Thesis
Enterprises have highly complex customer support operations from diverse product offerings to distinct business units and unique end-user bases. To reduce the burden on their human support agents of doing mundane, repetitive tasks, 58% of companies actively use chatbots as of December 2024. However, large companies feel "deep pain with their customer support," as incumbent chatbot solutions cannot resolve the deeply nuanced, multi-step inquiries that customers often pose, which "erode customer trust."
In a February 2023 study, nearly 75% of respondents reported that chatbots "aren't able to handle complex questions and are often unable to provide accurate answers." Further, a December 2022 study found over 80% of applicants had reported that chatbots "increased their frustration levels," while 63% indicated that their chatbot interaction did not resolve their inquiry at all. Apart from enterprises experiencing reduced customer trust due to ineffective chatbot solutions, Decagon founders Jesse Zhang and Ashwin Sreenivas identified that "a lot of bigger companies were hesitant to use AI in [customer experience] due to it being a black box and not having control over its guardrails or insight into how it's making decisions."
Decagon offers an AI agent designed specifically for enterprise customer support that possesses "human-like" intelligence. The product is focused on being able to reason through deeply nuanced and multi-faceted customer inquiries. The agent itself continuously learns and improves performance over time while it can also autonomously complete actions — like upgrading a subscription tier, setting up a direct deposit account, or processing refunds — on behalf of the end customer. Surrounding the AI agent is tooling that unlocks precise visibility and explainability in how agents make their decisions while enabling all employees, regardless of technical background, to construct custom rules, guardrails, and business logic around the agents.
Founding Story
Decagon was founded in August 2023 by Jesse Zhang (CEO) and Ashwin Sreenivas (CTO).
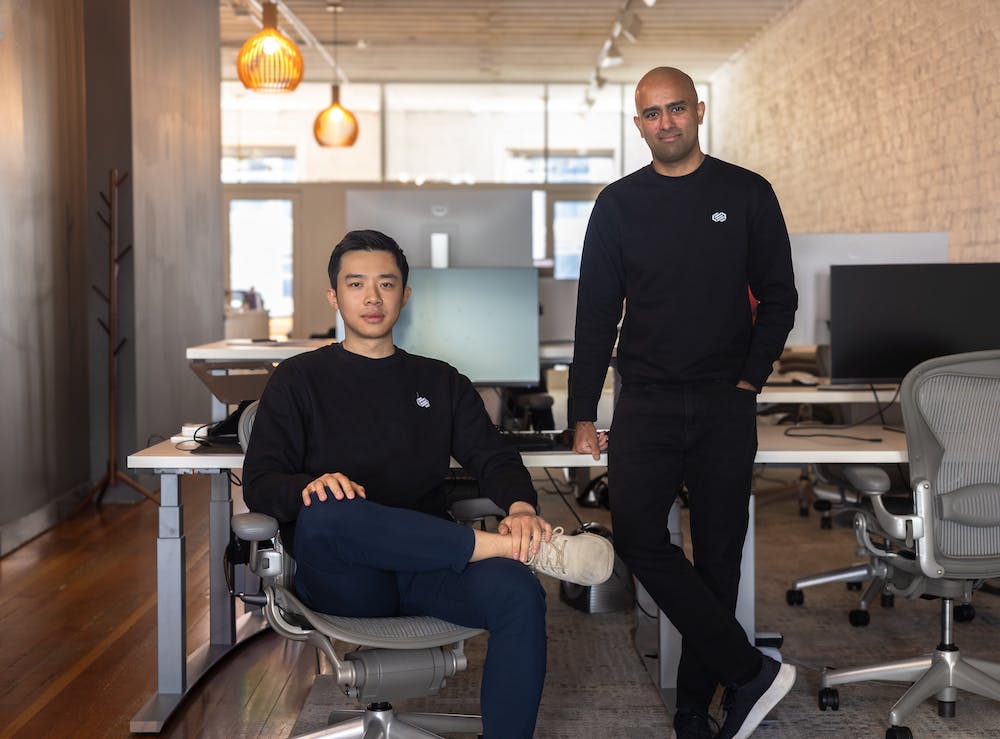
Source: Accel
Zhang’s Background
As Zhang grew up, he frequently did math contests, which helped him become more technical and enter the computer science path. While working toward his bachelor's degree in computer science at Harvard, he "focused on the more traditional internship route" as he held summer roles in quantitative trading at Hudson River Trading and Citadel. He also did an internship in software engineering at Google. After this experience, a few older friends of Zhang’s started getting into startups. The combination of those perspectives helped Zhang increase his conviction in starting his own company.
