Thesis
The phrase “data is the new oil” appeared as early as 2006, as the data produced by the internet began to take off. More than 463 exabytes (1 billion gigabytes) of data will be created worldwide by 2025. The future of digital businesses will be defined by their ability to capture value from data effectively. From devices to the cloud, data quantity is exploding. This proliferation represents an opportunity for organizations to build a data advantage. The global big data and business analytics market was valued at $198.1 billion in 2020 and is projected to reach $684.1 billion by 2030.
Studies have shown that companies prioritizing data-driven insights are 23 times more likely to attract new customers, 19 times more likely to be profitable, and can experience growth rates seven times faster than GDP growth. Businesses want to harness data to their advantage, including structured and unstructured data that varies in velocity and volume. However, in attempting this they face obstacles such as navigating complex legacy infrastructure, addressing data silos, and managing high latency issues. This has created the need for data lakes, which are storage repositories that can ingest large amounts of raw data in their native format, enabling businesses to access it whenever needed and empowering data scientists to apply analytics to get insights. The global data lake market was valued at $11.7 billion in 2021 and is expected to reach $61 billion by 2029.
Databricks helps organizations prepare data for analytics, empower data-driven decisions, and adopt machine learning. It was founded by the original creators of popular open-source projects, including Apache Spark, Delta Lake, and MLflow. It helps its customers unify their analytics across business functions, data science, and engineering. It also enables data science teams to collaborate with data engineering and other lines of business to build data products. Its journey started as a data lake supporting open-source Apache Spark. Today, it has expanded into a broader platform, including a Databricks Marketplace incorporating data storage and positioning itself as a data lakehouse.
Founding Story
Databricks was founded in 2013 by seven computer science Ph.D. students at UC Berkeley: Ali Ghodsi (CEO), Ion Stoica (Executive Chairman), Matei Zaharia (Chief Technologist), Patric Wendell (VP of Engineering), Reynold Xin (Chief Architect), Andy Konwinski (now advisor), and Arsalan Tavakoli-Shiraji (SVP of Field Engineering).
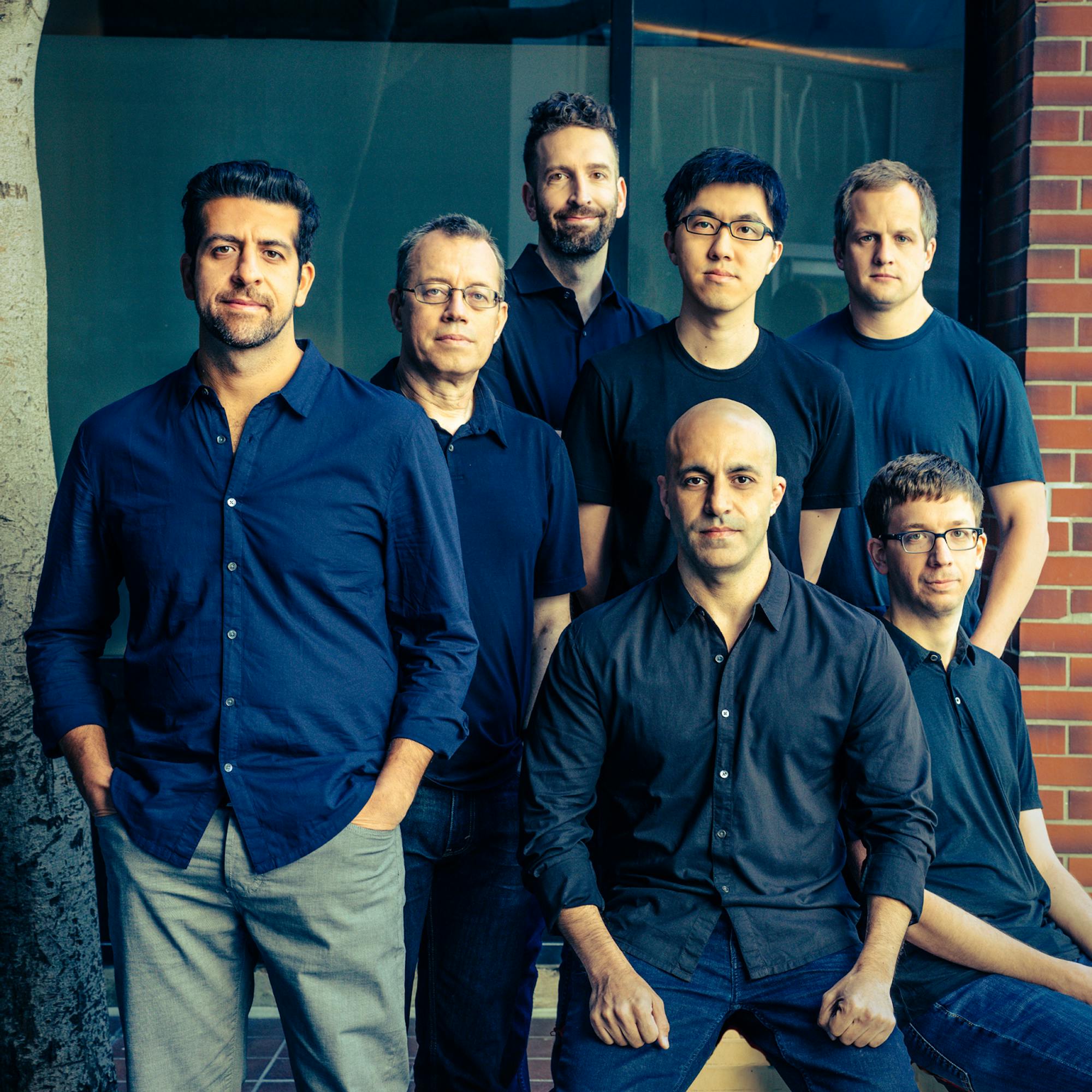
Source: Forbes
Ghodsi and his family fled Iran in 1984 due to the Iranian Revolution. They eventually settled in Sweden, where Ali spent his childhood in the suburbs, surrounded by computers. At age five, Ghodsi’s parents bought him a used Commodore 64, which became his source of entertainment and also spurred his curiosity. He started reading the manuals that came with the computer and became a self-taught programmer. In 2009, Ghodsi came to the United States as a visiting scholar at UC Berkeley, where he got his exposure to Silicon Valley.
