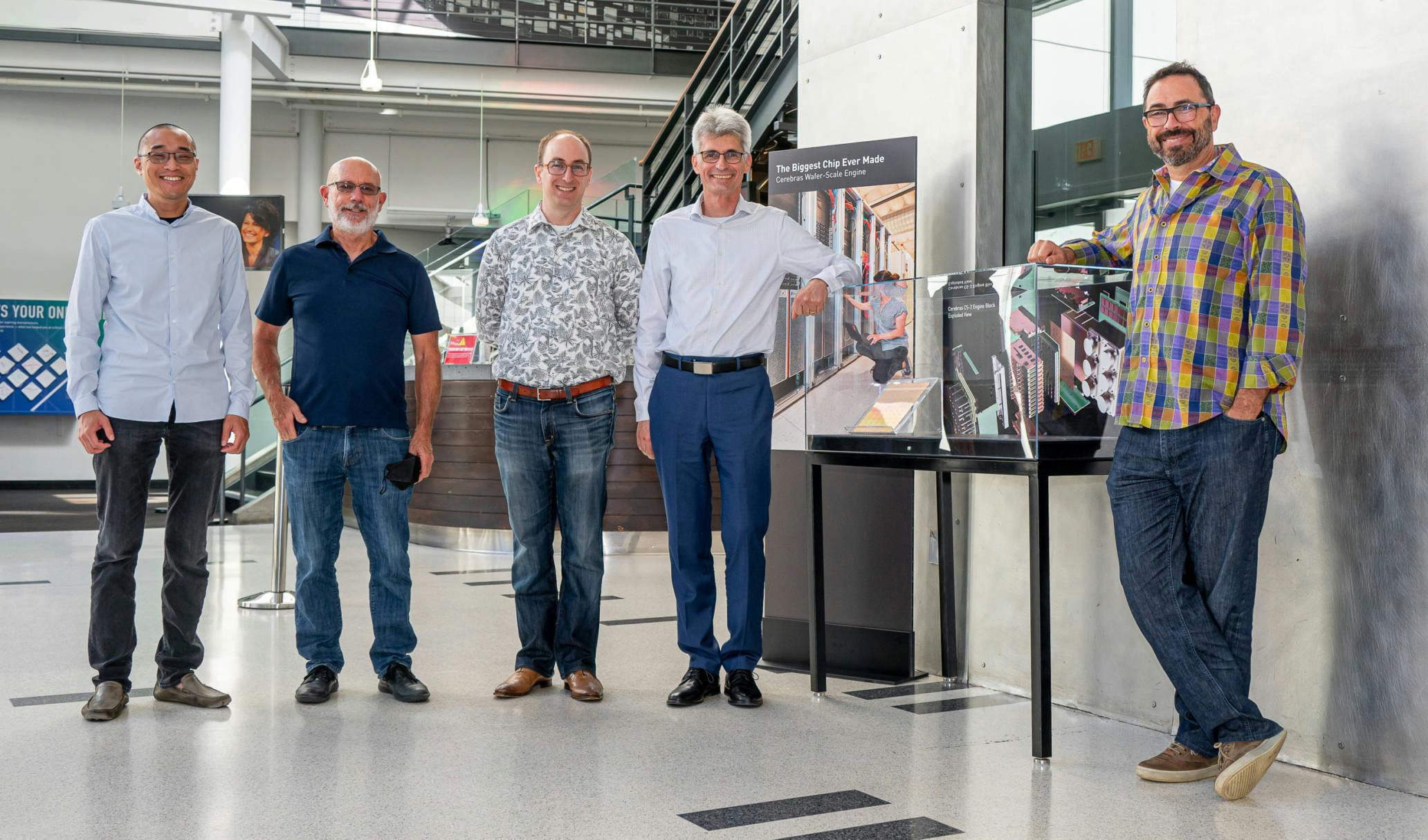
Source: Cerebras
Cerebras Systems was founded in 2016 by Andrew Feldman (CEO), Gary Lauterbach (CTO, retired), Michael James (Chief Software Architect), Sean Lie (Chief Hardware Architect and current CTO), and Jean-Philippe Fricker (Chief System Architect). Prior to founding Cerebras, they had all worked together at Feldman and Lauterbach’s previous startup, SeaMicro, until it was acquired by AMD in 2012 for $334 million.
Prior to Cerebras, Feldman had not only co-founded and sold SeaMicro, which produced energy-efficient servers, to AMD in 2012, but was also the Vice President of Product Management at Force10 Networks (which later sold to Dell Computing for $700 million), Vice President of Marketing at Riverstone Networks (which IPOed in 2001), and co-founded three other companies. Feldman’s first company, a gigabit ethernet company, was founded while he was still pursuing an MBA at Stanford and sold for $280 million a year later.
Meanwhile, Lauterbach held 58 patents in computing, with his inventions present within every chip and processor used. Previously, Lauterbach worked at Sun Microsystems, where he was the Chief Architect for the UltraSPARC III and UltraSPARC IV microprocessors and the DARPA HPCS Petascale computing project, a large supercomputer. At SeaMicro, Lauterbach was the principal investigator for a $9.3 million Department of Energy grant. Following SeaMicro’s acquisition by AMD, Lauterbach became the CTO for AMD’s data center server business.
The three other co-founders also had extensive experience in the computing industry. Lie earned both a Bachelor's and a Master's degree in electrical engineering and computer science from MIT. After graduating, he spent five years at AMD on its advanced architecture team before joining SeaMicro as the lead hardware architect. After SeaMicro was acquired, Lie became the Chief Data Center Architect at AMD. At Cerebras, he holds 29 patents in computer architecture. James was previously the Lead Software Architect at SeaMicro before becoming the Lead Software Architect at AMD. Finally, Fricker was the Senior Hardware Architect at DSSD (acquired by EMC in 2014), the Lead System Architect at SeaMicro, and holds 30 patents in computing systems.
In late 2015, the five co-founders got together after their experience at SeaMicro and wrote on a whiteboard that they wanted to work together again to transform the computing industry with the goal of being recognized in the Computer History Museum. Feldman later stated, “We were doing this so if there was to be a book on 21st century compute, there’d be a chapter on us, not a footnote.”
They began by exploring the future applications of computing, and soon honed in on the emerging developments in GPU-trained deep-learning models following the AlexNet paper. They were curious how Nvidia’s graphics processing unit (GPU), developed 25 years ago, was able to train deep-learning models. Within the AlexNet paper, the team read that there was almost no limit to how much data and computational power one could use to train a bigger and better model. This idea captivated the Cerebras team — they believed that this would lead to a sizable opportunity to build something specifically for a large computational space.
The team observed that GPUs for AI computing were not actually as efficient as they could be since the chip’s memory was located far from the GPU. Even a tiny model like AlexNet required two GPUs. While this was adequate for pushing pixels onto a screen, AI computing involves frequent data access and manipulation, relying heavily on close memory proximity. Otherwise, latency increases, resulting in memory bottlenecks. The team theorized that the solution was a bigger chip that integrated the memory directly within the GPU. However, for the past 70 years, no one in the computing industry had overcome the complexities of building large chips. Feldman recalled that not even Intel or Nvidia could overcome this challenge, despite having workforces consisting of tens of thousands of employees.
The five co-founders began by studying previous failures in building large chips, notably meeting with individuals who had worked at Trilogy Systems. In the 1980s, Trilogy Systems raised over $200 million to design a computer chip that was 2.5 inches on one side. At that time, computer chips could only be reliably manufactured up to 0.25 inches on a side. They also talked to individuals in the military and intelligence community who had worked on large chips but were told that a substantially larger chip wasn’t possible.
Most teams would have given up at that point, but Feldman was undeterred, believing they “had a world-leading team in the computing industry.” By late 2016, the company raised its Series A round, securing over $25 million in funding. Eventually, the team found other like-minded engineers and entrepreneurs who joined the company – the first 20 to 30 employees had actually worked at SeaMicro. Three years and 100 people later, in 2019, Cerebras publicly revealed WSE-1, a chip that was 56.7 times larger than the next largest GPU. In 2022, the Computer History Museum honored Cerebras by unveiling a display of its WSE-2 chip for “marking a key milestone in computer history.”
Cerebras added Dhiraj Mallick as COO in September 2023, Julie Shin Choi as CMO in November 2023, and Bob Komin as CFO in April 2024. Mallick was previously Vice President of Engineering at SeaMicro, CTO of Intel Data Centers, and Corporate VP at AMD before joining as the Senior Vice President of Engineering and Operations at Cerebras. Choi previously held product marketing roles at Hewlett Packard, Mozilla, and Yahoo, and prior to Cerebras was the CMO at MosaicML, helping it grow more than 10x before its $1.3 billion sale to Databricks in July 2023. Finally, Komin was previously the CFO of Sunrun Inc. for five years, where he led its IPO and growth from 100K customers to 500K customers to become the largest US residential solar company. Komin was also the CFO of Flurry Inc. (acquired by Yahoo for $240 million), Ticketfly (acquired by Pandora for $450 million), Tellme Networks (acquired by Microsoft for $800 million), and Solexel.
In August 2024, Glenda Dorchak, a former IBM and Intel executive, and Paul Auvil, former CFO of VMware and Proofpoint, joined Cerebras’ Board of Directors.
Product
Wafer-Scale Engines
Cerebras’ core technology is its wafer-scale engine (WSE) chips, specifically designed for training AI models and running AI inference. All chips begin their lives on a 12-inch circular silicon wafer. Whereas traditional chips are printed onto a wafer by the hundreds in a grid pattern to be cut into smaller individual chips, Cerebras’ wafer-scale integration process skips the step of cutting the wafer into many smaller individual chips. Instead, Cerebras designs the entire silicon wafer to become a single chip, hence the term “wafer-scale” engines. As a result, Cerebras’ WSE chips are approximately the size of a dinner plate.
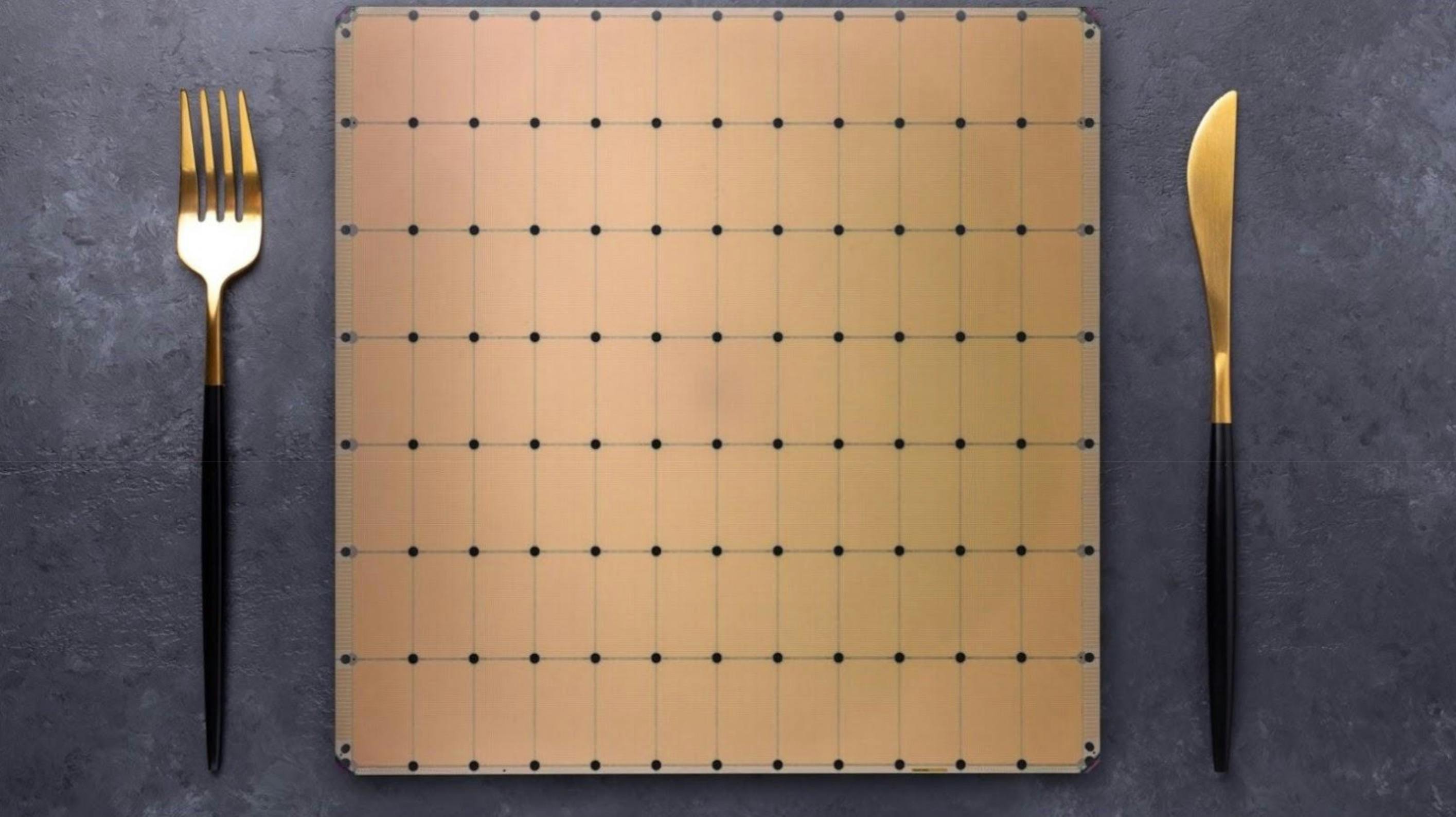
Source: Computer History Museum
To understand why, a simplified understanding of the two major time components involved in machine learning or AI development is necessary. The first is the compute power (in FLOPs) to process millions of matrix multiplication calculations. The second is the memory (in bandwidth) to update weights between connections in a model through various normalizations, SoftMax, or ReLU operations.
Advancements in computing power have historically followed Moore’s Law, but memory bandwidth has barely improved. From Nvidia’s A100 in 2020 to its H100 in 2022, compute power grew roughly 6x while memory bandwidth only grew by 1.7x. The dominant factor in training time shifted from compute power to memory size. The company’s wafer-scale engine was designed to solve this problem, a workaround to the main bottleneck of bandwidth.
Traditional processor chips are efficient at data computation but have limited internal memory to store the data used for computation. For graphics applications, this wasn’t a problem. However, as computational tasks, such as training an LLM model, grow in size, processor chips constantly need to stream data in and out of off-chip memory.
The communication speed between the processor and memory chips is slow relative to the computing speed of the processor, leading to the memory bottleneck problem. Chip-to-chip data communication is over 100 times slower than on-chip communication. By making larger chips, there is more room for processor chips, local memory chips, and the tens of thousands of silicon wires necessary for on-chip communication between the two. This design allows the WSE chip to bypass the interconnect bottlenecks that companies like Nvidia and AMD face when connecting GPUs together.
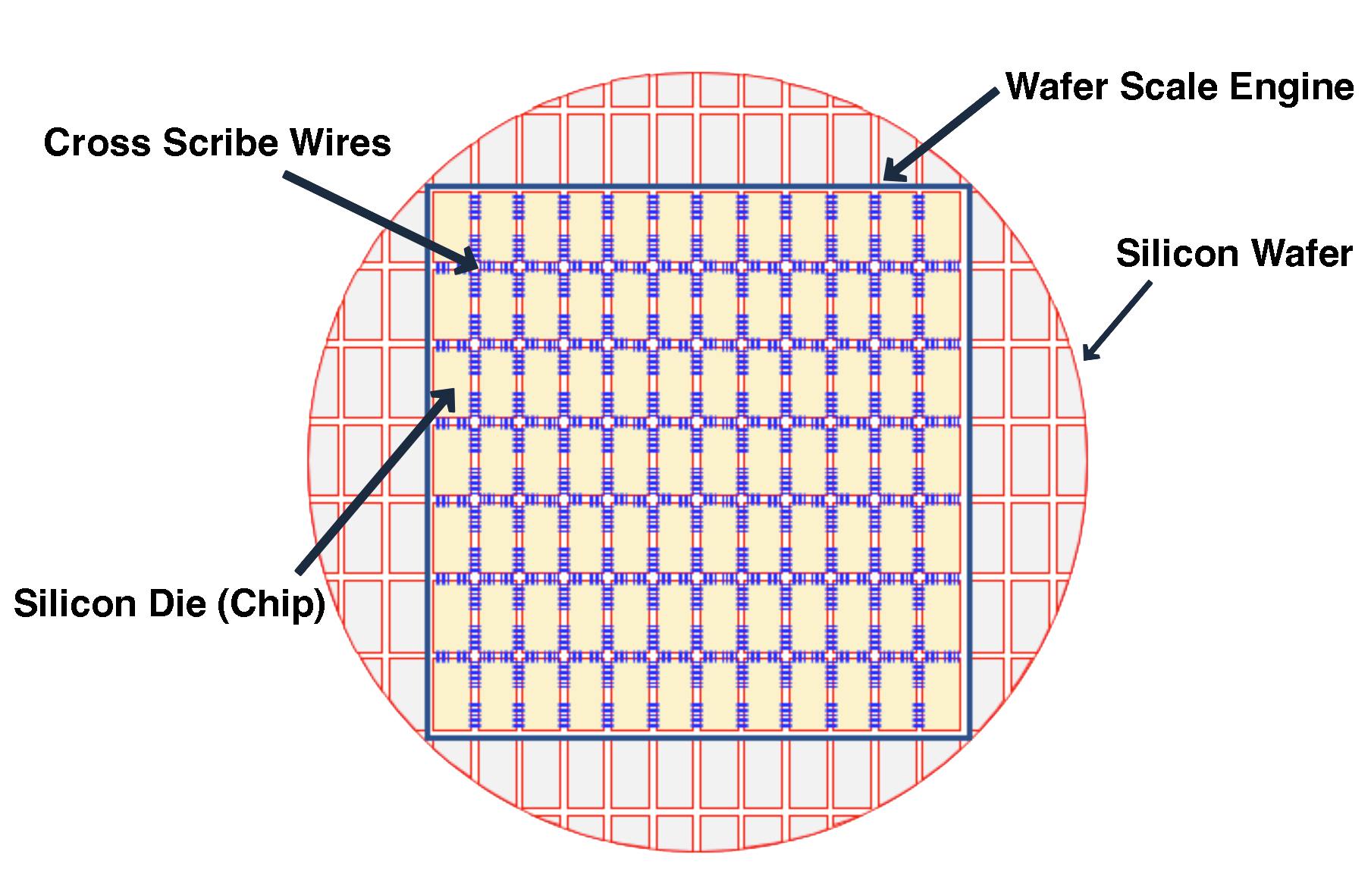
Source: Cerebras, Contrary Research
The challenge with making a large chip is that some dies turn out to be defective, leading to the entire chip being discarded. This was the problem unsolved by Trilogy Systems in the 1980s but advances in fabrication processes and lithography equipment have reduced the number of defects and allowed individual dies to be wired around defective ones, a standard process in memory chip manufacturing. This allows Cerebras to feasibly manufacture its WSE chips without a single defective die compromising the entire chip. Still, Cerebras cautiously adds redundant cores to prevent a single defect from affecting the whole chip.