He initially didn't know what to build but had tried a variety of ideas in the gaming space. When he graduated from Harvard in 2018, he founded Lowkey. Its core product was a clipping software tool for video games that allowed users to capture and share a video recording of their gameplay easily. The company's mission was to "build a social layer on top of and spanning multiple games that [Zhang] felt was missing." Andreessen Horowitz backed the company, and it was in the Y Combinator Spring 2018 class.
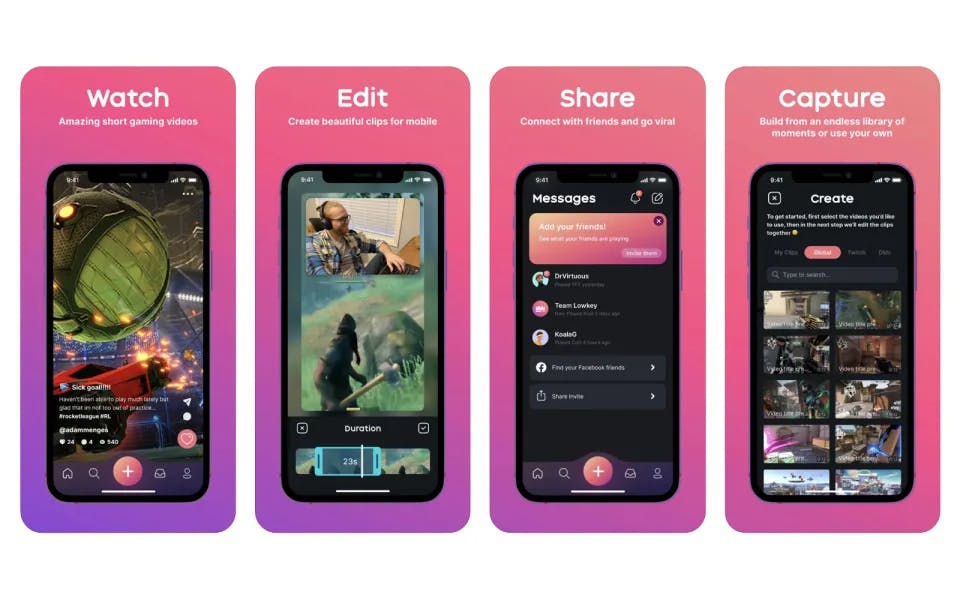
Source: Engadget
In December 2021, after about a year and a half in the market, Niantic then acquired Lowkey for an undisclosed amount. Just a month before, in November 2021, Niantic announced that it raised $300 million at a $9 billion valuation from Coatue to further work toward its vision of building a “real-world metaverse,” applying augmented reality to the physical world in video games. Niantic Ventures invested in Lowkey to “accelerate the social experiences” within its products. After the acquisition, Zhang joined the Social team at Niantic to work on this. On the side, he also became involved as an investor, having served as a scout for Sequoia. As of December 2024, he had made 20+ angel investments in startups, including Pika AI and Cognition.
Sreenivas’s Background
Like Zhang, Sreenivas is also a trained software engineer, having done his undergraduate and graduate studies in Computer Science at Stanford. Before he went to grad school in 2018, Sreenivas spent a year as a Deployment Strategist at Palantir. In this role, he helped companies build digital twins of their operations and served as a “mix between product manager, software engineer, and strategist.” After receiving his master’s degree in 2019, he co-founded Helia alongside two other student alumni and served as the company’s CTO. The team built a computer vision software product that used “deep learning to glean insights from video that are actionable at scale.” In December 2020, Scale AI then announced that it had acquired Helia for an undisclosed amount.
This was a strategic acquisition as Scale AI released a new data engine product, Nucleus, in August 2020. Nucleus was designed to enable data scientists and machine learning engineers to build better datasets, providing advanced tooling for collecting, curating, and annotating datasets for building better machine learning models. Helia was particularly appealing to Scale AI because its computer vision technology enabled Scale AI to more effectively apply metadata tags to objects within videos, which enabled video to be used in training sets for high-quality ML models.