Ghodsi joined Matei Zaharia, then a Ph.D. student, on a project to build a software engine that they dubbed Spark for data processing. They wanted to replicate what the big tech companies did with neural networks without the complex interface. This project set a world record for the speed of data sorting in 2014 and won Zaharia an award for the year’s best computer science dissertation.
Eager for companies to use their tool, they released the code for free but soon realized it wasn’t gaining any real traction. Over a series of meetings at Indian restaurants beginning in 2012, the seven academics agreed to join together to found Databricks. Entrepreneurial advice for the startup came from Zaharia’s thesis advisors, Scott Shenker and Ion Stoica, two well-respected academics. Stoica was an executive at video streaming startup Conviva, while Shenker had been the first CEO at Nicira, a networking firm sold in 2012 to VMware for about $1.3 billion. Stoica became the CEO, and Zaharia became the chief technologist. Shenker, who joined the board rather than working full-time for the company, arranged the initial meeting between a16z’s Ben Horowitz, an early Nicira investor, and the team.
The company raised a Series A funding round led by a16z in 2013. In 2015, Ion Stoica had to step away from his role as CEO to fulfill his commitments as a professor at Berkeley. Ghodsi became CEO in January 2016. A year into his journey as CEO, the company landed its first million-dollar deal. Under Ali's leadership, the company's recurring revenue increased to $40 million by the end of 2017, $100 million in 2018, and reached a revenue run-rate of $200 million during Q3 of 2019.
Product
Databricks initially focused on Apache Spark for querying large, unstructured data sets stored in data lakes. Databricks has since diversified its revenue streams by introducing products that cater to related markets, including AI lifecycle management/MLOps (MLFlow), data warehousing (Delta Lake), and data visualization (Redash).
Databricks Lakehouse Platform
Databricks Lakehouse Platform is a unified set of tools for building, deploying, sharing, and maintaining enterprise-grade data solutions at scale. It is offered on major cloud services including Amazon Web Services (AWS), Microsoft Azure, Google Cloud Platform (GCP), and Alibaba. The platform is built on Apache Spark, an open-source distributed computing framework, and includes various components, including Delta Lake, which provides ACID transactions and data versioning for data lakes; MLflow, which is an open-source platform for managing machine learning workflows; and Redash, a collaboration tool for SQL-based data analysis.
Databricks Lakehouse Platform combines elements of data lakes and data warehouses. It has the flexibility, cost-efficiency, and scale of data lakes while also providing the data management and ACID transactions of data warehouses. This enables business intelligence (BI) and machine learning (ML) on all data.