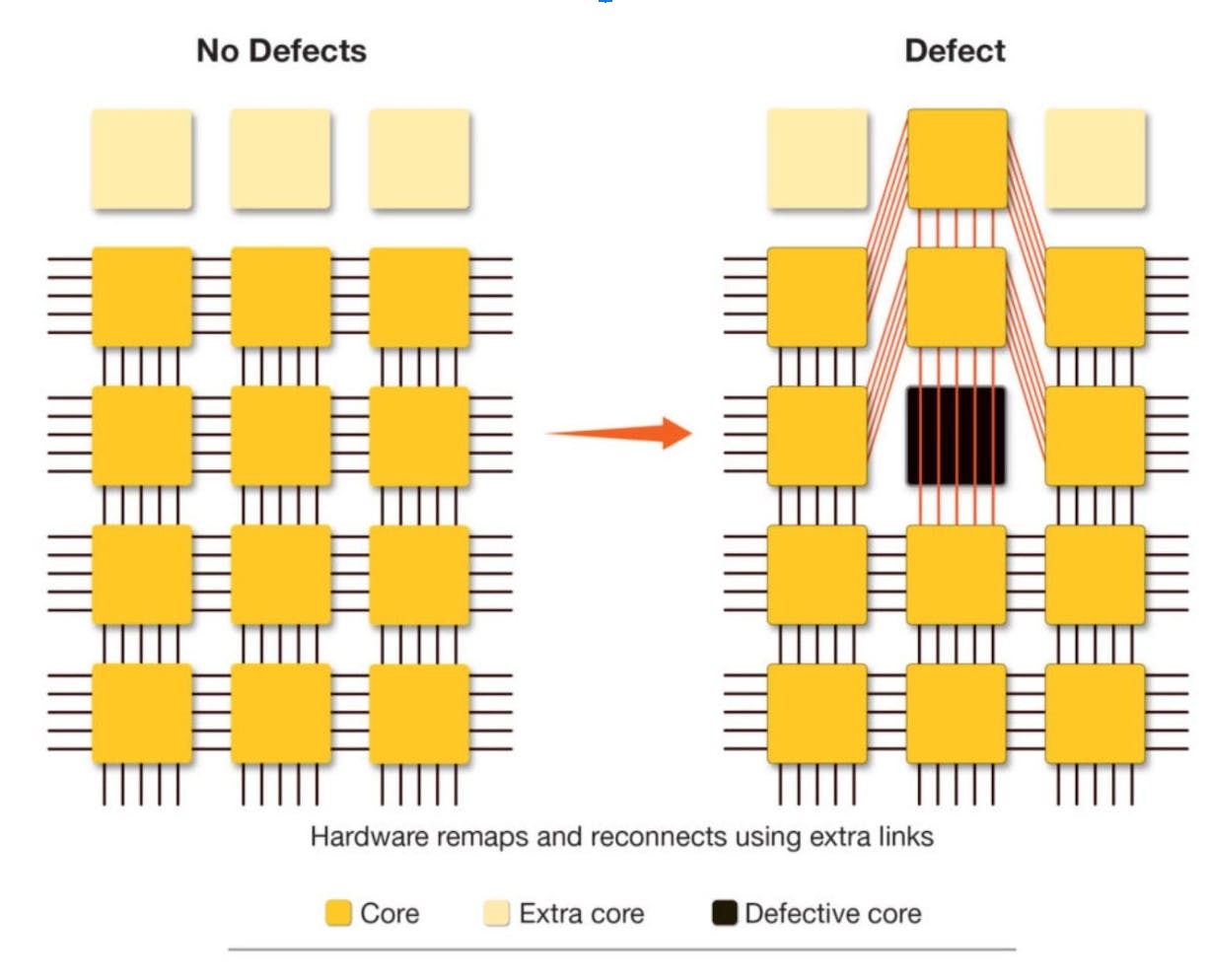
Source: Cerebras
Cerebras has released three versions of its WSE chips: WSE-1, WSE-2, and WSE-3. In 2024, Cerebras unveiled WSE-3 with four trillion transistors and 900K compute cores, capable of training AI models 13 times larger than OpenAI’s GPT-4. Meanwhile, Nvidia’s H100 GPU is 56 times smaller with 50 times fewer transistors.
Each core, known as a Sparse Linear Algebra Compute (SLAC) core, is optimized specifically for AI workloads. These cores are highly efficient at computing linear algebra and matrix multiplications that are the foundation of all neural network computation, allowing the SLAC cores to achieve performance often double or triple that of a GPU. This also ensures these cores can run newly developed neural network algorithms if they remain based on linear algebra principles.
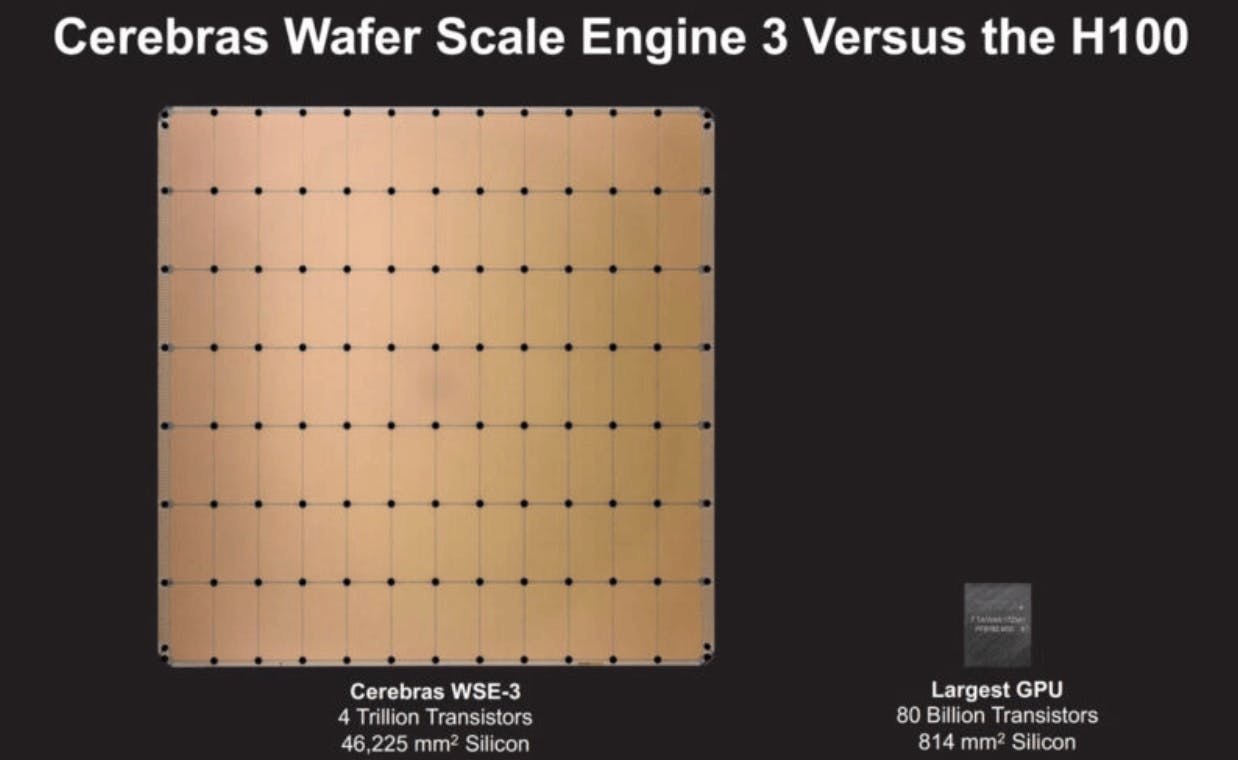
Source: Tom's Hardware
Cerebras’ earlier WSE-1 and WSE-2 chips were announced in 2019 and 2021, respectively. The WSE-1 contained over 1.2 trillion transistors and was roughly 46K square millimeters in size. In comparison, the next largest GPU at the time had only 21.1 billion transistors and was 815 square millimeters. The release of WSE-2 more than doubled the number of transistors to 2.6 trillion and delivered greater compute performance with less space and power than any other system available at the time.
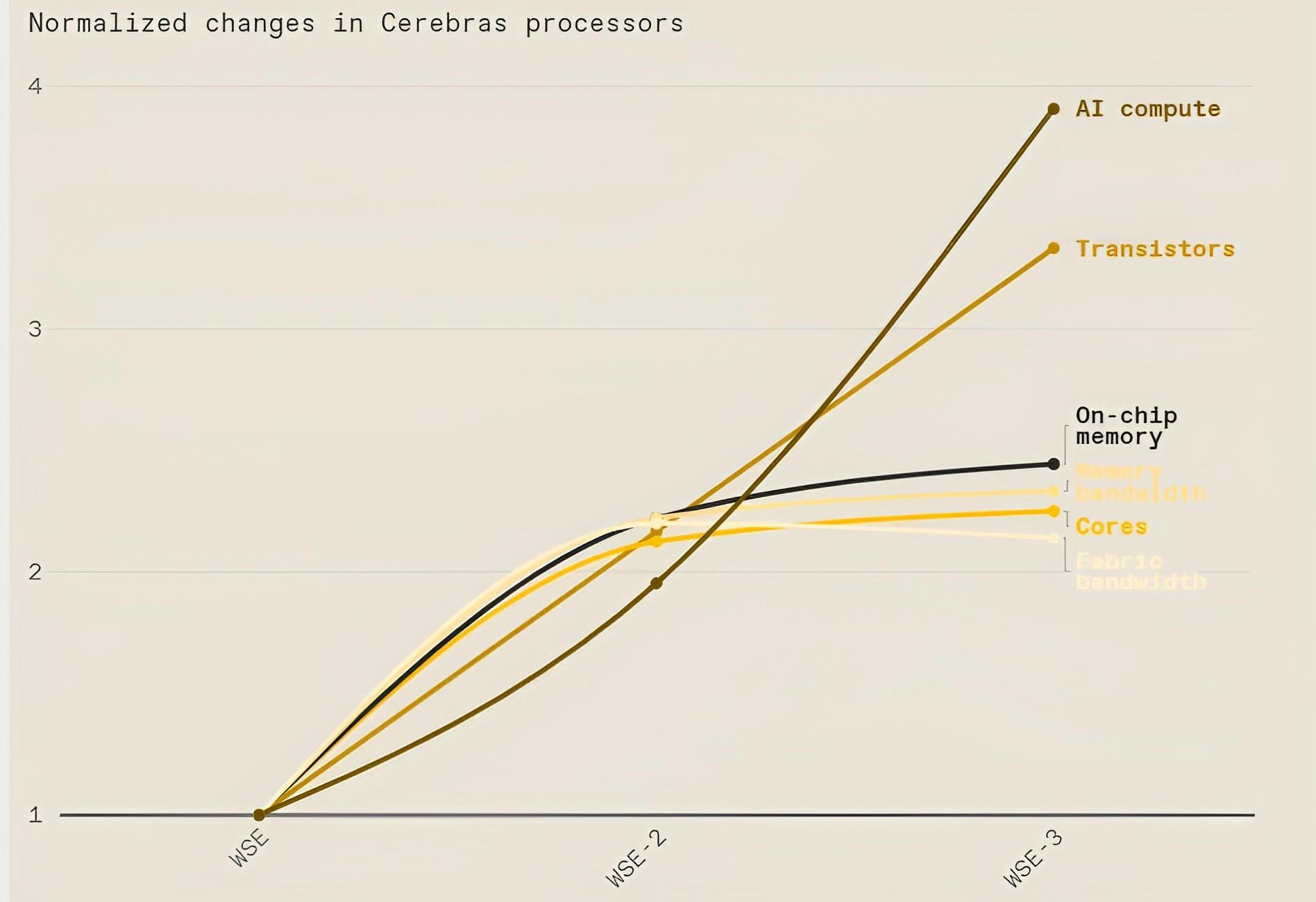
Source: Rhymes With Haystack
Cerebras Computing Systems
Unlike Nvidia or AMD, Cerebras does not sell individual WSE chips. Cerebras instead sells integrated computing systems, tuned and co-designed specifically with its WSE chips. To date, Cerebras has developed three computing systems: CS-1 for the WSE-1, CS-2 for the WSE-2, and CS-3 for the WSE-3.
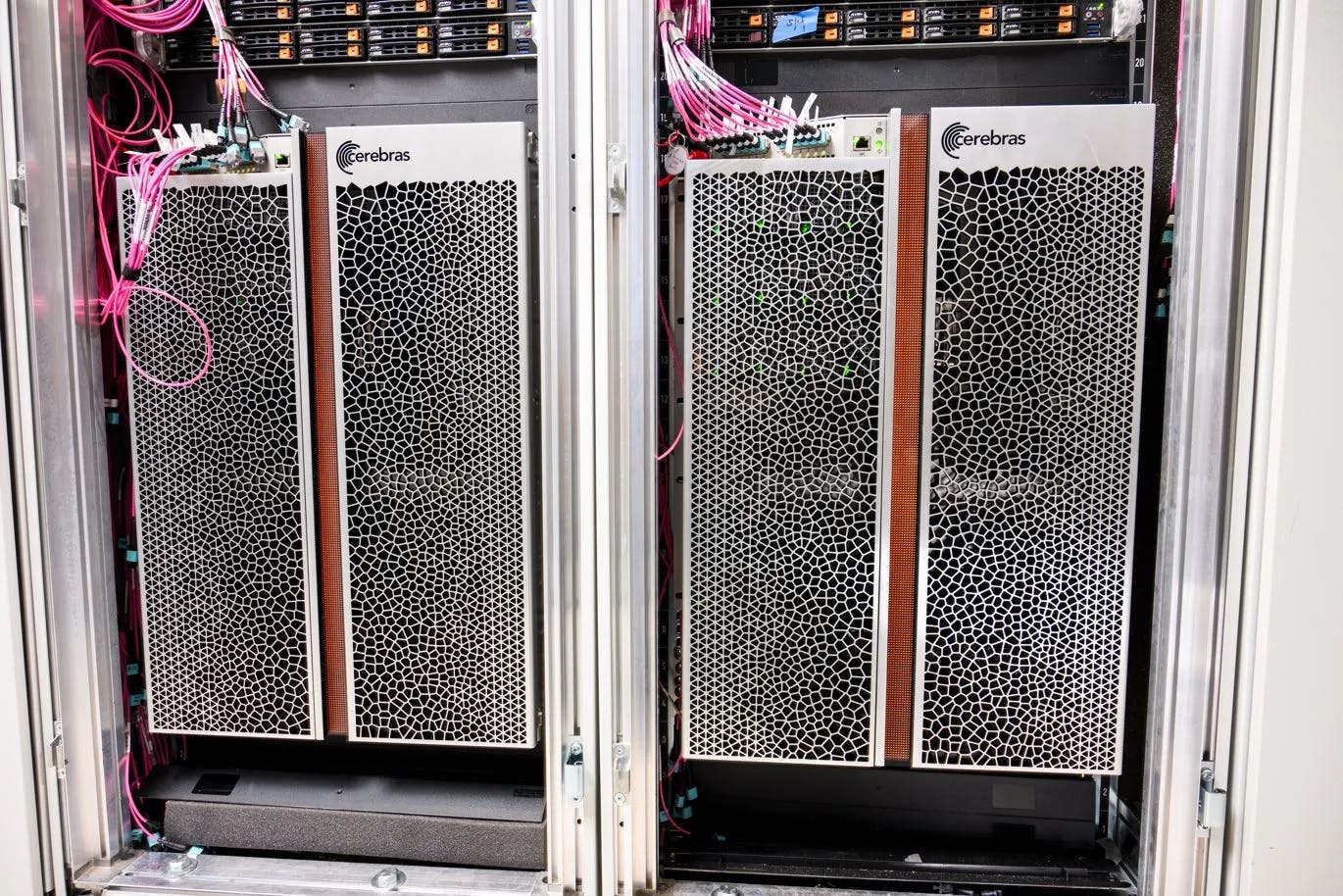
Source: EE Times
Each system contains an engine block that includes the packaging, power system, and a closed internal water loop to cool the power-intensive WSE chips. Additionally, all cooling and power supplies are built to be redundant and hot-swappable.
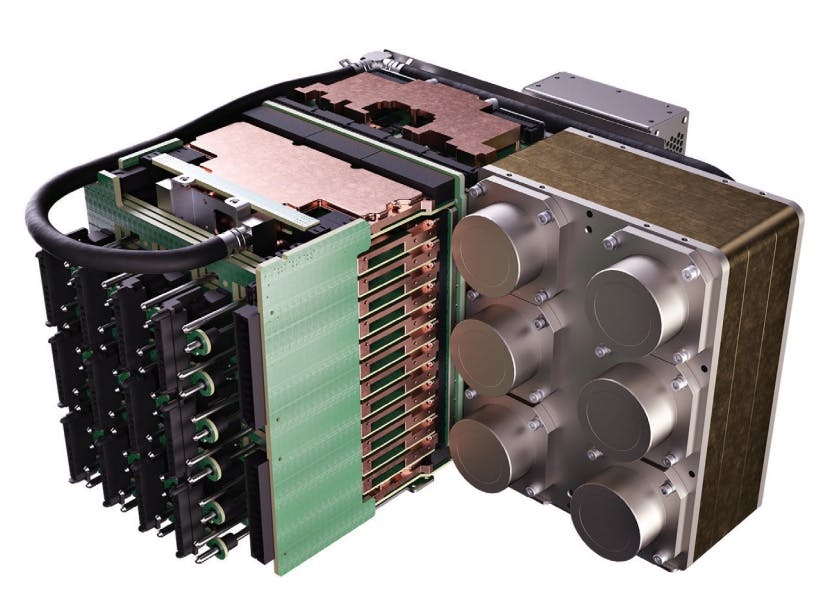
Source: Cerebras
Each of these computing systems is designed to scale using Cerebras’ SwarmX and MemoryX technologies. SwarmX enables up to 192 CS-2s and 2,048 CS-3s to be connected for a near-linear gain in performance. Triple the number of CS-3s and an LLM model is trained three times faster. This is not true for Nvidia, which acquired Melanox for $7 billion in 2019 to connect its GPUs for a sublinear gain in performance.