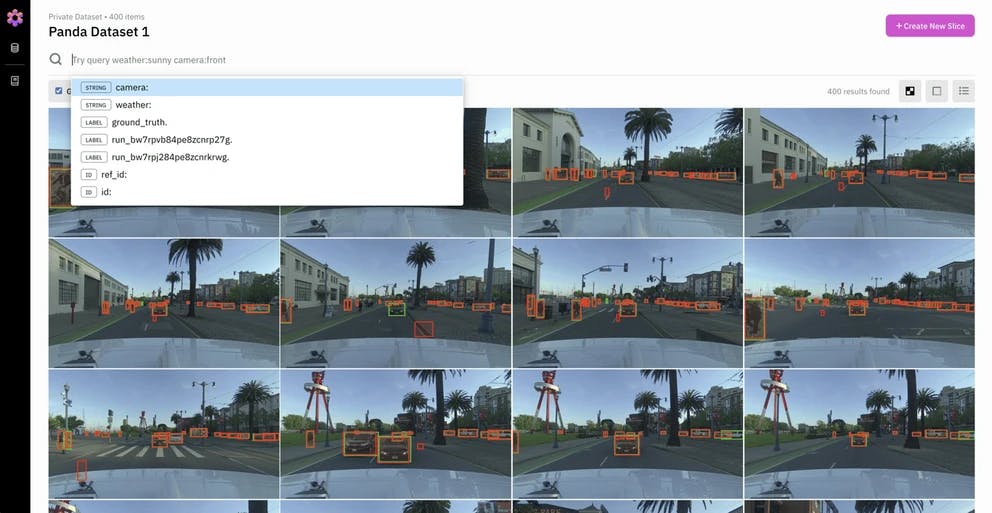
Source: Scale AI
Crossing Paths & Initial Ideation
Zhang and Sreenivas initially crossed paths during an encounter at a founder retreat in Utah hosted by Andreessen Horowitz in 2023. When discussing the fit between them, Zhang mentioned that he and Sreenivas “were both technical and are engineers and are in a similar point in life” and that they “both had a reasonably successful outcome before, but [they] both wanted to build a much bigger company.” The duo saw GPT-4 arrive along with the concept of AI agents. They “knew it would be the basis of the most important companies of this generation,” yet they didn’t initially know what to build. They only spent a few weeks ideating and were very intentional about the questions they asked customers to understand which forms of AI agents “actually will be valuable, which ones will get traction, and where there’s actually ROI.” After the pair surveyed dozens of startups, they arrived at this key insight:
“Enterprise customers felt deep pain with their customer support. While enterprises had previously implemented chatbots, these previous generation solutions eroded customer trust. Incumbent chatbots were a maze of decision trees and canned responses that ultimately did not solve customer issues.
Specifically, many “bigger companies were hesitant to use AI in [customer experience] due to it being a black box and not having control over its guardrails or insight into how it’s making decisions.” Andreessen Horowitz Investment Partner Kimberly Tan, who had invested in Decagon at inception, saw firsthand Zhang and Sreenivas identifying this enterprise need. “We saw them go through the idea maze and when they landed on support and started chatting with customers, it was very clear that all customers were desperate to have some sort of AI-native support solution.”
The Problem at Hand
Widespread Chatbot Dissatisfaction
Large companies have a “high level of complexity in their support operation, and they require a solution that innovates around the nuances of their business, learns from feedback, and adapts to new conversations.” However, nearly 75% of respondents in a February 2023 study reported that chatbots “aren’t able to handle complex questions and are often unable to provide accurate answers.” Additionally, after even a single negative experience with chatbots, 30% of customers are likely to purchase from a different brand, abandon their cart altogether, or tell others about their poor experience. This inability to resolve complex inquiries common in large companies results in user frustration — a concern for enterprises.
Limitations of Existing Chatbots
Traditional AI chatbots consistently fail for a few reasons. First, they are unable to understand customers’ intent and “often misinterpret the requests,” resultingin their “solving the wrong problem” for the customer. Further, incumbent solutions failed in an enterprise setting because they were unable to “interpret nuanced instructions.” They are programmed to “understand only a very specific set of instructions and falter when the ambit of conversation expands even a little too much.”
As a result of incorrect intent understanding and the inability to work with complex inquiries, 72% of respondents in a December 2022 study reported that their chatbot interactions were a “complete waste of time,” while 80% of consumers mentioned that chatbots increased their frustration level. Zhang emphasized the ineffectiveness of legacy solutions in the enterprise setting, as “it’s clear that while [enterprises] want greater operational efficiency, it cannot come at the expense of customer experience — no one likes chatbots.”