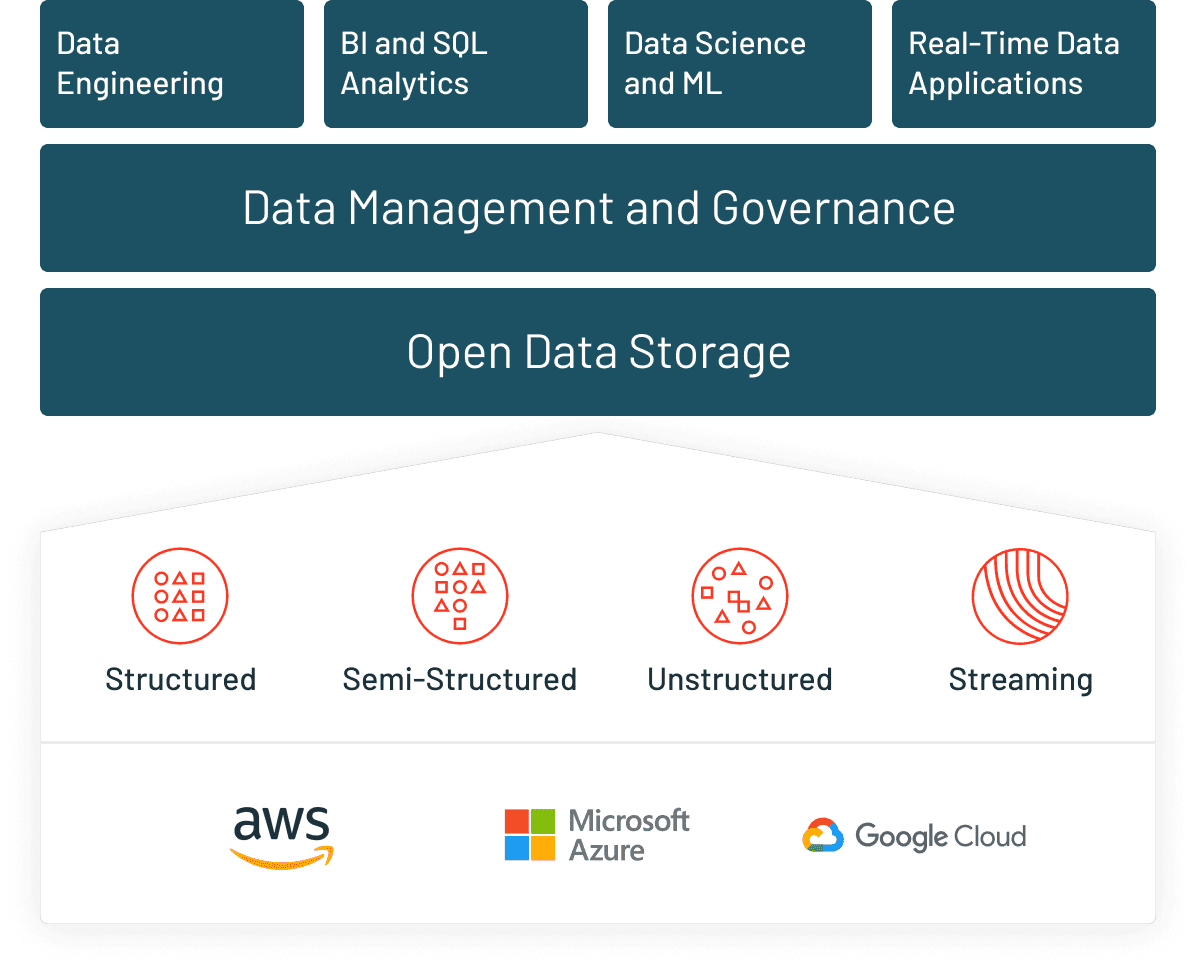
Source: Databricks
Delta Lake
Delta Lake is an open-source storage layer designed to run on top of data lakes and improve reliability, security, and performance. It delivers a single source of truth for all customer data, including real-time streams, so data teams are always working with the most current data. It supports ACID transactions, scalable metadata, unified streaming, and batch data processing. Delta Lake enables data management and governance, providing an additional layer of control to an open storage environment that can handle structured, semi-structured, and unstructured data.
Unity Catalog
Unity Catalog is a unified governance solution for all data and AI assets, including files, tables, machine learning models, and dashboards in customers’ lakehouses on any cloud. Customers can use standard SQL functions to define row filters and column masks, allowing fine-grained access controls on rows and columns. They can also find, understand, and reference relevant data from across data estate with a unified search experience for data analysts, engineers, and scientists.
Data Engineering
Data teams can eliminate silos with a single and unified API to ingest, transform, and incrementally process batch and streaming data. Databricks manages the customer’s infrastructure and the operational components of production workflows.