An individual CS-2 system, CS-3 system, or a SwarmX system can then be paired with a single MemoryX unit, adding up to 1.2 terabytes of memory storage per computing system, allowing each system to store 24 trillion parameters of an LLM. During training, the MemoryX unit can store all model parameters off-chip while delivering on-chip performance. Using a full cluster of 2,048 CS-3s, 256 exaflops of AI compute can train a Llama2-70B model from scratch in less than a day.
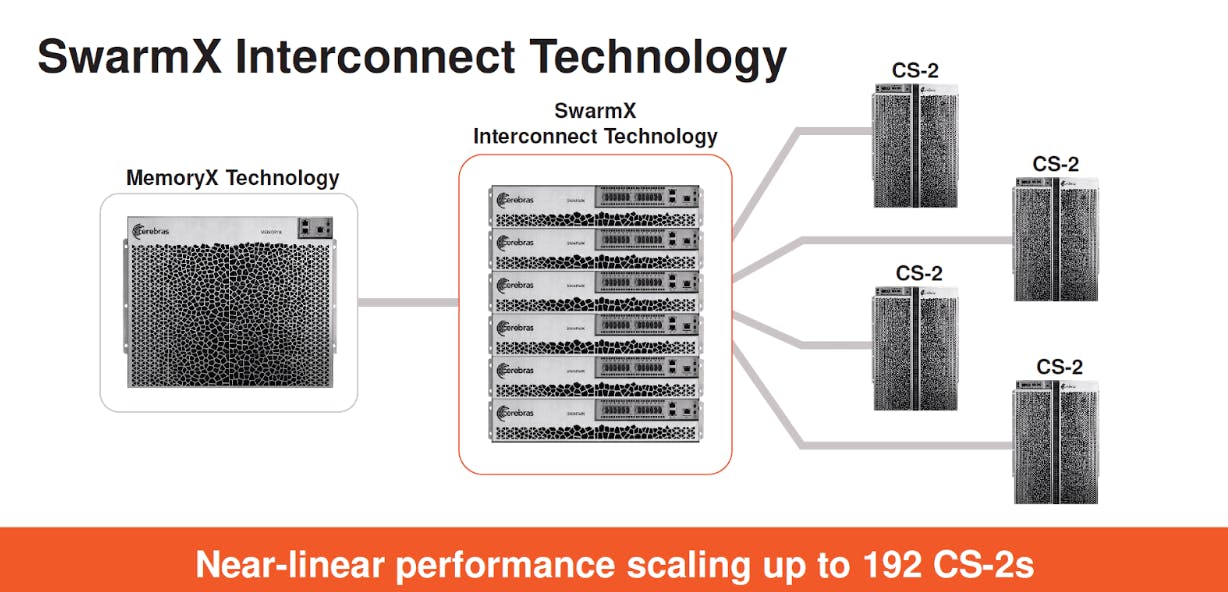
Source: Cerebras
By decoupling memory and compute, both can be scaled independently based on user needs. In contrast, the memory and compute capabilities of a GPU are fixed. For example, Nvidia’s current flagship H100 chip is limited to 80GB of memory. As a result, a GPU cluster is needed to divide an LLM into many smaller parts among the thousands of individual GPUs connected by Nvidia’s NVLink, forcing developers to manage model distribution across an “ocean of processors.” As a result, machine learning developers and researchers must write software to address this distributed compute problem, significantly increasing both the amount and complexity of the code.
This would be acceptable if distributed training were not so difficult to implement, even for experienced developers. Andrej Karpathy, co-founder of OpenAI, has said that managing thousands of GPUs can be a “frustrating daily life experience” in training large models. Similarly, Ivan Zhou, a senior model engineer at Uber, has said that building reliable training clusters from scratch is “damn hard.” This results in many developers working on solving the distributed training problem, increasing the time and complexity needed to train an LLM. Training GPT-1 required four contributors to implement the entire model. By GPT-4, 240 contributors were needed, 35 of whom were dedicated solely to the distributed training processes.
Although Nvidia has developed pre-packaged distribution systems, machine learning developers still need to manage the model distribution strategy themselves. This includes tasks such as distributed data parallelism, pipeline parallelism, tensor parallelism, expert parallelism, interleaved pipelining schedules, activation checkpointing, and recomputation – all of which are relatively time-consuming and difficult to implement, requiring additional hundreds of lines of code.
Because a single Cerebras computing system can be customized to hold and train an entire trillion-parameter model, no distributed training software is needed, reducing up to 97% of the code required to develop a model. On a single Cerebras CS-3s, a standard implementation of a GPT-3 sized model required just 565 lines of only Python code within a day, while implementing GPT-3 on Nvidia’s GPUs required 20K lines of code over weeks to implement with Python, C, C++, and CUDA.
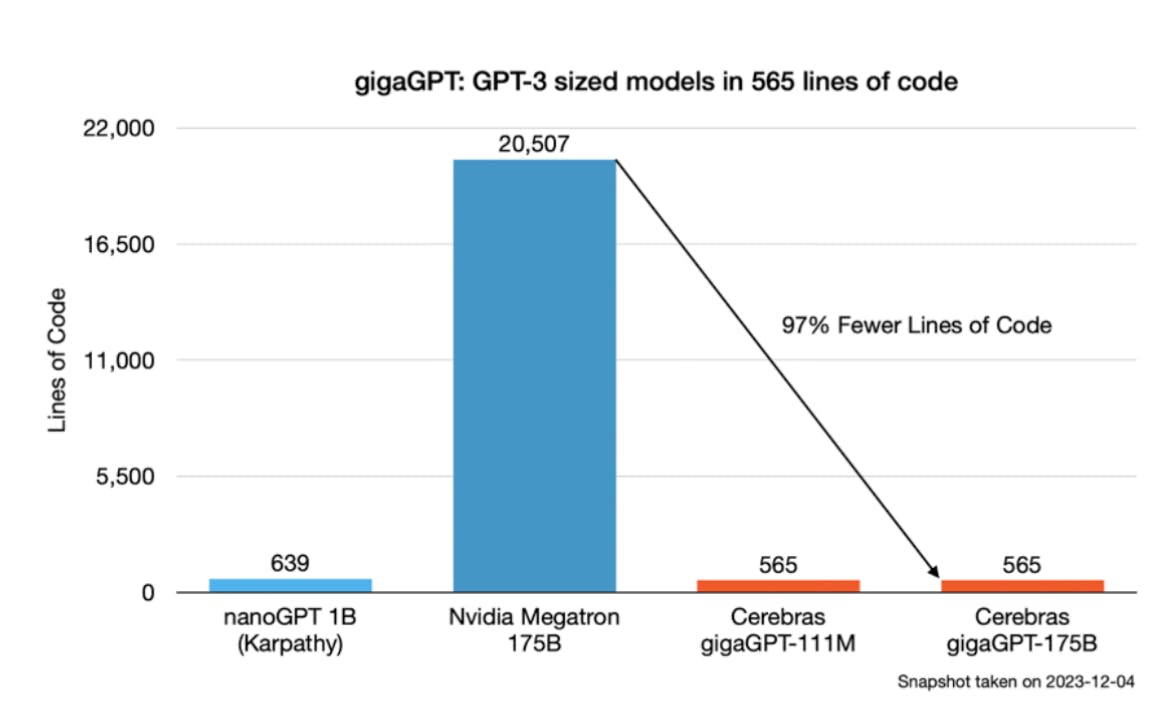
Source: Forbes
Even when 2,048 Cerebras CS-3 systems are connected into a larger cluster, Cerebras’ SwarmX and MemoryX technologies enable these interconnected individual systems to still function as a single cohesive device. Across any configuration of Cerebras’ computing systems, not a single line of code is needed to enable distributed compute.
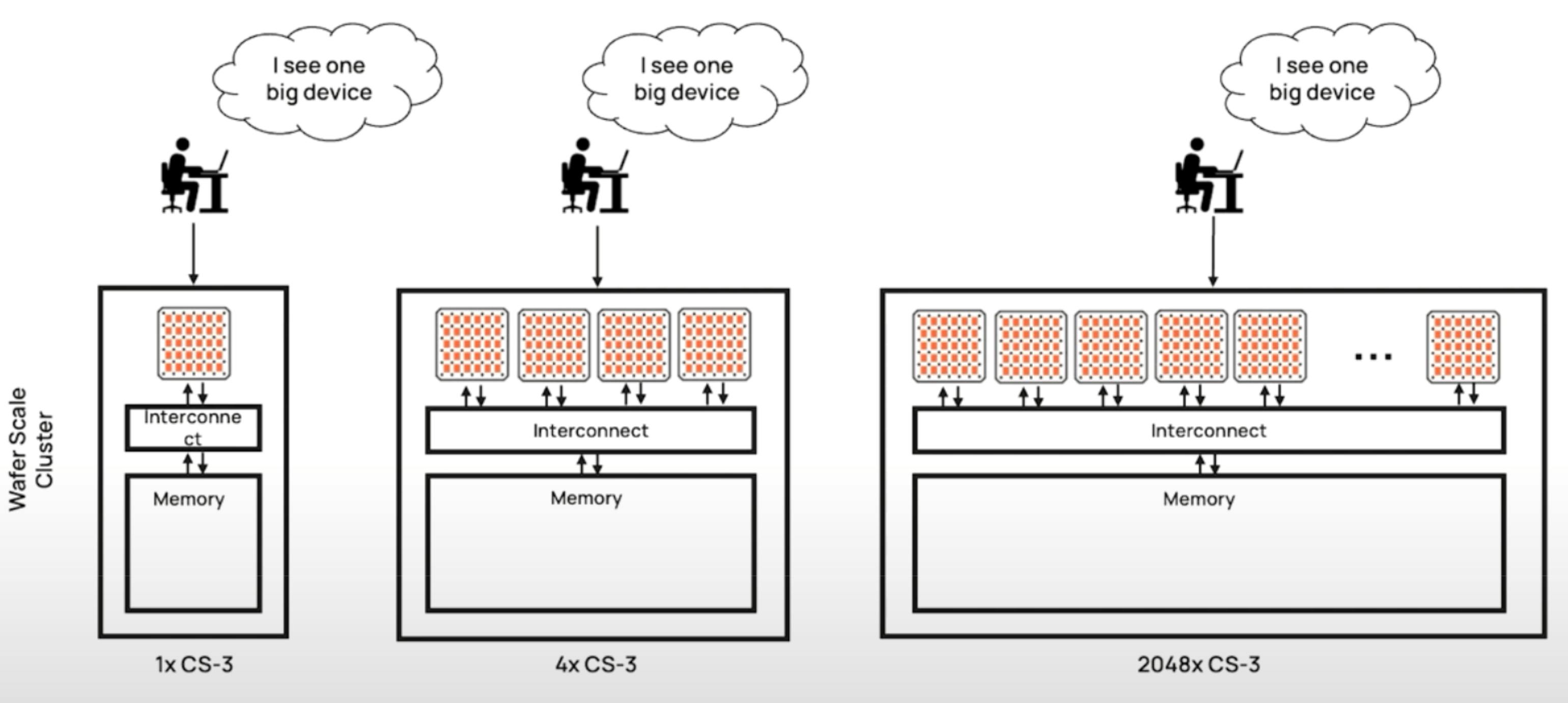
Source: Cerebras
Although Cerebras’ computing systems can be connected together, each individual system is still reportedly more powerful than a typical GPU rack. The original CS-1, released in November 2019, was already 10K times faster than a GPU and 200 times faster than the Joule Supercomputer, ranked 181 among the top 500 supercomputers in the world. The CS-2, released in 2021, doubled the performance of its predecessor, the CS-1. The CS-3 was released in 2024 and doubled the performance of the CS-2 with no increase in power or cost. A single CS-3 system, the size of a dorm room mini-fridge, typically delivers the computing performance of an entire room of servers with tens to hundreds of GPUs. Together, a Cerebras cluster of 48 CS-3s exceeds the performance of the US’s Frontier – the world's #1 supercomputer — while being 100 times cheaper.
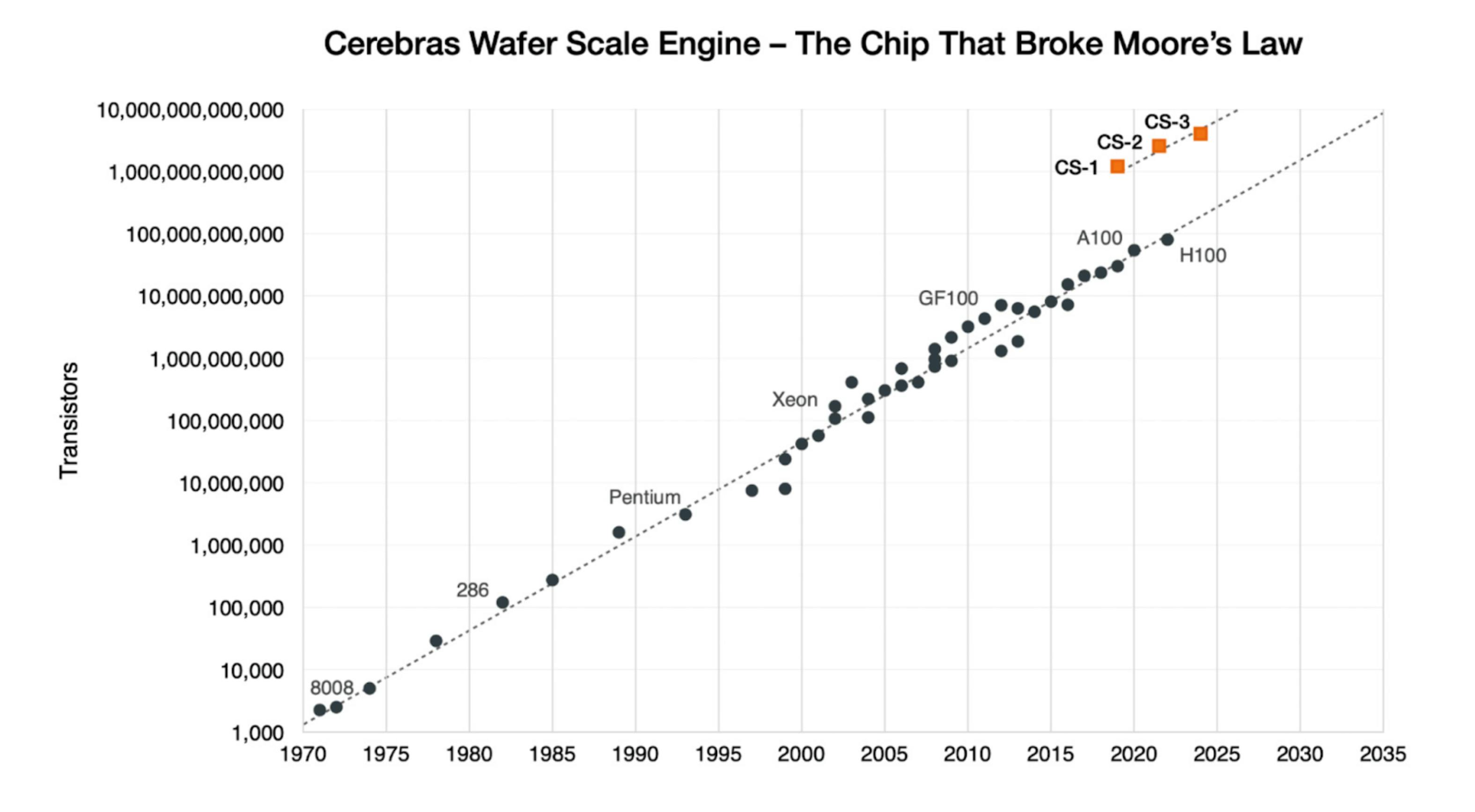
Source: Cerebras
In a real-world use case, a single CS-2 simulated real-time computational fluid dynamics 470 times faster than a conventional supercomputer, enabling accelerated research in the energy sector and climate tech sector. At Argonne National Laboratory, Cerebras’ CS-1s were 300 times faster than the existing compute infrastructure for cancer research. At GlaxoSmithKline, Cerebras’ CS-1s accelerated epigenetic research by 160 times. The French energy giant TotalEnergies found Cerebras’ CS-2s to be 100 times faster for a key element in oil exploration. In stencil-based computation used in various scientific fields such as seismic imaging, weather forecasting, and computational fluid dynamic simulations, a Cerebras’ CS-2 was 95 times faster than an Nvidia H100 chip and 292 times faster than an AMD MI210 chip. Finally, one research group found that training specific models was impossible on GPU clusters due to “out-of-memory errors,” and had to use Cerebras’ CS-2s to complete their model.