Aaref Hilaly, Partner at Bain Capital Ventures, commented that this lack of effectiveness is primarily due to existing solutions’ technical rigidity: "They give you a menu of responses, and if your issue falls outside of that narrow window, you’re out of luck until a real person can resolve your challenge. At best, it’s inefficient; at worst, hugely frustrating.” Decagon’s AI Agent Engine solves this problem with AI agents that provide more intelligent, context-aware, and nuanced customer support in the enterprise setting.
Customer Support Well-Suited For AI Agents
Zhang discussed two specific properties that make the customer support use case an effective domain to apply AI agents as “the business structure of the [use case] has to really be on your side,” and “the structure of customer support is probably what allowed it to be the first golden use case for LLMs and agents.”
First, for AI agents to be effective, it must be an incremental solution to existing infrastructure. Use cases that require AI agents to solve 100% of problems are not ideal — they need some sort of safety net. In customer support, AI agents don’t need to solve every single customer inquiry, as companies already have well-developed human support teams and operations that can field a request should the AI agent be unable to resolve it. In a use case like security in which even a single mishandled instance could result in catastrophe for a business, AI agents wouldn’t be appropriate.
Second, agent-based products should have measurable ROI. This is because, as of December 2024, AI agents are a novel technology that enterprises are just beginning to test, and they are “taking a leap of faith because [AI agents are] a very unfamiliar territory.” With customer support AI solutions, enterprises primarily care about average customer satisfaction (easy to quantify) and the percentage of customer inquiries resolved autonomously — which can easily be mapped back to labor cost savings. Even if an AI agent solves only 50% of the inquiries autonomously, this added value is concrete and helps prospective customers justify adopting this new technology.
In 2023, Zhang and Sreenivas founded Decagon because of enterprises’ poor experience with incumbent chatbots, hesitancy to adopt customer support AI due to limited visibility into agent decision-making, and the structural alignment of the customer support use case with AI agents.
Product
AI Agent Engine
Decagon’s core product is an AI agent for enterprise customer support that displays human-like intelligence, provides nuanced responses, and completes tasks on behalf of the customer while continuously learning over time. The AI agent is one component within a unified product called the AI Agent Engine. This platform is a “data flywheel” that helps the agent continuously learn and improve over time. Because enterprises were hesitant to adopt customer support AI solutions due to the lack of visibility into how decisions were being made, and the inability to provide custom guardrails, Decagon provides a no-code tooling suite that resolves these concerns. The five elements of the AI Agent Engine are described below:
Core AI Agent: The customer-facing, chatbot-like interface that is the “intelligent core agent [that] handles all the complex logic to respond to customers, proactively adapting to customer needs.”
Routing: If the AI agent cannot resolve a customer inquiry, it intelligently directs the inquiry to a human agent for manual support. This component enacts the ‘safety net’ that ensures the AI agent isn’t entirely relied upon and has a fallback option.
Agent Assist: If an inquiry is routed to a human agent, Agent Assist makes it easier for human agents to resolve the request by surfacing the most relevant information from across the agent’s knowledge base. It serves as a “strategic copilot for [the] human agent with real-time suggested responses and insights.”
Admin Dashboard: Serves as a control center for customers where they can analyze granular and themed insights aggregated across all customer interactions. If there are common areas of end customer confusion, the admin can include new documentation in the AI’s knowledge base so it can better handle these topics in the future.
QA Interface: This is the “audit layer, offering transparency into AI decision-making and self-serve adjustment options, ensuring [customers] maintain full control over agent behavior.” Here, customers can provide feedback in natural language to the AI agent so they can better answer these topics in the future.