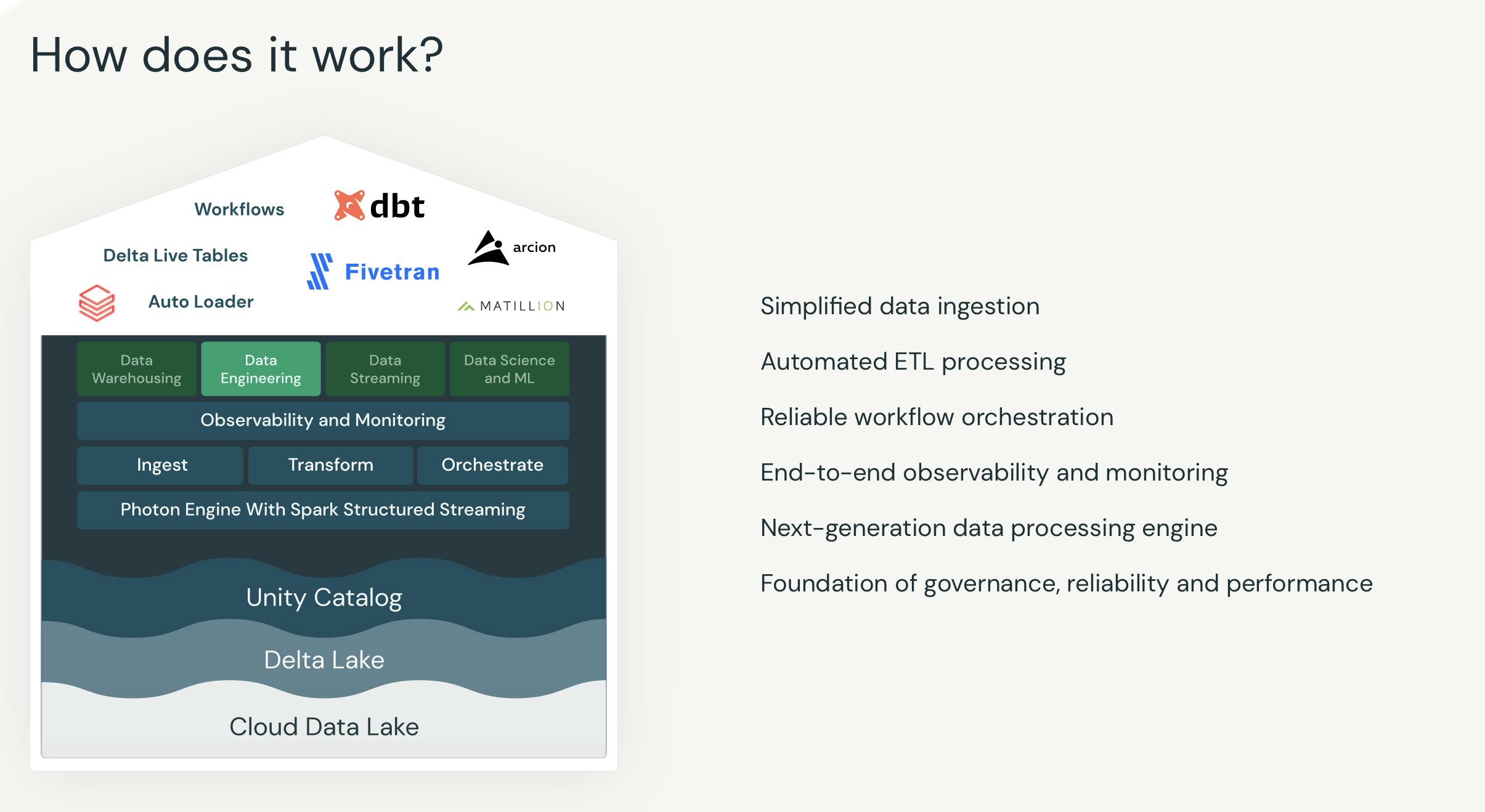
Source: Databricks
Data Streaming
Databricks simplifies data streaming to deliver real-time analytics, machine learning, and applications on one platform. It enables data teams to build streaming data workloads with the languages and tools they already know. It simplifies development by automating the production aspects of building and maintaining real-time data workloads.
Databricks SQL
Databricks SQL is a serverless data warehouse on the Databricks Lakehouse Platform that lets users run SQL and BI applications at scale with up to 12x better price/performance, a unified governance model, and APIs — all while utilizing their tools of choice.
Delta Sharing
Delta Sharing is an open protocol developed by Databricks for secure data sharing with other organizations regardless of their computing platforms. With Delta Sharing, Databricks users can avoid vendor lock-in and share existing data in Delta Lake and Apache Parquet formats with a person or group outside of their organization, irrespective of which data platform the recipient is using.