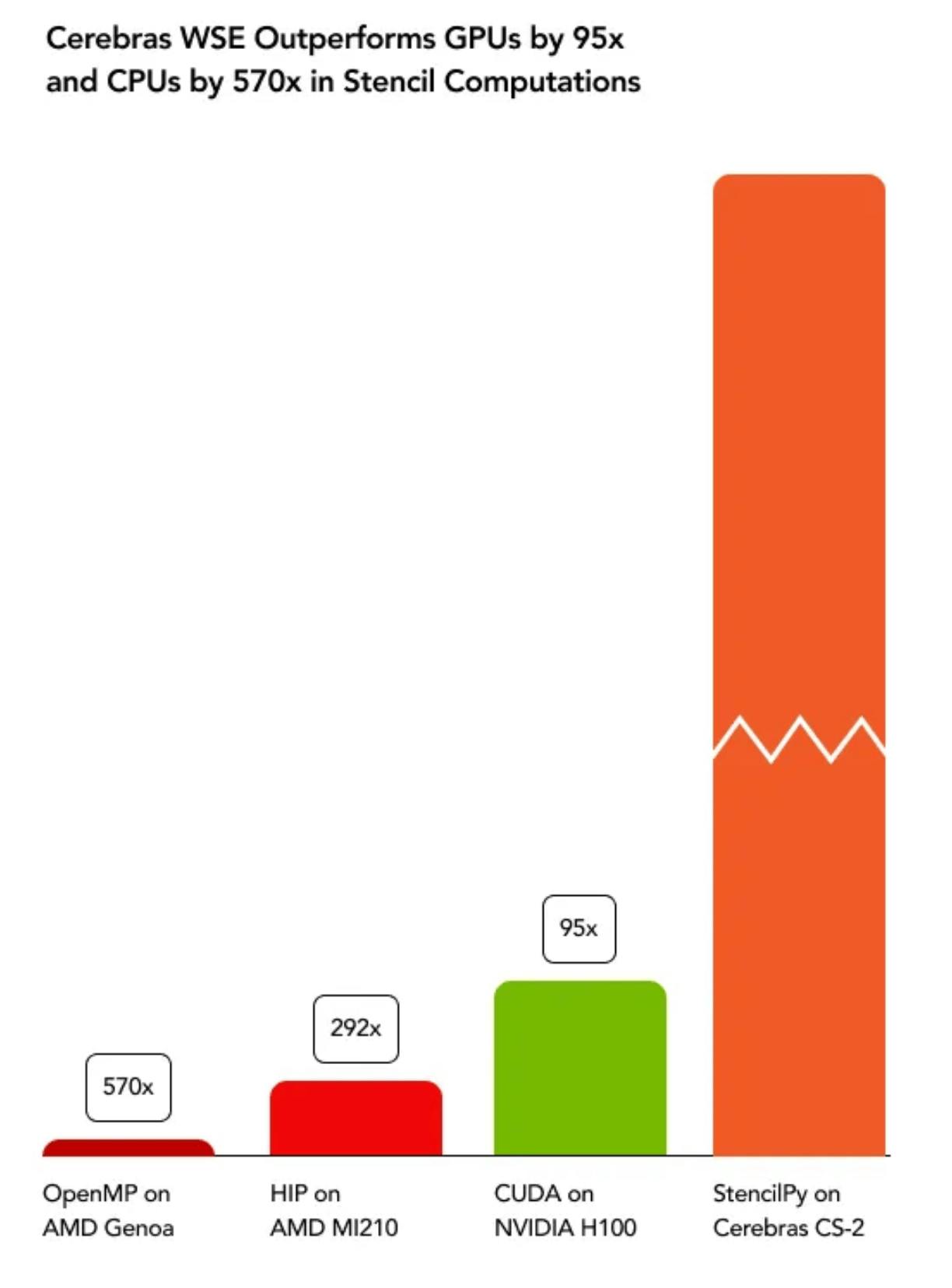
Source: Cerebras
Following the release of the GB200 chip at Nvidia’s 2024 ComputeX keynote, Cerebras demonstrated that its WSE-3 chip offers four times greater compute while being over two times more energy efficient. As a result, Cerebras can offer 10 times better compute per dollar and 3.6 times better compute performance per watt compared to currently deployed AI training platforms.
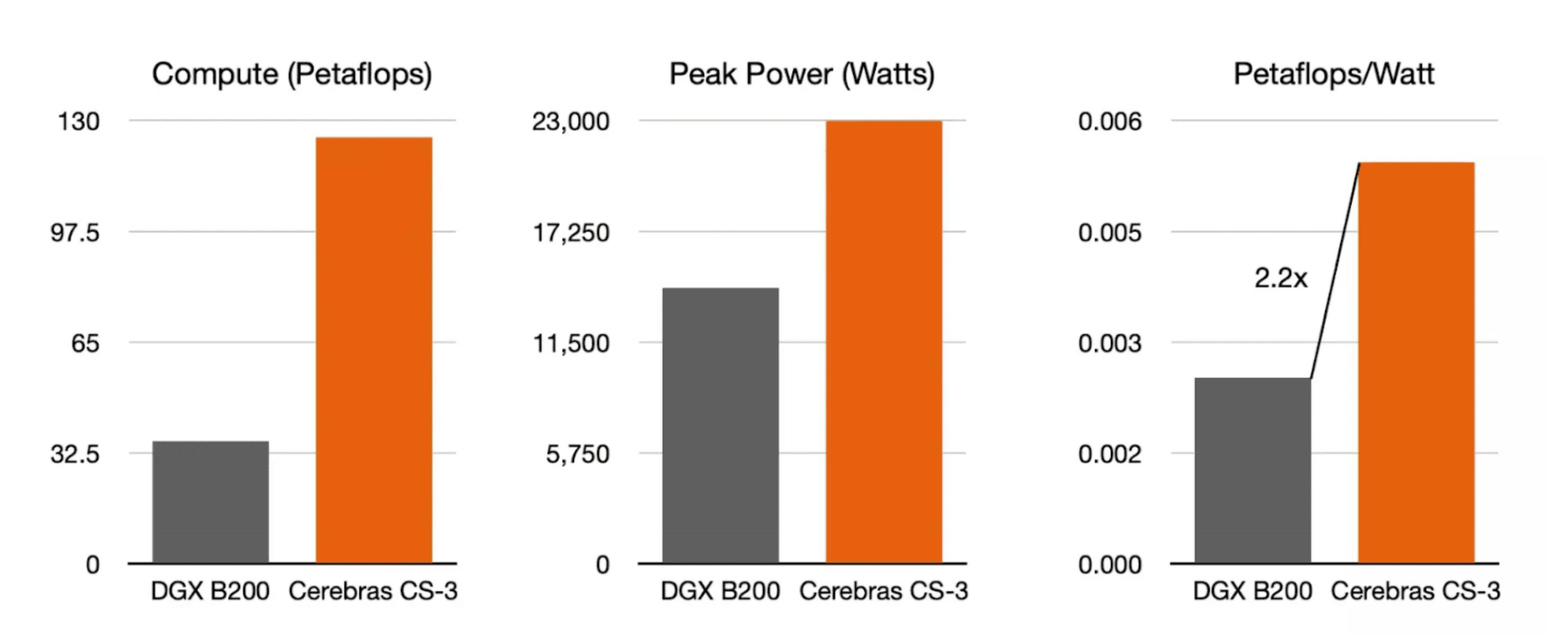
Source: Cerebras
In August 2024, Cerebras enabled inference capabilities on its CS-3 systems, becoming the world’s fastest AI inference provider. Just a month later, in September 2024, both Groq and SambaNova made strides in faster and faster inference before Cerebras reclaimed the title. Suffice it to say, it's a rapidly evolving race.
In an independent analysis by Artificial Analysis, Cerebras is able to deliver 1.8K tokens per second for Llama3.1 8B at ten cents per million tokens and 450 tokens per second for Llama3.1 70B at 60 cents per million tokens. According to Cerebras, this means its inference capabilities are 20 times faster for a fifth of the price compared to inference capabilities on Nvidia H100s. These lower latency inference capabilities will be important for AI developers creating products with real-time or high-volume inference requirements.
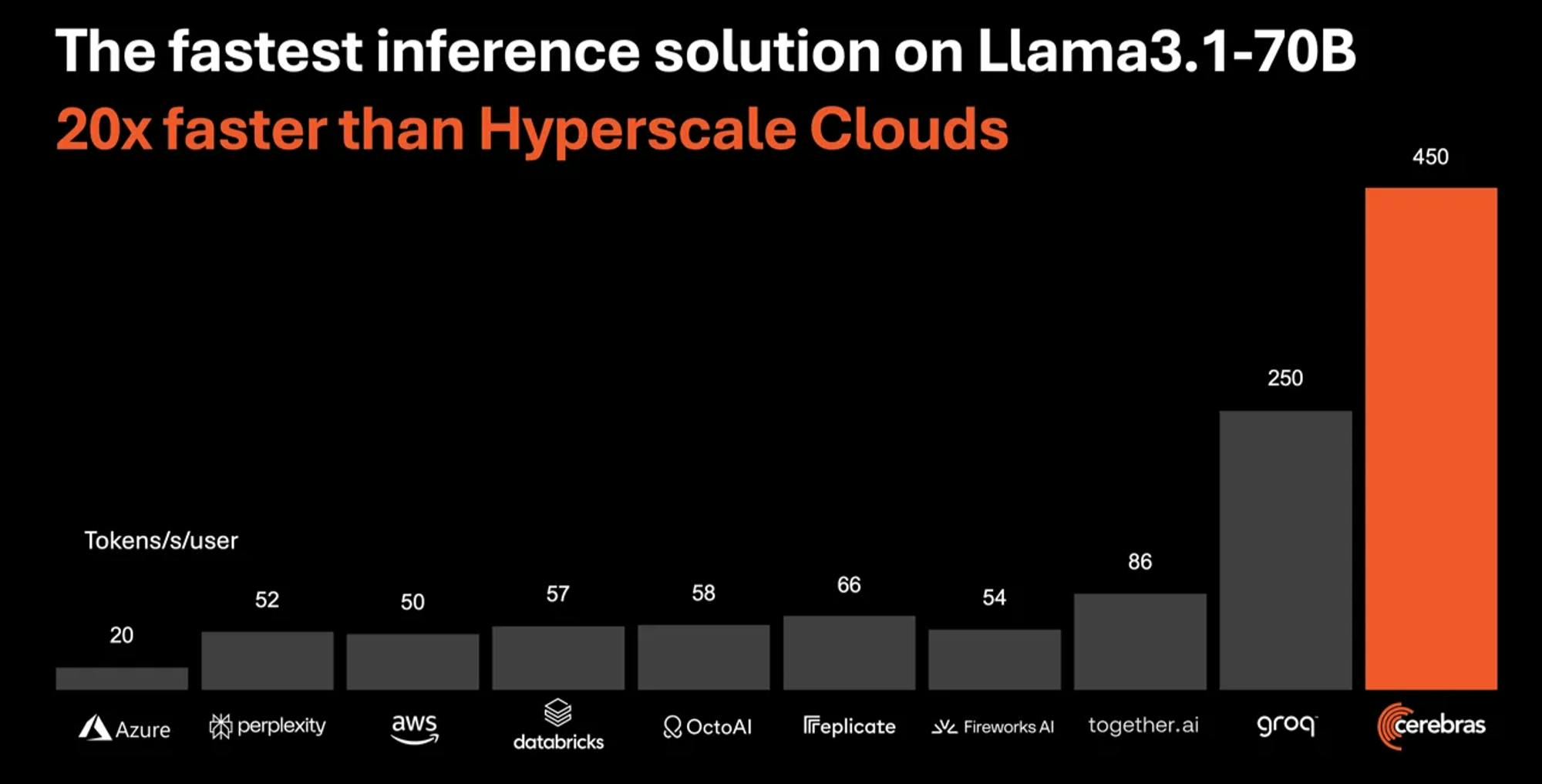
Source: Cerebras
According to Feldman, inference is slow largely because LLMs generate one token at a time. Each generated token must be processed through the entire model, meaning that all model parameters must be moved from memory to computation, and back to memory again. As each generated token is dependent on the previous token, the transfer of model parameters from memory to computation cannot be run in parallel. Therefore, generating 100 tokens per second requires moving the model 100 times per second, which requires a vast amount of memory bandwidth.
Because Cerebras tightly couples its memory cores with its compute cores, and the fact that it is the only AI chip in the world that has both petabyte-scale compute and petabyte-scale memory bandwidth, it is able to handle the high volume of memory transfer. In the future, if models do exceed the memory capacity of a single WSE-3 wafer, Cerebras is able to split the model up and distribute them across multiple CS-3 systems.
Through the Cerebras Inference API, developers can start building with Cerebras Inference. According to Cerebras, it is fully compatible with the OpenAI Chat Completions API, making “migration seamless from OpenAI to Cerebras Inference with just a few lines of code.”
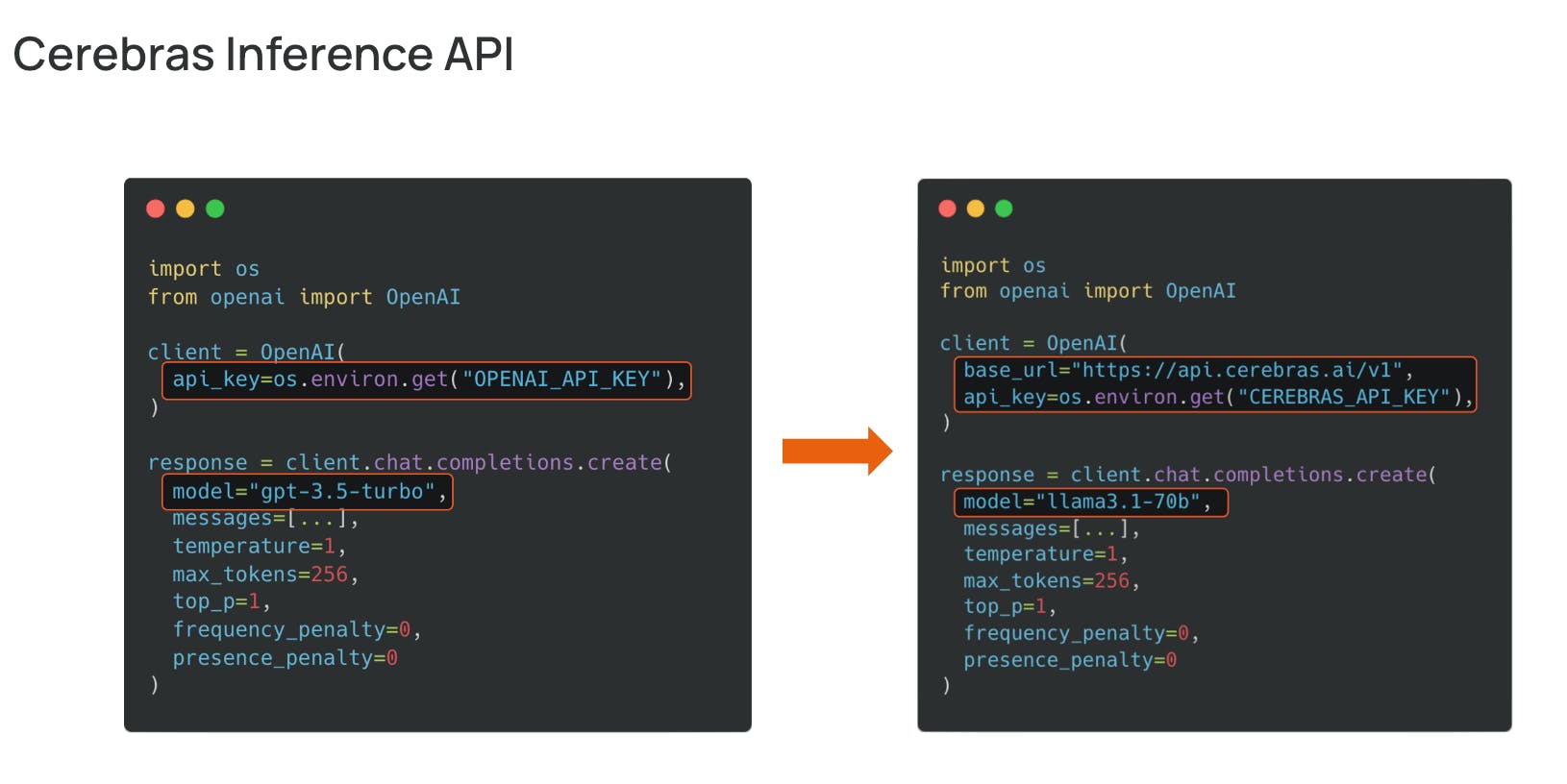
Source: Cerebras
Cerebras also offers the cheapest inference speed-to-price ratio to any provider as of September 2024. Compared to Groq, the formerly fastest AI inference provider in the world, Cerebras offers nearly double the output speed for roughly the same price. Compared to larger model inference providers like Amazon or Azure, Cerebras is up to 39% and 83% cheaper per million tokens, respectively.
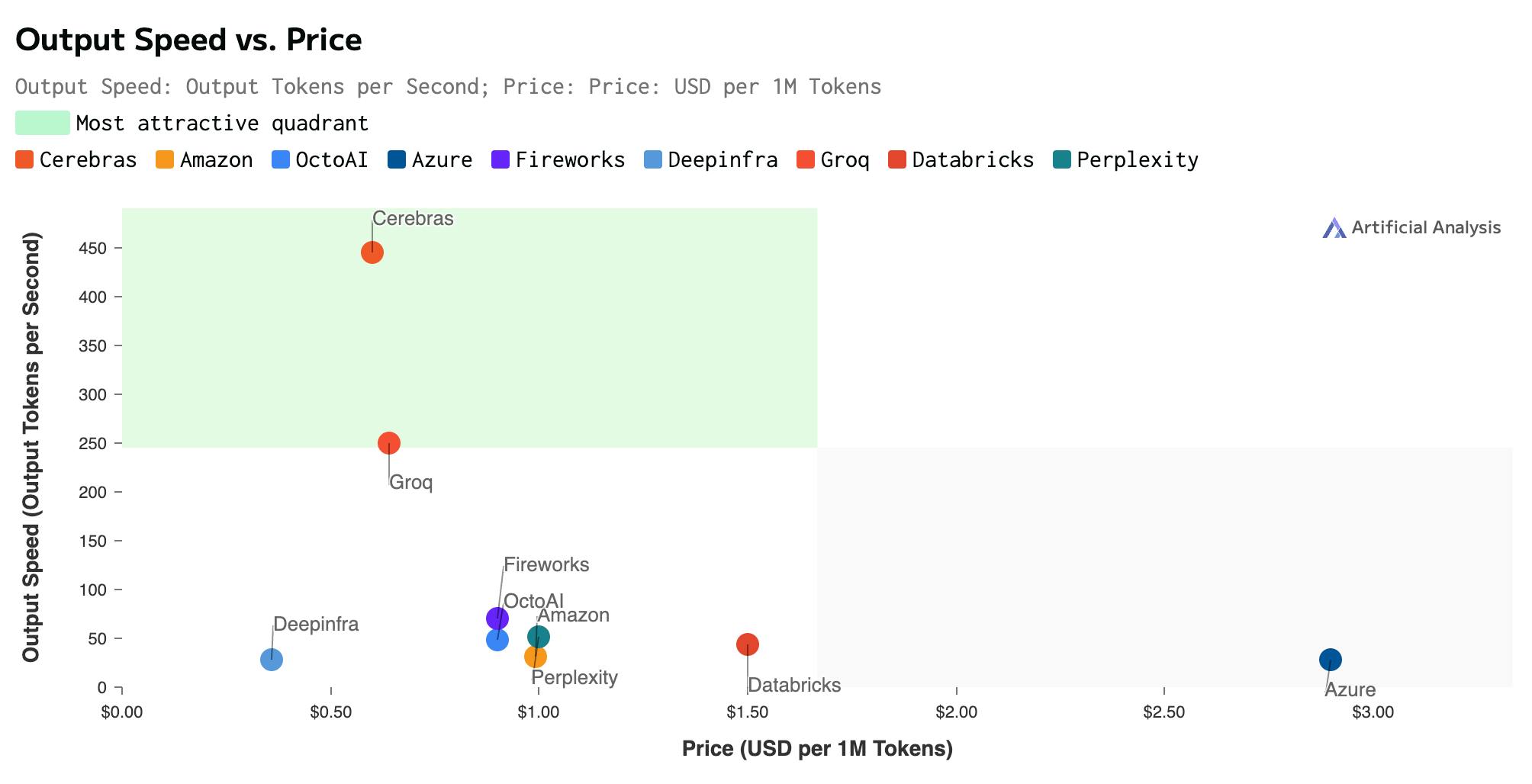
Source: Artificial Analysis
As a result, using Cerebras Inference, chat queries are near-instantly generated and completed, unlike any other provider on the market.