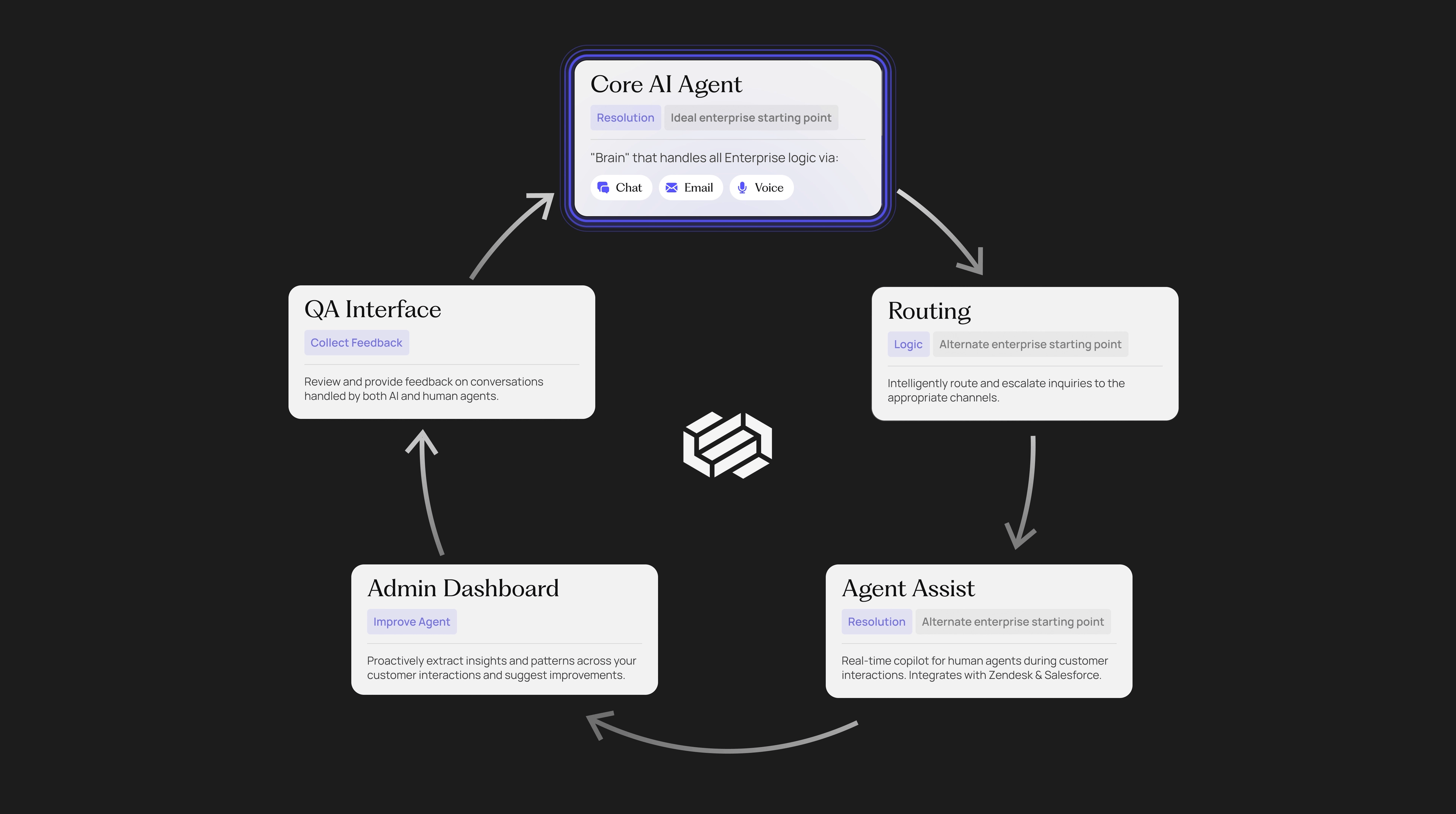
Source: Decagon
Core AI Agent
The Core AI Agent is the chat-based interface with which end users interact. It can be thought of as the “brain that handles all enterprise logic.” It is the “primary point of contact for customer inquiries” and easily integrates with Decagon customers’ native website or mobile app. Contrary to traditional chatbots, the AI agent reflects human-like intelligence that delivers “context-aware answers with conversational AI that are tailored to the customer’s query.”
The AI agent is not only able to answer questions quickly with higher accuracy, but it is also integrated with a suite of applications, including Salesforce, Zendesk, Snowflake, Stripe, and the customer’s internal APIs that enable it to autonomously complete repetitive tasks on behalf of the customer. These tasks may include processing refunds, submitting support tickets, and upgrading customers’ subscription tiers. This drastically reduces human agents' time on mundane, repetitive tasks.