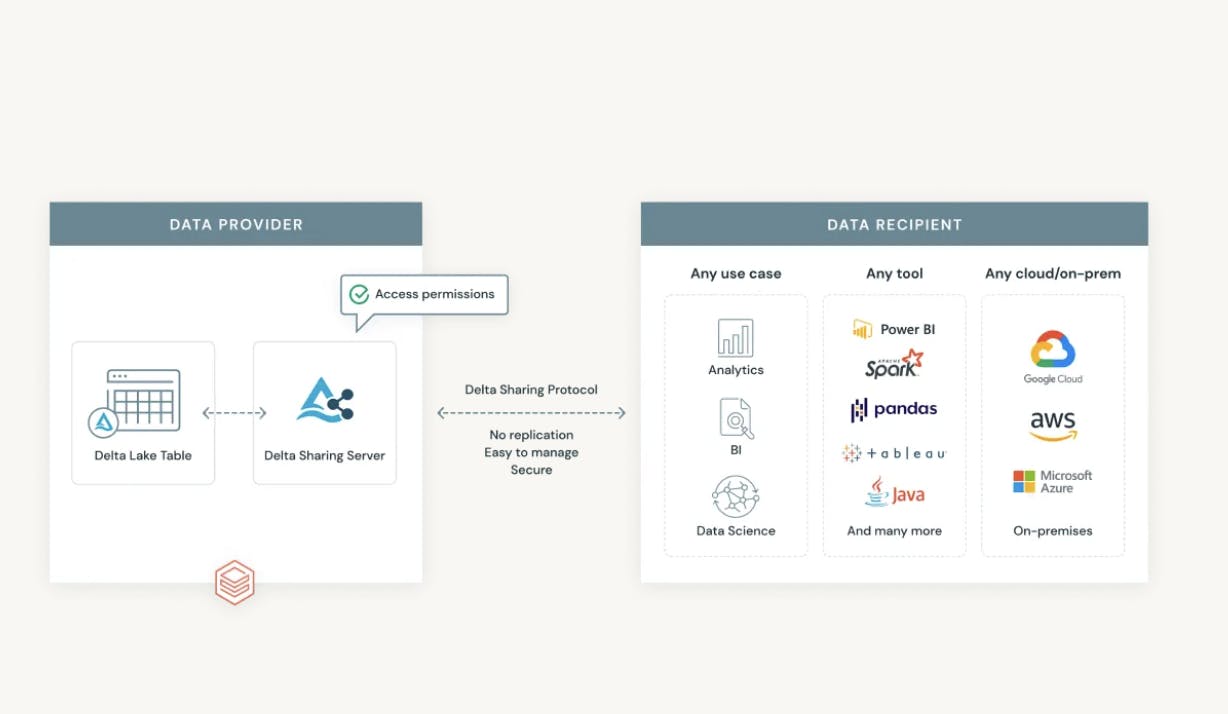
Source: Databricks
Machine Learning
Databricks Machine Learning empowers ML teams to prepare and process data, streamline cross-team collaboration, and standardize the full ML lifecycle from experimentation to production. It provides tools for tracking experiments, packaging code into reproducible runs, and sharing and deploying models.
Data Science
Databricks helps streamline the data science workflow end-to-end — from data preparation to modeling to sharing insights. Users can access clean data, preconfigured compute resources, IDE integration, multi-language support, and built-in visualization tools.
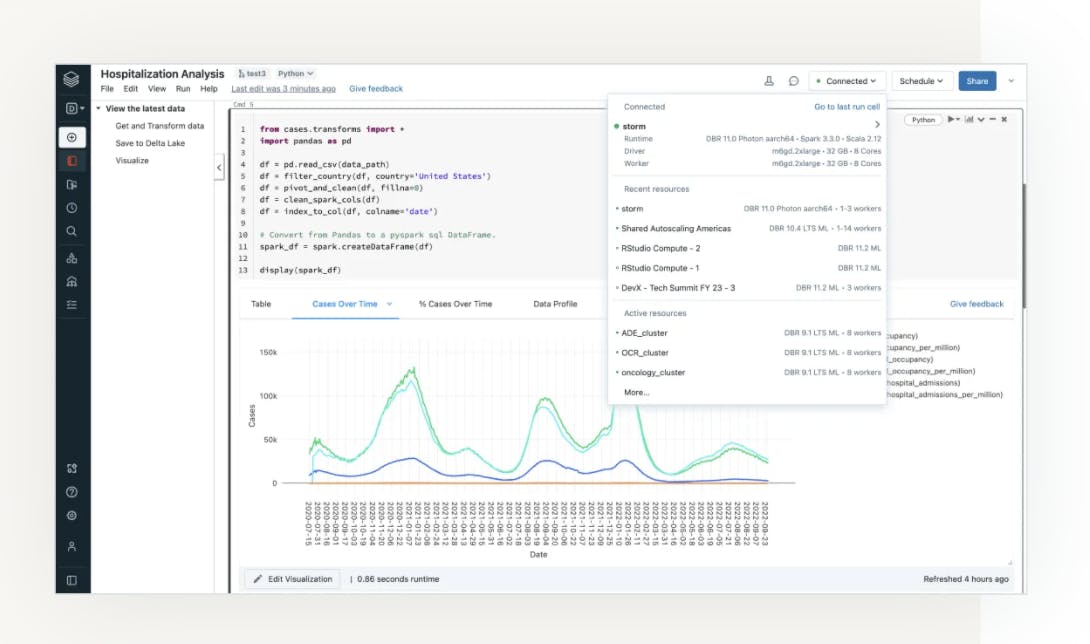
Source: Databricks
Market
Customer
Databricks sells to large enterprises, small businesses, and those in between. It counts many of the world’s most well-known companies as customers, including Microsoft, Atlassian, Apple, Disney, HSBC, etc. It is used across retail, advertising, entertainment, financial services, energy, gaming, healthcare, and manufacturing. Databricks is used by all data team members, including data engineers, analysts, business intelligence practitioners, data scientists, and machine learning engineers.