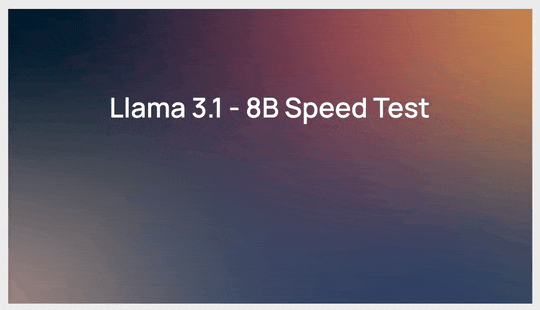
Source: Cerebras
Cerebras designed its computing servers to be compatible with industry standards. The CS-3 can be retrofitted into standard data center infrastructure and communicates using industry-standard protocols. The CS-3s can also take advantage of industry-standard machine learning frameworks like TensorFlow and PyTorch, allowing developers to use familiar programming languages. In the background, Cerebras’ graph compiler software automatically converts the TensorFlow or PyTorch code into optimized executables for its computing system.
Market
Customer
Cerebras’ computing systems typically cost upwards of $1.5 million per system, which is why the company aims to target sovereign cloud customers worldwide in any industry vertical that seeks to develop the “largest and hardest” AI models. Notably, pharmaceuticals, government research labs, and military agencies are willing to spend millions to host Cerebras’ compute clusters entirely on-site to keep their sensitive or private data local.
Although Cerebras does not disclose all of its customers publicly, it has spotlighted some of its notable customers, including:
Pharmaceutical companies: GlaxoSmithKline, Mayo Clinic, AstraZeneca, Bayer, and Genentech.
Government research labs: Argonne National Laboratory, Lawrence Livermore National Laboratory, Leibniz Supercomputing Centre, Pittsburgh’s Supercomputing Center, University of Edinburgh’s EPCC Supercomputing Center, National Center for Supercomputing Applications, and National Energy Technology Laboratory.
Military agencies: DARPA and Aleph Alpha.
Large enterprises: Tokyo Electron Devices, G42, TotalEnergies, and some undisclosed algo-trading firms.
Cerebras has also begun expanding its customer base to include smaller organizations. In late 2022, Cerebras teamed up with Cirrascale, allowing companies to send their data, describe a use case, and have Cerebras train and fine-tune multiple billion-parameter models. In 2023, Cerebras partnered with G42 and Colovore to allow smaller organizations to rent CS-3s by the hour.
Market Size
Prior to the release of ChatGPT, McKinsey’s 2018 AI and Semiconductors report predicted that the global training and inference hardware market could grow to $5 billion by 2025. More recent research indicated that the AI hardware market size could be dramatically higher than that; upwards of $53 billion in 2023, growing at a CAGR of 26%. Both figures could potentially still be too low given spending expectations among US hyperscalers.
Consider the following domestic investments into AI data centers for 2024: Amazon is planning to spend $10 billion each year for the next 15 years, Meta has stated that further investment is needed and is projected to spend $37 billion, Google has hinted at almost $50 billion in capital spending, and Microsoft and OpenAI are working on plans for a $100 billion AI supercomputer called Stargate. Even non-hyperscalers have increased their spending on data center-related infrastructure. For example, Elon Musk’s X and xAI will spend $10 billion on additional Nvidia GPUs in 2024.
Most prominently, Nvidia highlighted a 409% annual growth in its data center revenues for fiscal 2024, reaching a record $17 billion in sales. Meanwhile, demand for AI chips in 2024 shows no signs of slowing as more companies seek to train and deploy AI models. However, a growing number of private ventures and software giants are joining traditional chip producers in aiming to capture a share of Nvidia’s market dominance.
In August 2024, Cerebras also expanded to the $15.8 billion inference market. Compared to AI training, AI inference constitutes 40% of the total AI hardware market and will likely dominate AI costs in the future.
Competition
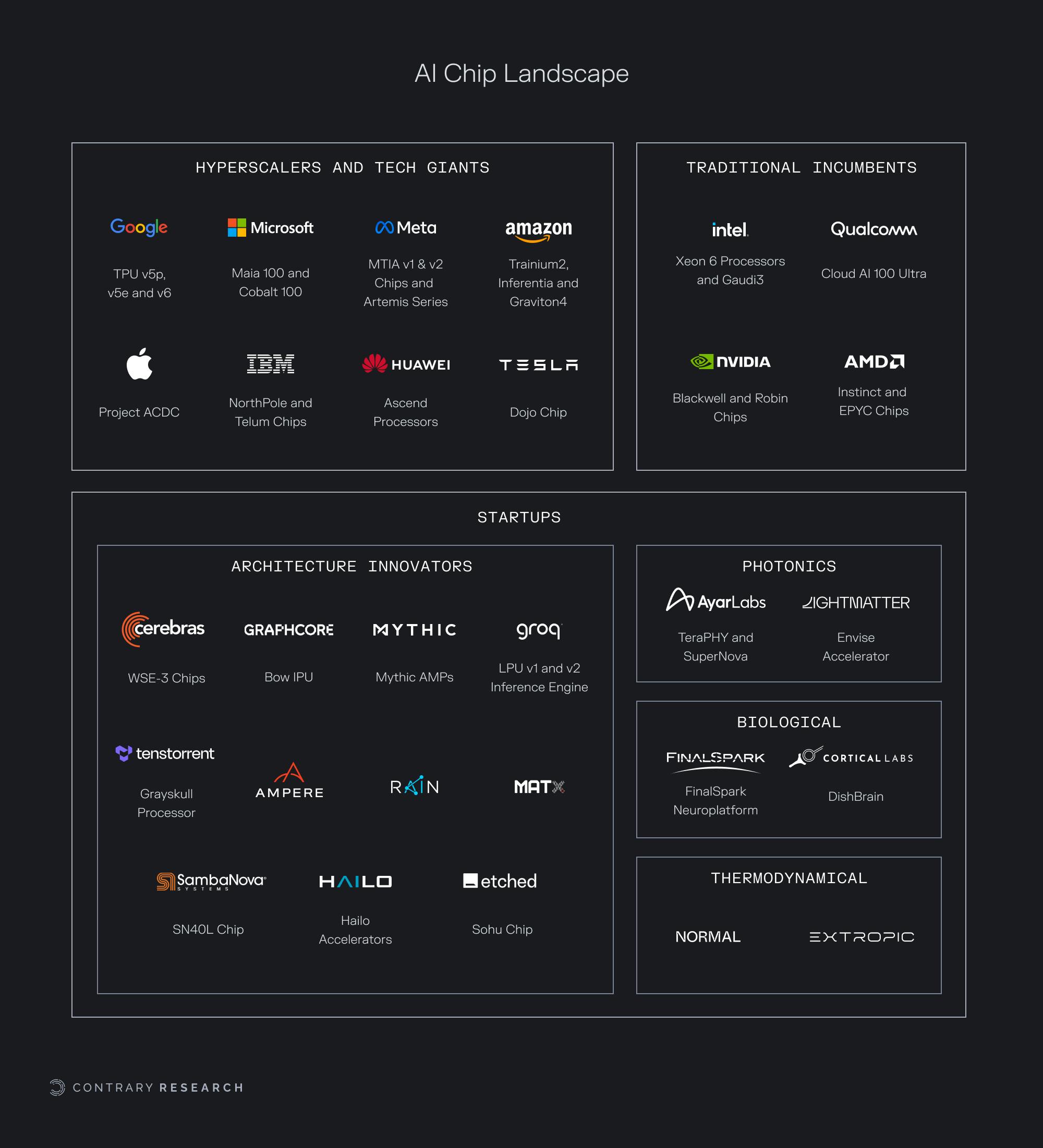
Source: Contrary Research
AI Training Competitors
SambaNova Systems: SambaNova Systems develops AI hardware chips with custom software as a full-stack enterprise solution. Like Cerebras, SambaNova Systems’ DataScale platform enables private companies, such as Accenture, to train their own AI models on proprietary data. Both companies have also released their own AI models, with SambaNova Systems releasing Samba-1, the first one trillion parameter enterprise model. Similar to Cerebras, SambaNova Systems is also capable of both training and inference. Running on its SN40L chip released in September 2023, which can run a five trillion parameter model, the Samba-1 model outputs 1K tokens per second. In comparison, GPT-4 running on clusters of 128 Nvidia A100 GPUs only outputs 21.4 tokens per second. Founded in 2017, SambaNova Systems had raised a total of $1.1 billion at a valuation of $5 billion as of September 2024, including a 2024 crowdfunding round that raised $1.5 million. In secondary markets, SambaNova’s valuation was estimated at $1.2 billion as of September 2024.
Graphcore: Graphcore custom processors, powered by its Intelligence Processing Unit (IPU) technology, enable 16 times faster AI training and inference speeds compared to Nvidia GPUs. Customers can train and deploy AI models using Graphcore’s Colossus MK1 IPU and Colossus MK2 IPU on the cloud or by purchasing its on-premise Bow Pod Systems for local development. Its customers include J.P. Morgan, Aleph Alpha, Carmot Capital, Microsoft, Citadel, and various universities, including the Imperial College London, Berkeley, Oxford, and Stanford. Graphcore was founded in 2016 and had raised a total of $682 million as of September 2024, being valued of $2.8 billion in 2020. However, Graphcore has struggled to gain further traction – reporting a 46% revenue drop from 2021 to 2022 and was in talks to be acquired by SoftBank as of May 2024.
Rain AI: Backed by Sam Altman of OpenAI, Rain AI manufactures brain-inspired “neuromorphic processing units” (NPUs) to optimize AI training and inference at maximum energy efficiency. Although not much is revealed about Rain AI’s NPUs, former Meta architecture leader Firoozshahian and Apple’s semiconductor leader Allegrucci joined the Rain AI team in June 2024. Rain AI was founded in 2017 and had raised over $40 million as of September 2024. Rain AI's energy-efficient chips may be attractive to companies prioritizing cost savings through reduced energy usage, potentially competing with Cerebras for customers who don't require the high-performance solutions Cerebras offers.
AI Inference Competitors
Ampere Computing: Ampere Computing develops energy-efficient CPUs for a wide range of computing solutions – including AI inference, web services, edge computation, and data analytics. Using a newly designed processor architecture, the Ampere Altra and higher-end AmpereOne CPUs use up to 67% less power for AI inference than a GPU and can be scaled linearly. Additionally, through a partnership with Nvidia, Ampere Computing offers full CUDA support when paired with Nvidia’s GPUs. Other notable partnerships include Oracle Cloud, Microsoft Azure, Google Cloud, Hewlett Packard Enterprise, Cloudflare, Cruise, and Tencent Cloud. Founded in 2017, Ampere Computing has raised $853 million and filed with the US Securities and Exchange Commission (SEC) for an original IPO date of April 2023. However, that has since been postponed and it remains a private company.
Tenstorrent: Founded in 2016, Tenstorrent develops AI cards designed to be plugged into traditional computers for accelerated AI inference, although the company has expressed interest in expanding to AI training. Whereas Cerebras offers large AI training solutions, Tenstorrent offers small-scale AI inference solutions. As of September 2024, Tenstorrent offers two AI Dev Cards: the Grayskull e75 for $599 and the Grayskull e150 for $799, which can be used by the average developer’s computer to accelerate inference speeds for local LLM models. Tenstorrent also sells two Networked AI Cards for commercial use: the Wormhole n150 and n300, which can be scaled to create Tenstorrent’s Galaxy systems. As of September 2024, Tenstorrent had raised a total of $334.5 million in funding, after raising $300 million in June 2024.
Groq: Groq was founded in 2016 by Jonathan Ross, who led Google’s Tensor Processing Unit (TPU) chip development. Through its cloud platform, GroqCloud, users can take advantage of Groq’s custom-designed “language-processing units” (LPUs) chips for rapid AI inference for cheaper. For example, Groq runs Meta’s Llama 3 model at roughly 350 tokens per second – which is 20 times faster than Microsoft’s Azure data centers while being eight times cheaper. As Cerebras looks to expand into the larger AI inference market, it will have to innovate against Groq’s inference-specialized LPU chips. As of September 2024, Groq had raised $1 billion in total funding.
Hailo: Founded in 2017, Hailo specializes in designing edge AI inference processors. While Cerebras builds large AI chips at a data center scale, Hailo builds processors that fit onto smaller smart machines and devices, such as the Raspberry Pi 5. This specialization in edge AI could limit Cerebras’ reach into the edge AI market, while capturing a share of cloud AI compute by meeting the demand for decentralized, efficient processing solutions in retail systems, healthcare devices, or autonomous drones. Hailo has raised $343.9 million, including a $120 million Series C in April 2024.
EtchedAI: EtchedAI was founded in 2022 by a pair of Harvard dropouts to create inference chips that specialize in running transformer-based models. The transformer model, created by a team of Google researchers in 2017, has dominated recent generative AI models, including GPT-4, Sora, Claude, Gemini, and Stable Diffusion. In June 2024, EtchedAI released its first Sohu chip, while claiming that it “is an order of magnitude faster and cheaper than even Nvidia’s next-generation Blackwell (B200) GPUs” and is 20 times faster than Nvidia’s H100 chips. Like Groq, EtchedAI will force Cerebras to innovate against the Sohu chip as Cerebras looks to expand into the inference market. As of September 2024, EtchedAI had raised $125.4 million in funding, including a $120 million Series A in June 2024.
Alternative Computing Frameworks
Lightmatter: Lightmatter was founded in 2017 to develop optical processing chips. Using lasers, Lightmatter has developed an efficient platform to accelerate compute. With its Passage interconnect, Lightmatter enables data centers a cheaper and easier way to connect multiple GPUs used in AI processes while reducing the bottleneck between connected GPUs. Lightmatter also developed its Envise AI accelerator chip using its photonics technology. The company raised a $155 million Series C in December 2023, and, as of September 2024, Lightmatter had raised a total of $422 million in funding to further develop its photonics microprocessors.
Ayar Labs: Ayar Labs was founded in 2015 to develop efficient optical solutions to transfer data between server chips. This is important as data transfer between chips is the main cause of power increases in server chips – so much so that Nvidia invested in Ayar Labs’ Series C round to “enhance the Nvidia platform.” It is likely that Nvidia seeks to leverage Ayar Labs’ optical interconnects for its GPU racks to improve energy efficiencies. As of September 2024, Ayar Labs had raised $219.7 million, including a $155 million Series C from notable strategic investors like GlobalFoundries, Intel Capital, and Nvidia in May 2023.
Cortical Labs: Unlike Lightmatter and Ayar Labs, which focus on optical technologies, Cortical Labs focuses on biological technologies. Founded in 2019, Cortical Labs had raised $11 million as of September 2024 to advance its DishBrain computer, which uses living brain cells instead of silicon transistors to compute. Cortical Labs has trained its brain cell-powered computers to play Pong and aims to expand to AI computing tasks. Cortical Labs believes its biological systems are more suitable for AI computing tasks as its DishBrain computer is more efficient than deep-learning algorithms while demanding up to 10 times less energy to run.
Traditional Chip Incumbents
NVIDIA: Nvidia is a public company with a market cap of $2.6 trillion as of September 2024. Founded in 1993, Nvidia has become the face of the AI revolution, capturing an estimated 70% to 95% of the market share for AI chips and supplying AI compute for most of the leading AI companies, including Microsoft, Amazon, Meta, Google, OpenAI, and xAI. Nvidia competes with Cerebras’ CS-3 systems through its widely adopted GPU hardware systems, such as the recently announced B200 GPU and its exclusive CUDA software. Although Cerebras’ most recent CS-3 systems outperform Nvidia chips in terms of performance and simplicity of installation, most AI developers worldwide rely on Nvidia GPUs and CUDA software. The high switching costs make it challenging for Cerebras to convince developers and companies to transition to its higher-performing servers and abandon their existing CUDA code.
AMD: AMD is a public company with a market cap of $231 billion as of September 2024. Founded in 1969, AMD is the next leading contender against Nvidia for AI compute, although AMD is catching up to Nvidia’s GPU dominance. AMD’s MI300X GPUs are generally more performant than Nvidia’s H100 GPUs, while the upcoming MI325X and MI400 are expected to take on Nvidia’s H200 and the latest B100 GPUs. As AMD’s chips rival Nvidia’s, they present an alternative over Cerebras systems. Developers and companies familiar with the traditional GPU architecture and software ecosystems, such as AMD’s open-source ROCm software, may find it easier to switch from Nvidia to AMD compared to adopting Cerebras’ unique systems.
Intel: Although primarily focused on CPUs, Intel has begun to develop its own AI processors. Founded in 1968, Intel announced a new product in 2024; the neuromorphic Gaudi3 chip, which positions Intel to compete with Nvidia’s H100 and AMD’s MI300X chips. Intel claims the Gaudi3 is 50% faster than Nvidia’s H100 for training AI models. Additionally, under the CHIPS and Science Act, Intel is set to receive $20 billion to advance domestic semiconductor manufacturing, reducing overseas reliance on TSMC. Nvidia, AMD, and Cerebras all solely rely on the Taiwanese TSMC for fabrication.
Qualcomm: Founded in 1985, Qualcomm historically focused on developing edge technology – mobile, cameras, Wi-Fi, 5G, and IoT systems – but has expanded into the AI compute space. In November 2023, Qualcomm announced its Cloud AI 100 Ultra chips, optimized for AI inference tasks. Leveraging its history in designing energy-efficient chips for smartphones, Qualcomm’s new AI chip is designed to be energy-efficient. This allows the AI 100 Ultra to outperform Nvidia’s H100 at image classification and object detection in power efficiency. As Qualcomm continues to develop energy-efficient inference chips, it is poised to expand its presence in the edge-AI market, potentially preventing Cerebras from entering this space in the future.
IBM: IBM has a long history of developing AI solutions, notably with Deep Blue in 1997 (beating chess champion Garry Kasparov) and Watson in 2011 (defeating Jeopardy’s all-time champions). Founded in 1911 for traditional computing, IBM has recently focused on developing AI solutions with watsonx AI and watsonx data. In October 2023, IBM announced its NorthPole chip, which uses a neuromorphic architecture similar to Intel’s neuromorphic Gaudi3 chip, achieving better performance with lower energy consumption than other chips on the market, including Nvidia’s H100 GPUs. This unique chip design offers low-energy performance for AI tasks, whereas Cerebras systems suffer from high energy consumption, requiring an internal liquid cooling system. As data centers increasingly look for more energy-efficient chips to power AI services, IBM is positioned to compete with Cerebras for cloud AI market share.