To understand how much customers spend on Databricks, we can estimate its Average Contract Value (ACV) based on several data points: By the end of Q2 2022, it had $1 billion in ARR and over 7,000 customers. Based on those rough numbers, we can estimate that Databricks’ ACV is at ~$143K, which is ~47% that of Snowflake (which is estimated to have a ~$301k ACV as of March 2023).
Market Size
The global data analytics market is projected to grow from $271.8 billion in 2022 to $655.5 billion by 2029. Companies are moving away from siloed systems to store their data, instead opting for centralized data stores. This approach helps them gain insights into past and future trends with business intelligence and predictive analytics. Databricks is riding this trend to grow its business since its technology is based on a data lake, allowing all data types and sources to be stored together. The data lake market was valued at $7.9 billion in 2019 and is expected to reach $20.1 billion by 2024.
Competition
Snowflake
Snowflake, founded in 2012 by ex-Oracle architects, began its journey by positioning itself as a cloud data platform offering data warehousing and analytical compute workloads. Historically, Snowflake has had users like business analysts and data engineers, while Databricks has been favored by data scientists and ML engineers. However, the lines between the two have been blurred as each company has introduced new products competing in the other’s domain. For instance, Snowflake has developed new offerings such as Snowpark for Data Science, transactional databases, and Python support to appeal to open-source developers and data scientists. Conversely, Databricks has launched offerings such as Databricks SQL, Delta Lake features, and Unity catalog, catering to data storage and security-conscious customers.
While Snowflake is often considered a closed platform because it controls its entire storage and computes platforms in a closed ecosystem, Databricks is open-source. All of Databricks' key product lines can be implemented for free, and customers can turn to Databricks' enterprise offerings for more advanced features and support. Snowflake provides an off-the-shelf solution, allowing companies to get basic analytics off the ground quickly, while Databricks offers greater customization and configuration, giving customers full control over their setup. Snowflake recorded $2.1 billion in annual revenue at the end of 2022, while Databricks crossed the $1 billion annual run rate in the second quarter of 2022 with estimated annual revenues of $1.4 billion in 2022. Refer to our deep dive for more information on the growing competitive dynamics between Snowflake and Databricks.
Cloud Providers
While Databricks capitalized on the shift to the cloud and built its business on top of major cloud providers, including Amazon Web Services (AWS), Microsoft Azure, and Google Cloud Platform (GCP), it competes with the major cloud providers’ own proprietary offerings across several areas.
For instance, in the case of big data processing, AWS offers Amazon EMR, Azure has Azure HDInsight, and GCP has Dataproc. Additionally, the major cloud providers have their own business analytics solutions, including AWS's Amazon QuickSight and Amazon FinSpace, Azure's Power BI Embedded and Microsoft Graph Data Connect, and GCP's Looker and Google Data Studio, all of which compete against Databricks.
Specialized Solutions
Databricks competes with specialized solutions in data management and data science spaces that run specific tasks. For example, Databricks’s scheduler is similar to Apache Airflow, and its MLflow offering competes with DataRobot and Alteryx. Databricks has an advantage by owning the whole pipeline from data coming into deploying ML models.
Business Model
Databricks develops open-source software for data processing and AI applications and offers a paid version with additional proprietary features. While open-source software provides flexibility and avoids getting locked into a proprietary architecture, most companies need more engineering resources to manage complexity. This is where Databricks has stepped in, providing enterprises with a fully managed version of their open-source software, with additional utilities like SaaS tools for writing queries and connectors to connect data sources.
Databricks offers a pay-as-you-go model where customers are charged based on how much compute resources they consume and use per-second billing to ensure customers only pay for what they need when it is needed. To measure and price the processing power, Databricks uses a normalized unit called a Databricks Unit (DBU). The number of DBUs a workload consumes is driven by several processing metrics, including the compute resources used, the amount of data processed, the region, the pricing tier, and the type of Databricks service being used.
Databricks offers a 14-day free trial for users to access Interactive notebooks to use Apache SparkTM, SQL, Python, Scala, Delta Lake, MLflow, TensorFlow, Keras, Scikit-learn, and a collaborative environment for data teams to build solutions together.
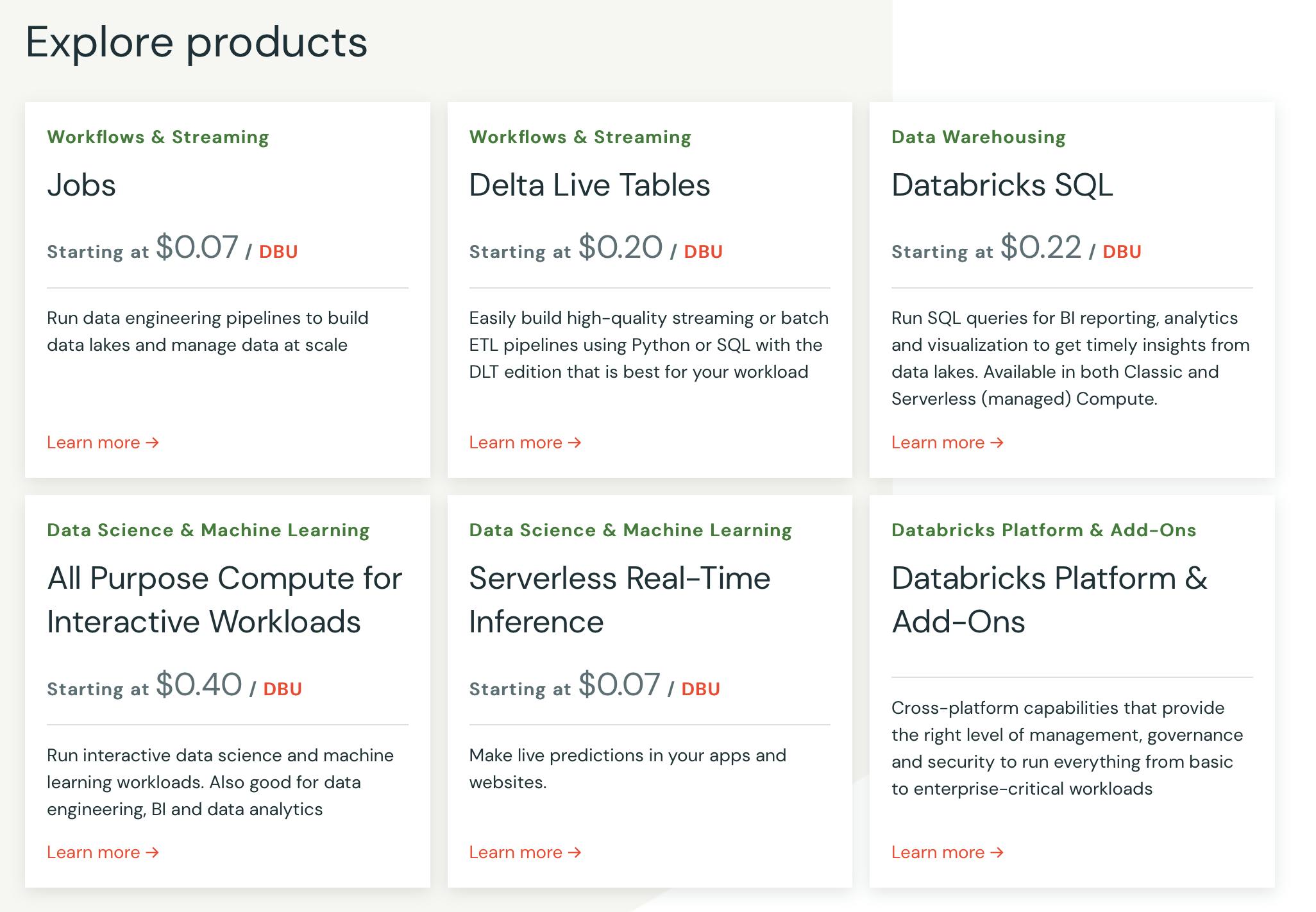
Source: Databricks
Traction
Databricks revealed that it crossed an annual run rate of $1 billion in the second quarter of 2022 and that its revenue was growing at more than 70% annually. It ended Q3 2019 with a $200 million revenue run rate before generating $425 million of revenue in 2020. Databricks topped $800 million in ARR in 2021 and had reportedly generated more than $1 billion in annualized revenue as of August 2022. The company further claimed to have a dollar-based net retention rate (DBNRR) of over 150% as of February 2022.