Hyperscalers
AWS: Following Google Cloud and Microsoft Azure, AWS also announced its custom-designed Trainium2 and Graviton4 chips in November 2023. Based on released specifications, it is rumored that the Trainium2 chip offers twice the AI training performance of the Nvidia H100 while being 30% to 40% more cost-effective. Meanwhile, the AWS Graviton4 was designed for AI inference tasks (like AWS’ other inference chip, Inferentia), offering 30% better compute performance compared to its predecessor, the Graviton3 chip. As the leading AI cloud provider, AWS, along with Nvidia, represents one of Cerebras’ biggest competitors.
Microsoft Azure: In November 2023, Microsoft Azure also announced its plans to break away from its dependency on Nvidia with its Azure Maia 100 and Azure Cobalt AI chips. The Azure Maia 100 was designed to run cloud AI workloads, including both AI training and inference. With Microsoft Azure 23% share of the AI cloud market, Microsoft Azure’s custom chips will also become a notable competitor to Cerebras’ cloud services.
Google Cloud: Although Google Cloud previously relied on Nvidia for its internal projects, it began developing its own Tensor Processing Units (TPUs) in 2015 for internal, and later, cloud use. Google Cloud TPUs are optimized for training and inference of AI models and designed to scale cost-efficiently. The latest TPUs – the v5p and v5e – are now available for cloud use, offering cheaper prices than other cloud providers and rumored to be on par with or even superior to Nvidia’s H100 GPUs. In May 2024, Google Cloud announced Trillium, the sixth generation of Google TPUs, which offers nearly five times better computing performance compared to the TPU v5e.
Meta: While Meta isn’t specifically a hyperscalers, in that it hasn’t started offering its own cloud computing services, Meta has developed its own AI inference accelerator. First released in May 2023, the MTIA v1 is now widely used for Meta’s internal workloads, with the second generation, MTIA v2, announced in April 2024. As Meta projects that its AI compute needs will grow over the next decade, the MTIA v1 and MTIA v2 are part of the company’s $40 billion capital expenditures to support AI processes.
Business Model
Cerebras operates on a hybrid model, offering both hardware sales and cloud services. The company generates revenue through three main channels: direct sales of CS-3 systems, supercomputing packages, and cloud-based services.
Cerebras targets sovereign cloud customers and large companies with its direct sales and supercomputing packages, while smaller companies and individual developers are the focus of its cloud-based services. This diversified approach allows Cerebras to cater to customers with varying needs and resources.
Direct Sales of CS-3 Systems
While Cerebras doesn’t sell its WSE chips as standalone components, it does sell entire units of the CS-3 system for on-premise deployment. This model is particularly attractive to customers with secure data requirements, such as military or pharmaceutical companies. On-premise deployment keeps sensitive data within the customer's controlled environment, reducing data breach risks that could occur in shared cloud environments, and allowing these organizations to comply with regulatory requirements. It also provides full control over AI infrastructure, which is important for handling classified or proprietary data.
While the exact price of the CS-3 is not publicly disclosed, it is reported to be priced similarly to its predecessor, the CS-2, which costs approximately $2 million or more per unit.
Supercomputing Packages
Cerebras offers packages of multiple CS-3 systems combined for high-performance computing needs, primarily targeting sovereign cloud providers and large government entities due to the substantial investment required. For example, the company's Condor Galaxy 1 system, comprising 64 CS-2 units, was priced at $100 million. Additionally, Cerebras plans to deploy nine more AI supercomputers for UAE-based G42.
Cloud-based Services
For customers with less intensive AI needs, Cerebras provides cloud-based access to its systems through a "Pay by the Hour" model on its Andromeda supercomputer, located in a Colovore colocation facility. This service offers flexible pricing based on model size and training duration.
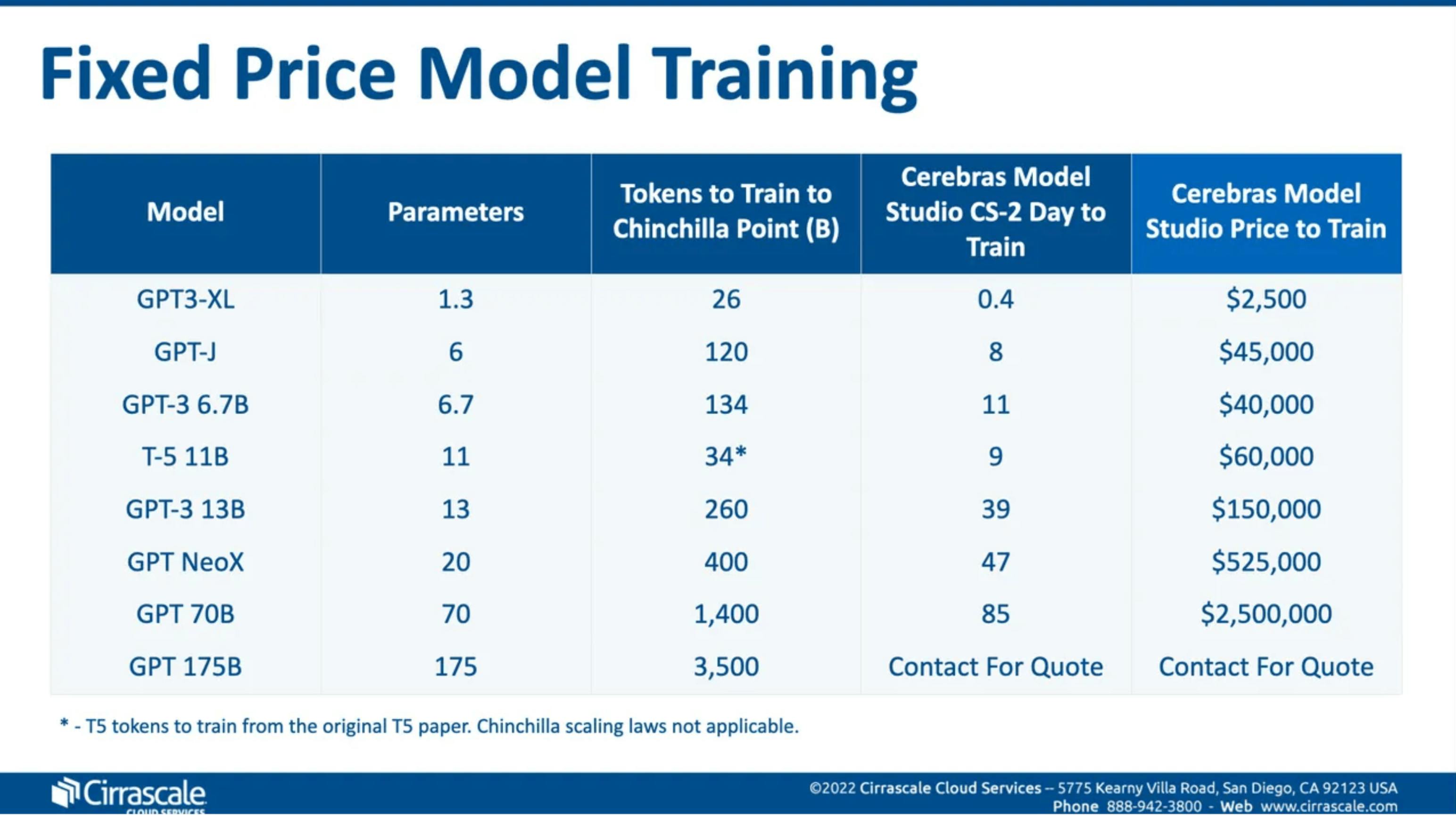
Source: Cirrascale
These prices are reported to be, on average, half the cost of renting equivalent cloud capacity or leasing machines for similar workloads. Additionally, the company claims its systems can train models up to eight times faster than clusters of Nvidia A100 machines in the cloud.
Cerebras also offers an AI Model Service as a "Pay by Model" option. Charging a flat fee, Cerebras will train models of a given size with a specified number of tokens for clients, competitively priced with AWS. Utilizing the company’s 50+ in-house developers and CS-3 systems, Cerebras delivers fine-tuned models within two weeks and pre-trained custom models within three months. These models can range from custom chatbots, code completion tools, summarization services, and classification systems.
Inference Service
For developers looking to build with Cerebras Inference through its developer API, there are three available pricing tiers, though the specific prices are not disclosed publicly:
The Free Tier offers free API access and specific usage limits to anyone.
The Enterprise Tier offers fine-tuned models and dedicated support for large enterprises with sustained workloads. Enterprises can access Cerebras Inference through a dedicated private cloud system managed by Cerebras or through on-premise systems.
Traction
In 2023, Cerebras reported a 10x increase in revenue, generating more than $250 million. This growth was matched by a 10x increase in manufacturing capacity for its chips, following a "massive" backlog of orders for CS-3s in 2023. As for 2024, Feldman has stated that “growth hasn’t slowed down” and, as of March 2024, estimated that Cerebras would deploy a zetaflop by the end of 2024. For comparison, the likely estimate for total worldwide GPU computing capacity in April 2023 was four zetaflops.
Supercomputing
Cerebras has already built two supercomputers — Condor Galaxy 1 in July 2023 and Condor Galaxy 2 in November 2023 — in partnership with G42, each valued at approximately $100 million. A deal is in place to build seven more with G42, including Condor Galaxy 3 coming online within 2024, for an additional $900 million. Within nine months, the deployment of three supercomputer clusters represents a significant portion of the revenue generated by all AI hardware startups to date. Cerebras also partnered with Nautilius in late 2023 to house approximately 80 CS-3s, providing enough compute power to be classified as a small supercomputer.
US National Labs Research
In February 2023, Cerebras’ CS-3s simulated real-time computational fluid dynamics 470 times faster than other supercomputers. In May 2024, with the Sandia National Laboratories, Lawrence Livermore National Laboratory, and Los Alamos National Laboratory, a Cerebras CS-2 accelerated a Molecular Dynamics simulation to be 179 times faster than the Frontier Supercomputer, the world's #1 ranked supercomputer. According to various principal investigators from the National Laboratories:
“The Advanced Memory Technology program started 1.5 years ago with the goal to accelerate exascale supercomputers by 40x. We all had our doubts on achieving this goal within the short timeframe, but Cerebras’s technology and team have helped us exceed this goal by demonstrating unprecedented 179x performance improvement on MD simulations. With Cerebras’ currently deployed wafer-scale computers, the teams achieved these materials science breakthrough and a speedup that exceeded the goal by more than 4x. Simulations that would have taken a year on a traditional supercomputer can now be completed in just two days. Scientists will now be able to explore previously inaccessible phenomena across a wide range of domains.”
In August 2023 at the Argonne National Laboratory, Cerebras' systems were found to be 300 times faster than existing compute infrastructure for cancer research. In a different project, the lab was able to uniquely model COVID-19 in a way that Feldman stated “had never been modeled before.”
Healthcare & Pharmaceuticals
In May 2021, AstraZeneca reported completing work in days that previously took weeks due to a collaboration with Cerebras. Meanwhile, in January 2022, GSK used Cerebras' CS-1 system to train an AI model for genetic data 160 times faster than traditional compute infrastructures. According to GSK:
“The training speedup afforded by the Cerebras system enabled us to explore architecture variations, tokenization schemes, and hyperparameter settings in a way that would have been prohibitively time and resource-intensive on a typical GPU cluster.”
In January 2024, Cerebras collaborated with Mayo Clinic to develop new LLMs for improving patient outcomes and diagnoses. Using Cerebras’ compute infrastructure and Mayo Clinic’s longitudinal data repository in healthcare, the companies aim to deliver a Rheumatoid Arthritis diagnostic model, which will combine data from patient records, DNA, and drug molecules to help match patients with Rheumatoid Arthritis to the best therapeutics to manage their disease. Mayo and Cerebras also plan to develop a similar model for pancreatic cancer, the fourth leading cause of cancer death in both men and women.
Military & Government
In May 2024, Cerebras secured a multi-year partnership with Aleph Alpha, a leading European AI firm, to develop sovereign AI models for the German Armed Forces. This marks the first installation of Cerebras’ CS-3s in a European data center.
Technology Partnerships
In June 2024, Cerebras collaborated with Dell Technologies and AMD to advance Cerebras’ memory storage solution in its supercomputers, extending its supercomputers to train models of near-infinite size. The collaboration also opens new global sales distribution channels for Cerebras.
Cerebras is also expanding its reach beyond AI training. In March 2024, the company partnered with Qualcomm to optimize its Cloud AI 100 accelerator. This enables Qualcomm to deliver up to 10x acceleration in LLM performance for inference tasks.
Other Industries
In November 2022, Cerebras partnered with Jasper, allowing Jasper to use Cerebras’ Andromeda supercomputer to train its computationally intensive generative AI models in a fraction of the time. In the same year, TotalEnergies adopted Cerebras' technology, integrating CS-2s into its research facility in Houston, Texas. This collaboration enabled TotalEnergies to advance its clean energy roadmap by achieving over 200 times speedup on tasks such as seismic wave propagation modeling. In March 2022, an undisclosed financial institution implemented Cerebras' systems and reported a 15x reduction in training time for a financial AI model, with more than twice the accuracy and half the energy consumption of traditional GPU clusters.
Training LLMs
Using its Andromeda and Condor Galaxy supercomputers, Cerebras has trained and open-sourced seven state-of-the-art LLM models. These include what the company claims to be “the world's leading bilingual Arabic models” Jais-70B, Jais-30B, and Jais-13B; BTLM-3B-8K, the top-performing 3B model on Hugging Face as of July 2024, offering 7B parameter performance in a compact 3B parameter model; MediSwift, a biomedical LLM that employs sparse pre-training techniques to reduce compute costs by half while outperforming existing 7B parameter models; and Med42, a leading clinical LLM developed in a single weekend that surpasses Google’s Med-PaLM in performance and accuracy.
Notably, Cerebras claims to have trained these models with significant efficiency, assigning only a single engineer to the task compared to the 35 engineers OpenAI reportedly used for distributed model training across GPU infrastructure. Some of these models have gained substantial traction, with download counts exceeding 400K.
Inference Service
Although Cerebras has focused primarily on training large AI models with billions of parameters, in August 2024, Cerebras announced that it now provides the world’s fastest inference capabilities as well. Since its release, several influential individuals in AI have praised Cerebras inference capabilities. Founder of DeepLearning.AI, Dr. Andrew Ng, stated that Cerebras has built an impressively fast inference capability. Denis Yarats, CTO and co-founder of Perplexity, also said that the lower latencies and ultra-fast inference speed by Cerebras will be “big for user engagement and unlock better user interaction.”
Valuation
In November 2021, Cerebras raised a $250 million Series F at a $4 billion valuation, bringing the company’s total funding amount to over $720 million. The Series F round was led by Alpha Wave Ventures, Abu Dhabi Growth Fund, and G42, joining existing investors such as Eclipse Ventures, Sequoia, Altimeter Capital, Benchmark Capital, and Coatue Management. This round followed Cerebras’ Series E raise of $272 million in November 2019, where it had been valued at $2.4 billion.
As of September 2024, Cerebras valuation was estimated at $4.7 billion in secondary markets. For comparison, other AI chip startups have achieved similar valuations: Graphcore was valued at $2.8 billion, Lightmatter at $1.2 billion, Groq at $1.1 billion, and Tenstorrent at $1 billion. While SambaNova Systems was valued at $5 billion in April 2021, the company’s valuation has since come down in secondary markets to an estimated $1.2 billion as of September 2024.
Cerebras has also attracted investment from prominent individual investors in the tech industry, including Sam Altman (CEO and Founder of OpenAI), Ilya Sutskever (Founder of OpenAI and Founder of Safe Superintelligence), Andy Bechtolsheim (Founder of Sun Microsystems), Saiyed Atiq Raza (Former COO of AMD), Fred Weber (Former CTO of AMD), Greg Brockman (Former CTO of Stripe), Adam D'Angelo (Former CTO of Facebook and CEO of Quora), Pradeep Sindhu (Founder and Former CEO of Juniper Networks), Jeff Rothschild (Former VP of Engineering of Facebook), and Lip-Bu Tan (Former CEO of Cadence).
In 2023, Cerebras was estimated to be generating more than $250 million in revenue. The company announced in December 2023 that the combination of the company’s revenue and overall customer commitments represented a number approaching $1 billion, with revenue doubling year-over-year. It also reported reaching cash flow break-even and announced that it picked Citigroup as the lead bank for a Cerebras IPO in April 2024. This has sparked interest among investors in purchasing Cerebras stock once it becomes publicly available, as evidenced by interest in Cerebras secondaries on platforms like Caplight and Hiive.
In August 2024, Cerebras pushed ahead by submitting a confidential submission for a proposed IPO. Based on Cerebras’ estimated $4.7 billion valuation in secondary markets, the implied revenue multiple is approximately 4.7x. In comparison, public companies in the AI semiconductor space as of September 2024 show a range of revenue multiples from 2-27x.