Databricks grew its total customer count from 400 in December 2016, to more than 500 as of August 2017, to over 2K in February 2019, to more than 5K in February 2021, and to 7K+ as of August 2022. In March 2023, over 9K companies use its platform worldwide, including AT&T, Shell, Burberry, Toyota, Adobe, Conde Nast, and Regeneron.
A 2017 partnership with Microsoft played an important role in Databrick’s growth. That’s when the companies debuted Azure Databricks, a tool for processing and analyzing large corporate data streams. Azure Databricks is integrated with other Azure data-related services, including the Azure Cosmos DB database introduced earlier that same year, Azure SQL Data Warehouse, and Azure Active Directory. At approximately 5K employees in Q1 2023, Databricks has also been one of the few late-stage startups that has continued to expand its team size despite macroeconomic headwinds.
Valuation
Databricks raised $1.6 billion in its Series H round, valuing the company at $38 billion in August 2021. Databricks has raised a total of $3.5 billion. Its investors include Andreessen Horowitz, NEA, Franklin Templeton, Amazon Web Services, Microsoft, Tiger Global Management, and Coatue Management. In October 2022, it was reported that Databricks trimmed its internal share price, which lowered its implied valuation to $31 billion, about 7% lower than at the same time in 2021.
Regarding public market companies comparable to Databricks, Snowflake has a 21.5x revenue multiple as of March 17th, 2023. While the exact enterprise value and revenues for Databricks are not public, its Enterprise Value /Sales can be estimated by using a couple of data points that are public. Based on Databricks’ $38 billion valuation from its latest round and its estimated sales of $1.4 billion as of the end of 2022, Databricks has an implied revenue multiple of 27.1x.
Key Opportunities
Move to Data Centralization
Databricks is well-positioned to take advantage of the trend toward data centralization, given its data lake architecture. With the availability of cheap cloud storage and fast networks, businesses increasingly opt to store all of their data in a central repository rather than relying on separate data stores for different types of data. The Data Lake market was valued at $7.9 billion in 2019 and is expected to grow at 21% CAGR from 2019-2024 to reach $20.1 billion by 2024. This move to centralization allows companies to better understand their business operations through real-time business intelligence and predictive analytics. Databricks is betting that the explosion of data in the digital age will make it impractical for companies to maintain multiple large-scale data stores, leading to the convergence of data lakes and data warehouses into a single platform.
Rise of Artificial Intelligence
Thanks to the release of OpenAI's ChatGPT, AI has been making headlines across industries. The global AI market size was estimated at $119.8 billion in 2022 and is expected to hit roughly $1.6 trillion by 2030. Databricks is poised to ride this wave. The company's Lakehouse platform allows data teams to store and safeguard data, generate analytics and insights, and power the development of machine learning tools. In addition, Databricks offers integrations with popular AI frameworks like TensorFlow, PyTorch, and Scikit-learn, making it easy to build and deploy machine learning models.
In a potential recession, the opportunity for cost savings is another reason why Databricks is well-positioned to benefit from AI. Comcast says AI has helped reduce computation costs by 10x, and J.B. Hunt says it shaved $2.7 million in IT infrastructure spending in 2022. Companies that leverage AI can cut costs and improve efficiency by automating processes and generating manual insights that would take much longer to uncover.
Key Risks
Stickiness of the Data Warehouse
Databricks is betting on a future where companies will stop using separate software for data warehouses and instead shift to Databricks for all data processing/storage requirements. While the data lake market is expected to grow at 20.6% CAGR from 2020-24 to reach $20.1 billion by 2024, the data warehousing market is also expected to experience robust growth even with its larger scale: projected to reach $51.2 billion by 2028, growing at a CAGR of 10.7% from 2020 to 2028. If Databricks’ predicted future does not play out as planned, it may jeopardize its position in the market.
Dependence on Major Cloud Providers
Databricks relies on cloud infrastructure providers like AWS, Microsoft Azure, and Google Cloud Platform for its cloud-based platform. Notably, Databricks’ partnership with Microsoft, when the two partnered to debut Databricks on Azure as a first-party service, was critical to Databricks’ growth and helped grow its revenue from less than $1 million in revenue at the beginning of 2017 to over $100 million in revenue in 2018. Disruptions to Databricks’ relationships with the major cloud providers or changes in pricing could impact Databricks' ability to provide its services.
Summary
In the age of data expansion, Databricks empowers companies to capitalize on their data by developing open-source software for data processing and AI applications and offering paid versions with additional proprietary features for enterprises. The trend towards data centralization presents an opportunity for Databricks to leverage its data lake architecture and offer companies a single data storage and analytics platform. In addition, the rise of AI provides Databricks with an opportunity as it is well-positioned to ride this wave.