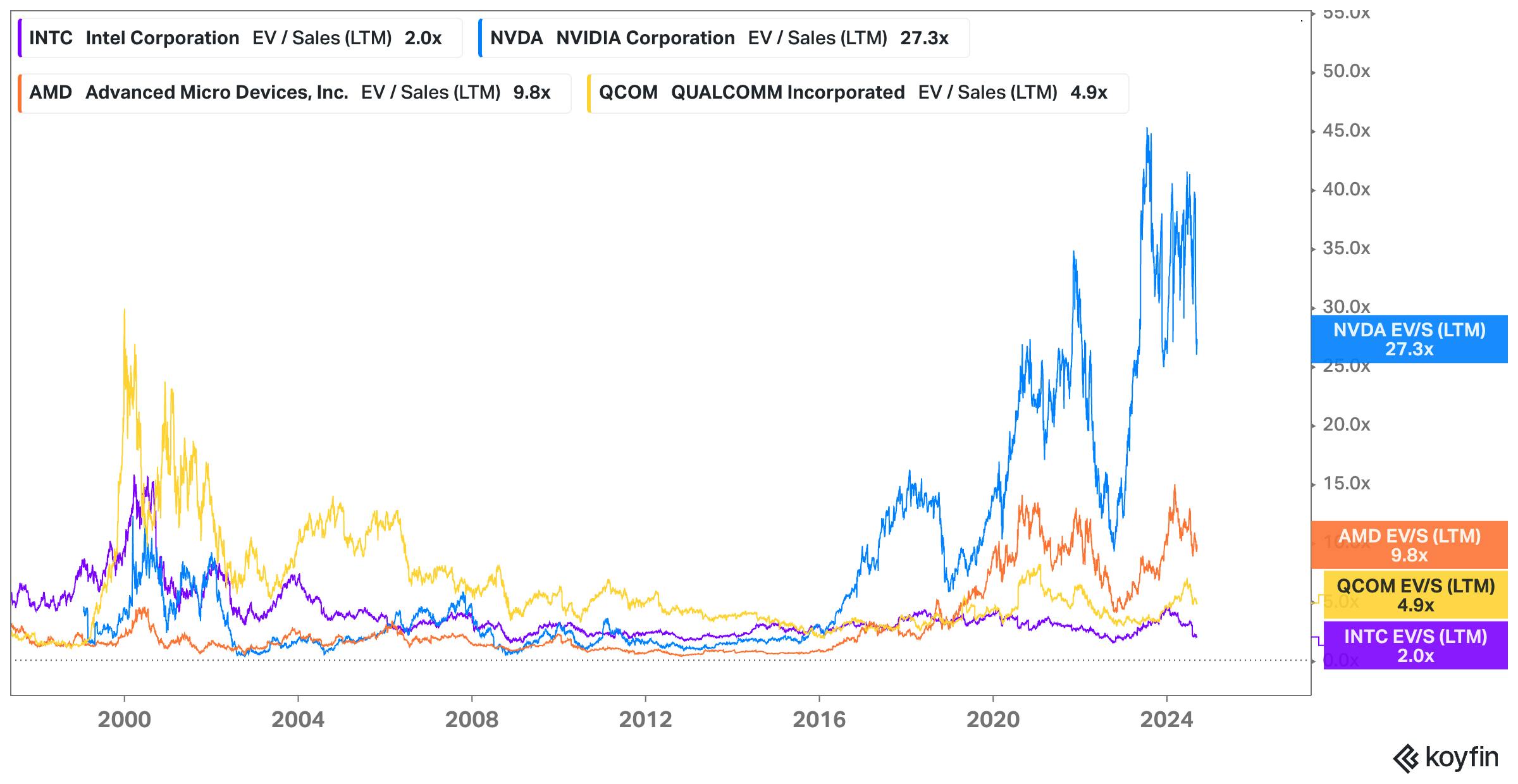
Source: Koyfin
Key Opportunities
Optimizing Model Inference for Inference-Specific Chips
During the early adoption of AI, companies focused on training their own models. However, as the industry evolves, the bigger opportunity lies in AI inference, which involves running models indefinitely after they have been trained. Colette Kress, the CFO of Nvidia, has stated that 40% of Nvidia’s data center revenue now comes from inference rather than training, indicating a significant shift towards AI inference. Likewise, OpenAI spends more on inference ($4 billion) than on buying data and training models combined ($3 billion). Furthermore, McKinsey predicts that the opportunity for AI inference hardware is double that of AI training hardware.
Although Cerebras focuses primarily on AI training tasks, the company has begun to expand into the AI inference space without creating new chip technologies to account for the differences in training compute and inference compute. In May 2024, Cerebras partnered with Qualcomm to optimize an LLM for Qualcomm's AI100 Ultra chip, achieving 10x better performance per dollar.
By proving the capability to optimize models for specific hardware chips, Cerebras will be able to:
Expand partnerships with other AI inference chipmakers to optimize models for their chips.
Diversify revenue by offering model optimization services for smaller AI companies.
Attract customers concerned with end-to-end AI workflow efficiency in training and inference.
If Cerebras replicates this success with other hardware partners, it could become a key player in connecting AI training and inference, potentially improving AI deployment efficiency industry-wide.
Pre-tuning Sparse Language Models for Faster AI Training & Inference
Emerging advancements in AI training have focused on developing sparse language models. Sparse language models are neural networks pruned of unimportant connections, reducing model size while maintaining accuracy. This makes inference more cost-efficient.
Unlike traditional hardware built for unpruned and "dense" LLMs, Cerebras’ unique SLAC cores comprise the only platform that supports native hardware acceleration for sparse language models, speeding up training by up to eight times. As of March 2024, this wasn’t possible in other chips.
Cerebras’ chips avoid unnecessary matrix multiplication calculations by skipping multiplications involving zero, unlike traditional GPUs. According to Feldman, the mathematical foundation of AI involves many zero multiplications, leading GPUs to waste time and power on these operations. In contrast, Cerebras' chip skips these multiplications, making it more efficient for sparse linear algebra problems common in AI.
Cerebras has demonstrated a level of superiority of sparse LLMs over dense LLMs:
On its MediSwift LLM model in March 2024, Cerebras pruned 75% of the connections in the neural network during pre-training, resulting in a 2.5x reduction in required training FLOPs with only a 1.4% accuracy drop compared to the dense MediSwift model.
In May 2024, Cerebras partnered with Neural Magic to demonstrate that sparse optimization of dense LLM models can be achieved without sacrificing model quality. By sparsifying a dense Llama-7B model, Cerebras achieved 8.6x better inference performance, and thus inference costs, without sacrificing model quality.
In light of these advancements, Cerebras may be able to create a new market in AI model development. As seemingly no other company can offer the unique combination of hardware and software capabilities for efficient sparse model training at scale, Cerebras could emerge as the go-to solution for AI model developers looking to pre-train and sparsify their models before final training to reduce overall training costs. This approach could also allow companies to explore larger and more complex models with Cerebras while maintaining reasonable computational budgets.
Faster & Easier Model Training
The development of larger AI models presents an increasing challenge for AI developers, primarily due to the exponential complexities of distributed computing across numerous GPUs. Training GPT-1 required four contributors to implement the entire model, while GPT-4 required 35 dedicated solely to solving the distributed computing problem. As larger AI models are developed, the complexity of this task may become a bottleneck in AI research and development, limiting the ability of smaller teams or organizations to compete in the field of large-scale AI.
For example, Imbue encountered major difficulties when scaling AI training across over 4K H100 GPUs:
Out of the 511 racks of GPUs, 10% failed to boot due to unconnected or miswired Ethernet cables and missing internal wires.
Some racks had failing GPUs, requiring engineers to physically slide out the 200-pound server from the rack, remove all cables, and then reseat the GPUs before reconnecting the cables and reracking the server.
To ensure the health of all racks, Imbue had to develop software to perform checks on disk space, docker health, PSB health, and other metrics.
In another research paper released by Meta, there were significant challenges in using a cluster of over 16K H100 GPUs:
Of the 419 unexpected failures, 148 (30.1%) were due to H100 GPU issues, particularly with NVLink, and 72 (17.2%) were related to HBM3 memory failures. This is not surprising, given that Nvidia's H100 GPUs, consuming 700W each, endure significant thermal stress.
This means that there was an average of one failure every three hours, with GPUs being the root cause in nearly half of the cases. Given the scale and synchronous nature of clustered GPU training, a single GPU failure, if not mitigated, can disrupt and delay the entire training job. As clusters grow larger (for example, Meta plans to use over 350K H100s by 2025), this problem is likely to worsen.
By consolidating thousands of GPUs into a single computing system, Cerebras is able to eliminate many of these challenges, offering a more reliable and manageable training environment. This allows engineers to focus more on model architecture and application rather than on the intricacies of distributed computing, leading to fewer lines of code and code languages. According to Cerebras, a single engineer was able to train several LLMs in a matter of weeks compared to multiple months on standard GPU clusters. For many AI companies, this speed advantage could greatly accelerate innovation cycles and reduce time-to-market for new AI solutions, and thus be worth paying for.
As the demand for increasingly sophisticated AI models grows across industries, Cerebras is well-positioned to capitalize on this trend by offering a solution that addresses the key pain points in large-scale model training. Additionally, as regulatory and competitive pressures drive more companies to develop proprietary AI models in-house, Cerebras' on-premise offering becomes increasingly attractive, enabling organizations to easily set up and train large models while maintaining data privacy and intellectual property control.
Capitalizing on the Mainframe Resurgence
The emergence of AI has reignited interest in mainframe computing solutions, particularly among industries that require high-speed data processing and prefer in-house solutions over cloud services. Banks, telecommunication companies, healthcare organizations, and government agencies are among the sectors finding renewed value in secure, on-premises computing systems. This resurgence is primarily driven by speed requirements, high compute volumes, and data privacy concerns. For example, in banking, analyzing potentially fraudulent transactions must be accomplished in milliseconds, a task that cloud-based solutions often struggle to achieve due to latency issues. According to Stephen Dickens, VP of Futurum Group, a research and advisory firm, "One cannot go to the cloud, go through a search, and go through a generative AI query because it would just timeout."
Because Cerebras not only offers cloud solutions but also sells its CS-3 systems directly to companies, the company is capitalizing on the growing demand for localized mainframes — evident through IBM's reported 6% growth in its mainframe business in Q2 of 2024. Additionally, a 2023 survey found that 54% of existing mainframe customers plan to increase their usage over the next two years. As Cerebras’ mainframes offer greater scalability, are AI-optimized, and easier to deploy, Cerebras can position itself as a modern alternative to traditional mainframes, offering both the speed and security benefits of on-premises traditional mainframes with the compute power and flexibility found in cloud systems for AI workloads.
Key Risks
Breaking Nvidia’s CUDA Moat
While Cerebras' training chips outperform Nvidia in terms of raw performance, the company has no answer to Nvidia's established CUDA ecosystem, which has developed for over 15 years into a stable and reliable platform for AI development. This entrenched software and development environment presents a barrier to Cerebras' expansion in the AI hardware market despite its superior chip performance.
Most companies today already use Nvidia's GPU and CUDA stack, including some of the largest tech companies in the world: Microsoft, Amazon, Meta, Google, OpenAI, and xAI. Even if Cerebras convinces these companies to overcome the switching costs to adopt its higher-performing and easier-to-set-up solutions, it must contend with the reality that over 500 million GPUs run CUDA, and thousands of applications have been developed using Nvidia's closed-source CUDA ecosystem. This means there are many more examples, documentation, and resources for programming in CUDA, and finding AI engineers experienced with Nvidia’s ecosystem is easier. This abundance of CUDA-experienced engineers and the platform’s integration with major frameworks make it challenging for new entrants like Cerebras to gain traction.
However, Feldman argues that most AI work is done on open-source frameworks like TensorFlow and PyTorch, which has weakened Nvidia’s CUDA moat. Additionally, Feldman believes that developers have increasingly adopted PyTorch as the preferred framework for AI work, leading Cerebras to focus its efforts on being easily integrated with PyTorch. Other startups are also developing open-source solutions to replace CUDA. One such example is Modular, which allows CUDA-level performance for Nvidia GPUs by optimizing existing PyTorch models without forcing developers to write low-level CUDA code. Through its free MAX (”Modular Accelerated Xecution”) platform, Modular provides the opportunity for any developer to have their PyTorch models reach near-CUDA level performance. New programming languages, such as Mojo with over 175K developers, also improve upon Python (which PyTorch uses) performance without the need for complicated C++ or CUDA code.
Still, some tasks and libraries are tightly integrated with CUDA – such as CuPy, OpenCV CUDA, and DeepStream – which currently locks engineers into Nvidia's GPU platform. In other scenarios, CUDA-optimized code significantly outperforms optimized PyTorch implementations (although PyTorch 2.0 and future versions continue to close the gap between CUDA and PyTorch performance). Overcoming Nvidia's established ecosystem remains a hurdle for Cerebras' expansion given current market dynamics.
Crowded Market
Since 2015, the AI hardware market has seen continuous entrants. The landscape is populated by over 20 well-known competitors, each approaching the AI hardware market from different technological angles. This includes AI training competitors like SambaNova Systems and Graphcore, AI inference competitors such as Ampere Computing and Groq, companies exploring alternative computing frameworks like Lightmatter and Cortical Labs, traditional chip incumbents including Nvidia and AMD, and hyperscalers developing their own chips, such as AWS and Google Cloud.
The crowded market presents several interrelated risks for Cerebras. As new technologies emerge, such as improved silicon materials or novel architectures like neuromorphic chips, Cerebras faces fierce competition both now and in the future. Any company in this market risks losing its position as every other competitor races to provide faster training times. Already, Cerebras' main competitor, Nvidia, has improved AI compute by 1K times in just 8 years, narrowing the gap between its GPUs and Cerebras' WSEs.

Source: Nvidia
Some industry experts also suspect that it's only a matter of time before Nvidia applies similar scaling principles to those used in Cerebras' WSEs. In April 2024, Nvidia demonstrated the ability to combine two separate GPU dies into a larger single GPU in its latest Blackwell GPU, effectively creating “just one giant chip,” just as Cerebras has done.
However, in August 2024, Nvidia revealed that the numerous complexities involved in manufacturing the combined two GPU dies — including power delivery issues, overheating, water cooling leakages, and board complexity challenges — have led to months of shipment delays as Nvidia redesigns a part of the chip. In response, Cerebras released a video deconstructing Nvidia’s GB200 challenges and explaining how Cerebras has avoided this issue despite joining dozens of WSEs together onto a dinner-plate-sized system. As AI models grow in size and require more memory, the traditional chip design used by Nvidia will likely increase in complexity and manufacturing difficulty, challenges that Cerebras can avoid with its simplified manufacturing approach using WSEs. Even if Nvidia manages to solve these difficulties, Cerebras’ technology remains years ahead.
Finally, Nvidia’s Blackwell specifications may indicate that Nvidia may have achieved near-perfect scaling with its chips, potentially neutralizing one of Cerebras' key competitive advantages.
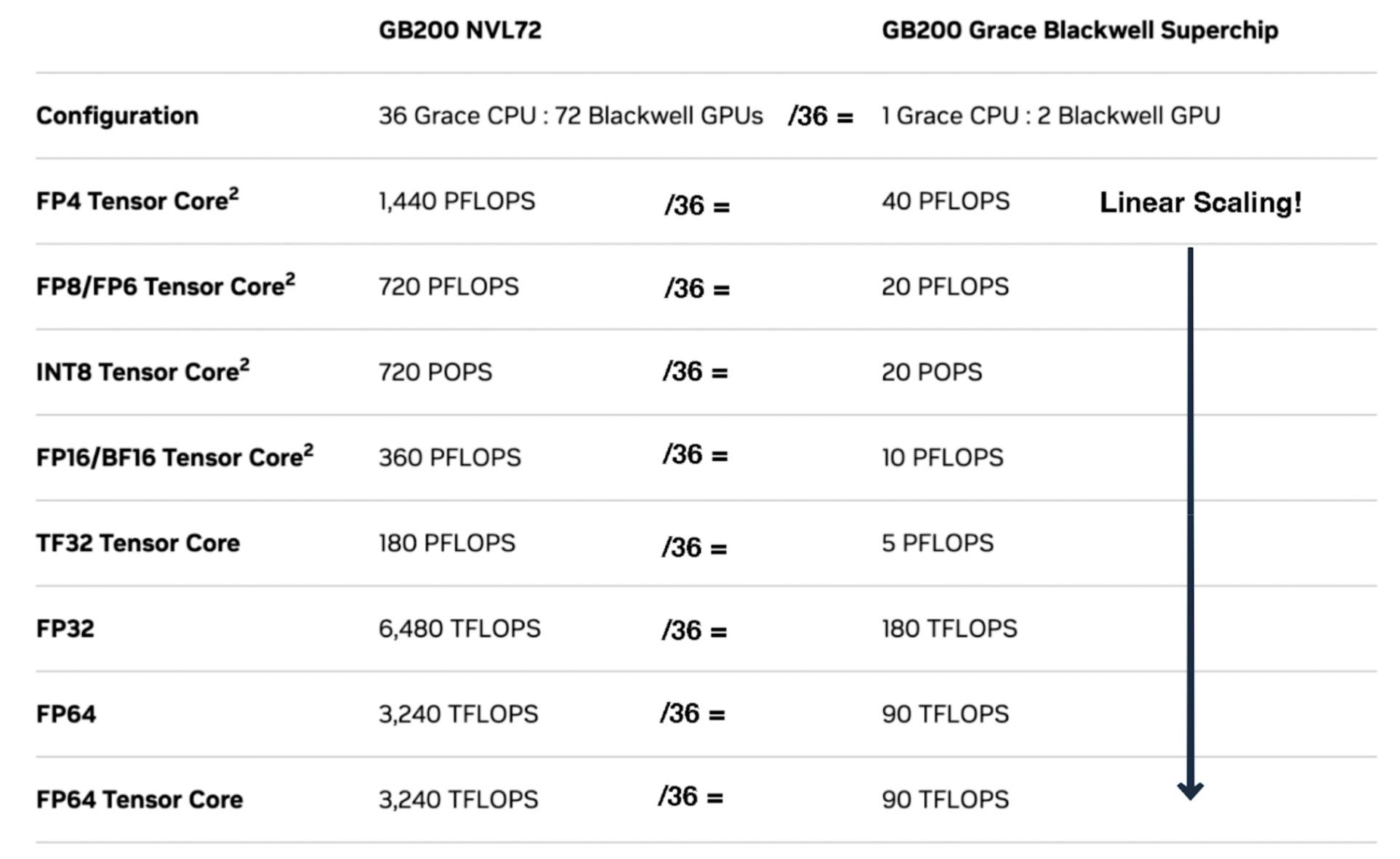
Source: Nvidia, Contrary Research
The fragmentation of the market, with numerous players offering diverse solutions for diverse customer needs, could lead to a scenario where no single approach dominates. This will make it challenging for Cerebras to achieve significant market share in the long term. Adoption barriers among competitor chips present another challenge. As established players like Nvidia, Google Cloud, and AWS continue to improve the price-to-performance of their services and chips, the switching costs for potential Cerebras customers will remain high. Convincing companies to abandon existing infrastructure and software ecosystems in favor of Cerebras' solutions may become increasingly difficult, especially if competitors can offer comparable performance improvements.
Challenges in Large Chip Manufacturing
All semiconductor chips begin with lithography machines that etch patterns onto a standardized 12-inch silicon wafer. The entire semiconductor industry relies on ASML’s Extreme Ultraviolet (EUV) lithography equipment for chip manufacturing, which is designed to work with the 12-inch wafer size. This standardization has set a physical limit on the maximum size of chips that can be produced using current manufacturing processes.
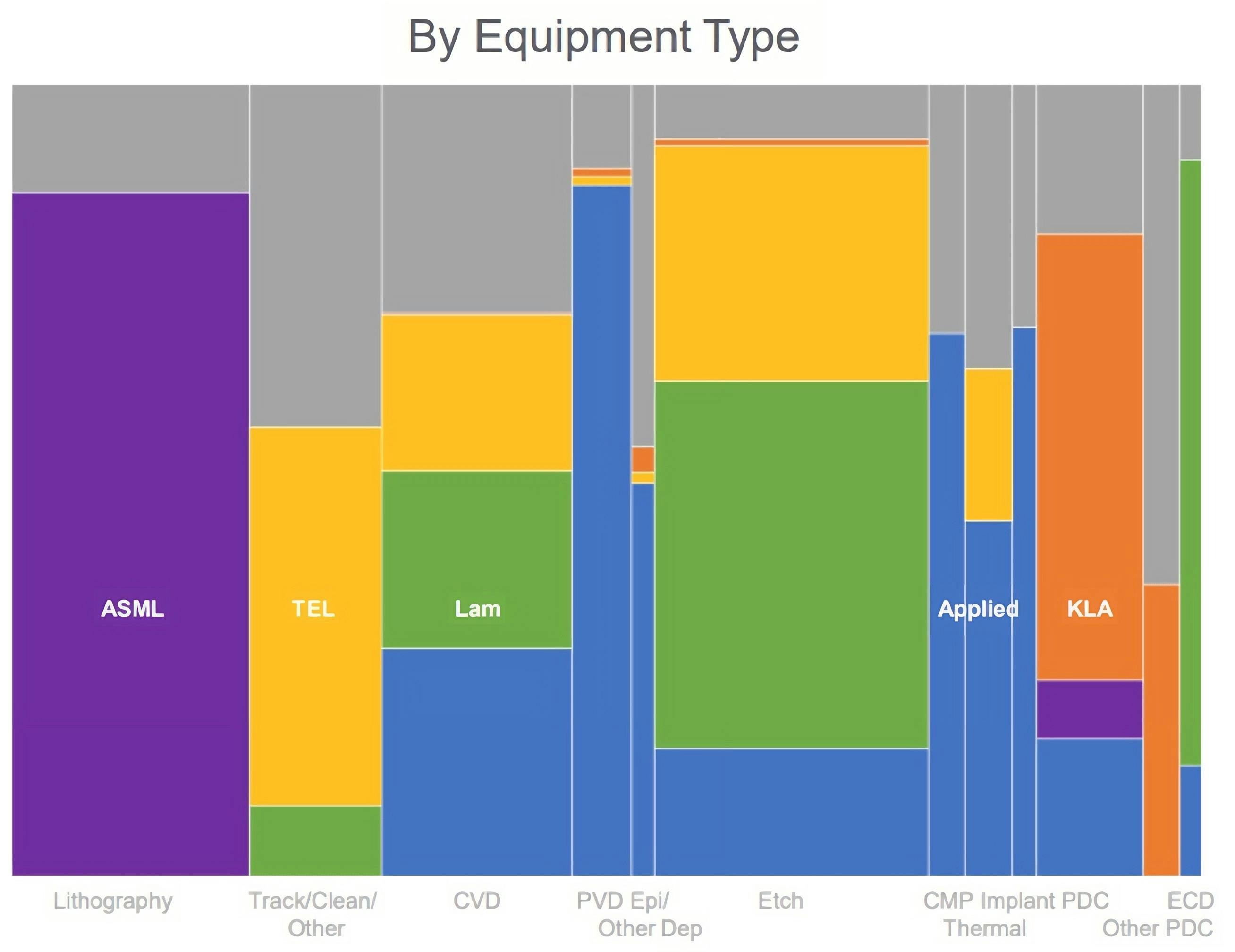
Source: Applied Materials
This presents the first problem for Cerebras: the company has reached the maximum possible chip size given the current limitations of ASML's EUV equipment. This means that future improvements will likely be incremental, focusing on making the existing chip design more efficient rather than increasing its size unless ASML creates new equipment to process larger silicon wafer sizes. However, ASML is unlikely to develop equipment for wafers larger than 12 inches in the foreseeable future. The transition to larger wafer sizes requires massive industry-wide investments and coordination, which has proven challenging as evidenced by the failed attempt to move to 450mm wafers. Additionally, the lack of enthusiasm from other chip manufacturers and the unclear return on investment for equipment makers further diminish the likelihood of ASML pursuing larger wafer processing capabilities. Therefore, the 12-inch wafer size has remained unchanged as the standard for over 20 years.
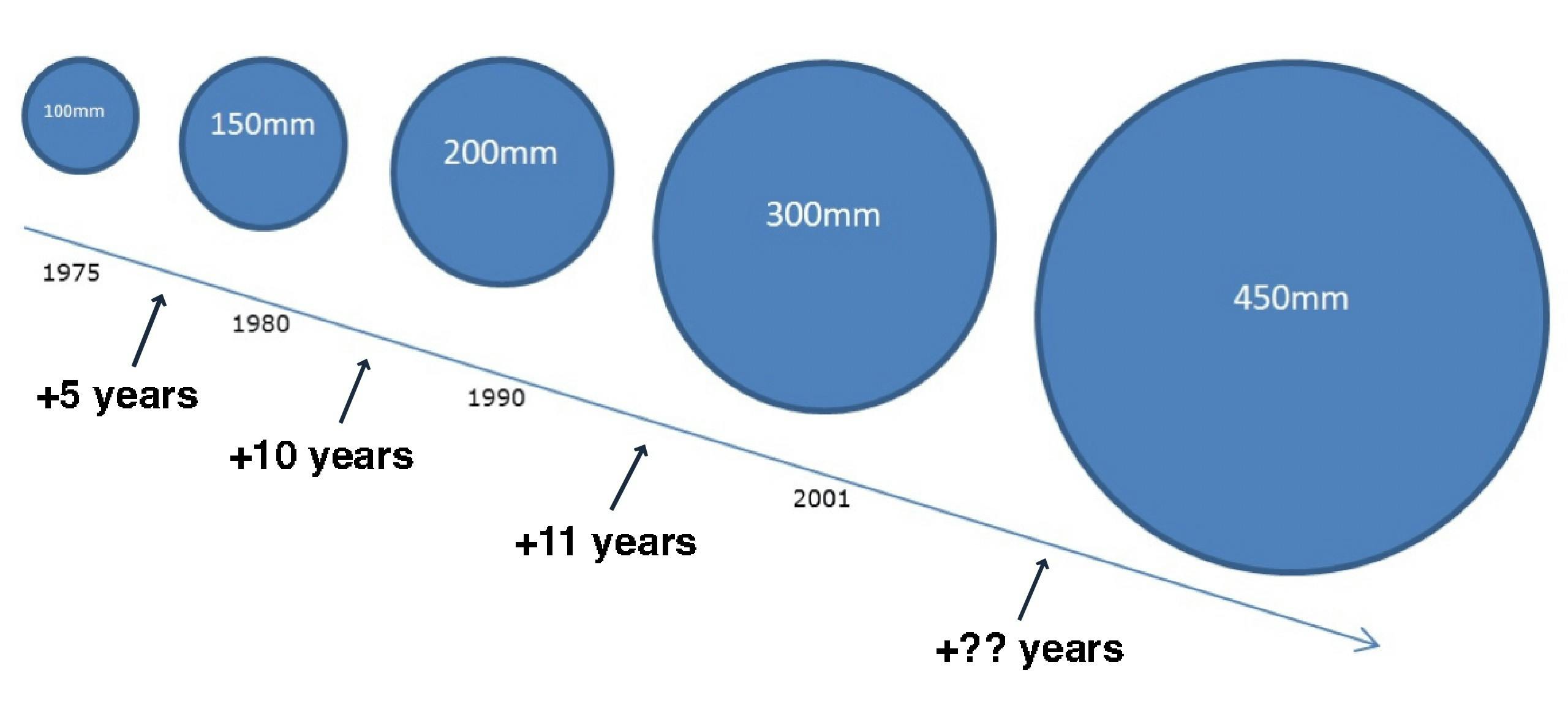
Source: AnySilicon, Contrary Research
Second, because of the large size of Cerebras' chips, the probability of defective dies increases. As a single imperfection could render the entire chip inoperative, Cerebras has allocated 1% of its cores to act as spare cores while redundant communication links route around the damaged cores to the spares. However, this solution adds complexity to the manufacturing process, which could impact Cerebras' ability to produce chips at scale efficiently and cost-effectively.
Changes in Domestic Policy & Emerging Geopolitical Risks
The United States government has demonstrated its willingness to restrict the export of advanced computing technologies to certain countries, particularly China. For example, the US has limited the export of Nvidia GPUs to China due to concerns over potential military applications. As Cerebras’ systems offer greater performance than Nvidia GPUs, similar export restrictions could be applied to Cerebras’ products in the future. While Cerebras has primarily focused on European and Middle Eastern expansion, such as with Aleph Alpha and G42, future geopolitical tensions or shifts in US foreign policy could result in broader export bans or restrictions – impacting Cerebras’ ability to expand into international markets.
Top of mind in geopolitical tensions is the US-China conflict over Taiwan, home to the Taiwan Semiconductor Manufacturing Company (TSMC). Cerebras relies on TSMC for chip manufacturing, making it vulnerable to these tensions. China’s ambitions regarding Taiwan threaten the global semiconductor supply chain, with TSMC being a critical node. Disruption to TSMC’s operations due to political instability or conflict could severely impact Cerebras’ ability to manufacture its products.
Data Center Construction Complexity
Data centers are being constructed at an unprecedented rate to meet the demands for AI training and inference. In 2023, the market supply for data centers in North America grew 26%, reaching an all-time high of 3.1K megawatts of future data centers under construction, representing a 46% year-over-year increase.
Compared to typical data center racks, operating AI data centers requires vastly larger amounts of electricity. While a typical data center rack may consume five to ten kilowatts of power, a single rack in an Nvidia superPOD data center can use over 40 kilowatts. Meanwhile, Nvidia's GB200 NVL72 consumes up to 120 kilowatts per rack. As AI models grow larger and more computationally intensive, some projections suggest that AI could consume up to 20% of global electricity supply by 2030.
As a result, power availability has become a critical constraint in building new data centers. In April 2024, nine of the top ten electric utility companies in the US have identified data centers as their main source of customer growth, while one data center company owner claimed that the US was nearly “out of power” for additional data centers. This has caused some jurisdictions, including established data center hubs, to curtail construction due to energy concerns. For instance, Northern Virginia, the world's largest data center market, recently rejected a data center application for the first time in the county’s history due to power availability issues. Industry leaders, including Meta's CEO Mark Zuckerberg, have also noted that energy constraints have limited their ability to build larger computing clusters.
The rapid growth of AI model size and computational requirements further exacerbates this challenge. While internet traffic took about a decade to increase by a factor of 20, cutting-edge AI models are becoming four to seven times more computationally intensive each year.
These electric grid limitations may hamper Cerebras’ ability to sell and deploy its computing systems. This infrastructure bottleneck could slow the adoption of Cerebras' technology and limit its market expansion, giving a competitive edge to established companies with existing data centers like Google Cloud, Microsoft Azure, and AWS.
Rise of Small-Scale Models Over Large-Scale Models
Cerebras’ computing systems are optimized for training large-scale AI models. Feldman previously stated that Cerebras’ target customers were those working on “the hardest and largest, most complicated of the artificial intelligence problems.” However, for smaller models, Cerebras’ computing systems are less cost-effective than traditional GPU-based solutions like Nvidia’s. This specialization may limit Cerebras’ market appeal for companies working with smaller AI applications.
Moreover, there is an increasing trend towards small-scale AI models due to their efficiency, lower cost, and performance capabilities. Companies are realizing that cheaper, specialized models often make more sense than large general models for most real-world AI applications. Improvements in training technology have enabled smaller models to match or even outperform larger models on focused tasks while costing a fraction to train. For example, a paper published by researchers at UC Riverside in July 2024 showed a new training approach for high-quality models while achieving 14 times lower costs than traditional approaches. As new methodologies are created for efficient training of high-quality, small-scale models, Cerebras may find its market share shrinking rather than growing.
For example, Microsoft's 2.7 billion parameter model Phi-2 can outperform models 25 times larger on complex language tasks. In another case, Microsoft’s Phi-3-mini can outperform models twice its size on tasks like content creation. Finally, in July 2024, Salesforce’s AI research team released two models tuned for function calling: xLAM-1B, a 1 billion parameter model that beats GPT3.5-Turbo, and xLAM-7B, a 7 billion parameter model that’s on par with GPT-4. For reference, GPT-4 is rumored to have 1.7 trillion parameters — roughly 243 times larger than xLAM-7B.
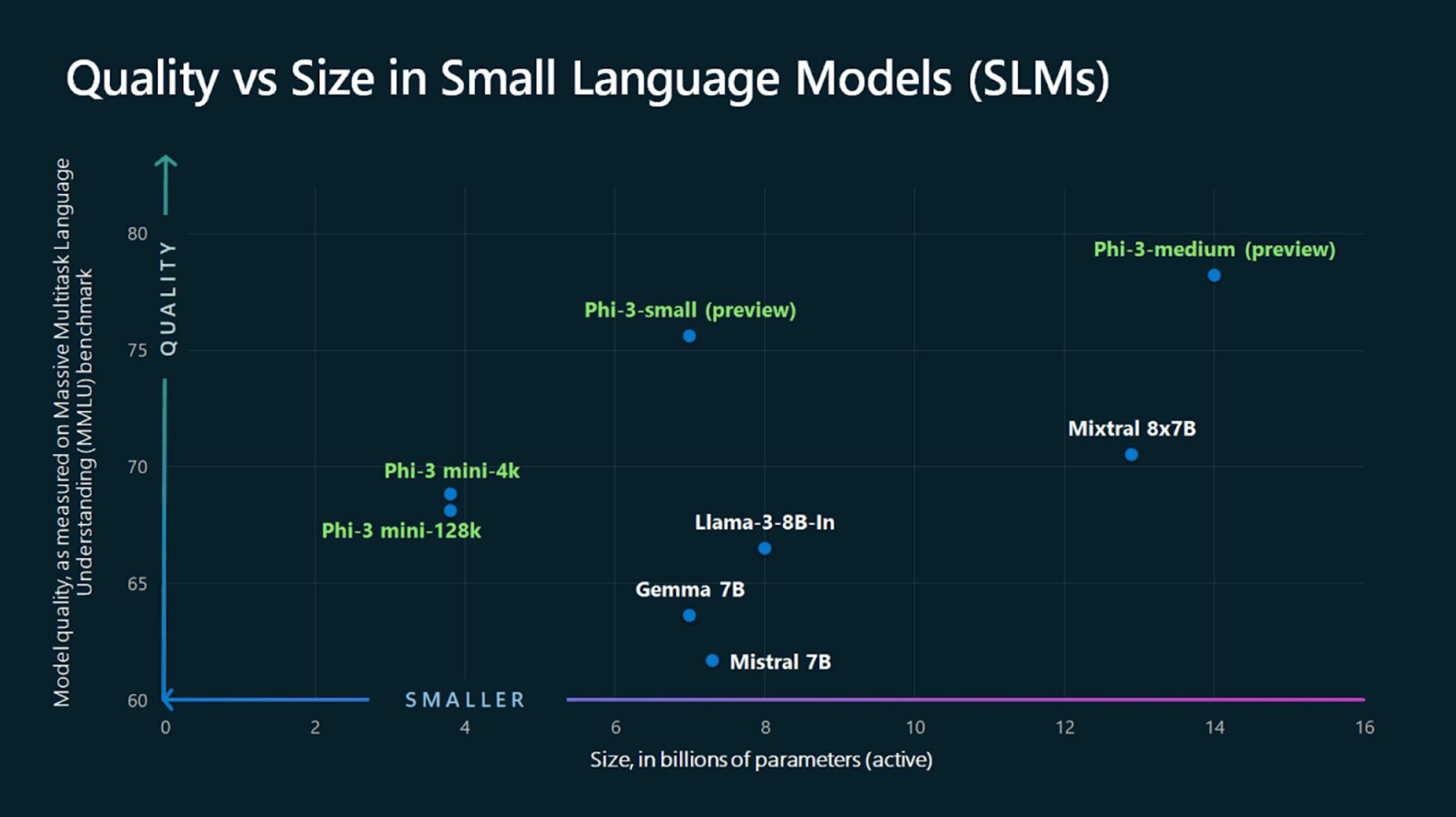
Source: Microsoft
This trend is concerning for Cerebras, which can’t necessarily handle small-scale AI training as efficiently. If small-scale AI models become the dominant approach, Cerebras may find its products overspecialized for a less common market approach. The company’s value proposition is built around handling large-scale models, but if the market shifts to smaller AI applications, Cerebras’ competitive advantage could diminish, reducing demand for its high-performance systems.
CPUs for Inference
Currently, AI inference is performed on specialized AI chips like the Qualcomm Cloud AI 100 accelerator. However, advancements in CPU technology (x86, ARM, and RISC-V) and the nature of inference tasks may shift this paradigm. First, CPUs have benefitted from decades of toolchain investment, resulting in a mature ecosystem of development tools and community support. Second, CPUs are generally cheaper to run than GPUs or specialized AI chips. As inference tasks use fixed model weights – unlike training tasks – inference can be easily distributed across many CPUs. As CPUs continue to advance and their cost-effectiveness for inference tasks improves, they could become the dominant hardware choice for AI inference.
If the shift towards CPU-based inference becomes widespread, it could threaten Cerebras’ entry into the inference market. While Cerebras has optimized models for specialized chips like Qualcomm’s Cloud AI 100, optimizing for commodity CPUs may present unique challenges. The company’s expertise in high-performance AI systems and specialized hardware may not directly translate to general-purpose CPU architectures. As a result, Cerebras may find it more challenging to establish a strong position in CPU-based inference optimization, hindering its ability to diversify revenue streams and capture the growing inference market.
Advances in AI Training Techniques
Cerebras’ chip technology is based on optimized matrix multiplication, which is used to train all currently available AI models. Because 50% to 98% of all data in a deep-learning training set are zeros, the SLAC cores on each WSE chip skip these multiplications, reducing unnecessary computations and accelerating training speed.
However, recent AI developments suggest that Cerebras’ highly specialized approach may become obsolete. In June 2024, a new method for training LLMs was unveiled that doesn’t rely on matrix multiplication, which Cerebras’ chips specialize in. This shift in AI training paradigms could render Cerebras’ solutions for faster linear algebra computations less relevant. Although the possibility is low, as better training techniques are discovered, Cerebras’ chip architecture could become obsolete.
Summary
In past technological shifts, new leaders emerged as incumbents fell: Cisco and Broadcom in networking, Intel and AMD in PCs, Qualcomm in mobile phones, and Nvidia in advanced graphics. Similarly, the AI revolution presents an opportunity for Cerebras to establish itself as a dominant player in this new computing paradigm.
Cerebras’ approach to computing offers substantial speed and cost advantages in AI training compared to traditional GPU clusters. With a growing customer base and nearing $1 billion in revenue, Cerebras shows strong market traction. However, competition from established and emerging players, geopolitical risks over Taiwan, and changes in future AI methodologies pose key challenges. If successful, Cerebras could play a historic role in shaping the future of AI computing infrastructure.