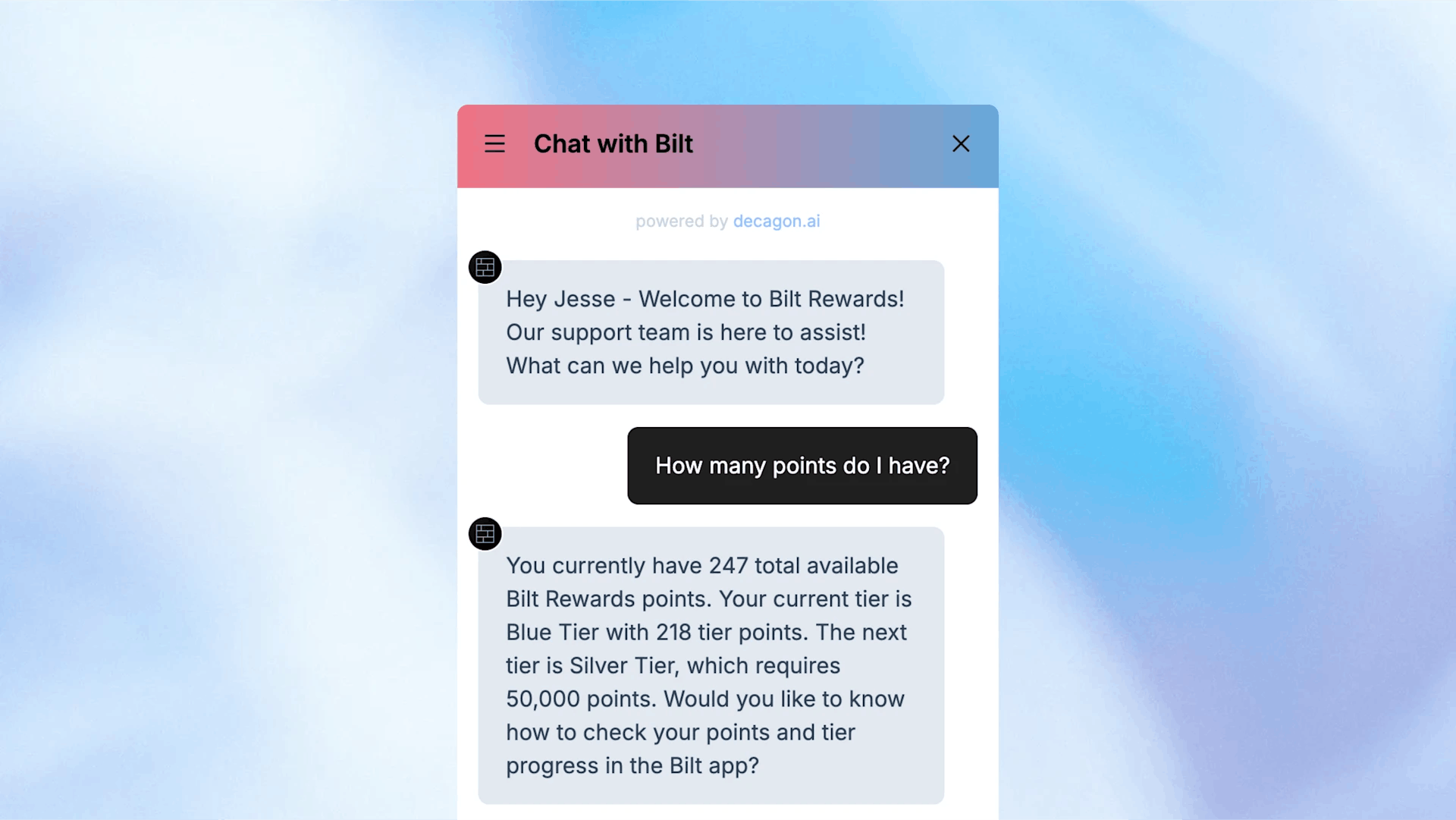
Source: OpenAI
AI Agent Effectiveness
As of October 2024, Decagon’s agents resolve between 70% to 90% of customer inquiries. As of February 2023, almost 75% of consumers indicated that chatbots couldn’t handle complex questions and were often unable to provide accurate answers, while the majority of customers reported that chatbot interactions did not resolve their questions as of December 2022.
The improved resolution rates of Decagon’s AI agents have enabled enterprises to justifiably cut down on the number of human support agents they employ while retaining the best of the best. Thatcher Foster, Vice President of Customer Success at Bilt, emphasized that “working with Decagon was like hiring 65 [human] agents overnight” and that the company’s “agent teams became much stronger, and the ones we retain are real product experts.”
Routing Logic
While Decagon AI agents can resolve 70% to 90% of customer inquiries without human intervention, Intelligent Routing Logic ensures that the 10% to 30% of inquiries unsuitable for AI agent resolution are directed to humans. This mechanism determines whether the AI agent can handle a customer inquiry or if it should be delivered to a human agent for manual resolution. Even when the agent cannot complete a request, a quality customer experience is maintained as it defaults back to the human-in-the-loop, who can then answer the customer’s question.
Agent Assist
When a customer inquiry is elevated to a human support agent, Decagon Agent Assist makes them more productive by surfacing the most relevant documentation and information from within the AI’s knowledge base. The AI Agent is a “strategic copilot for your human agent with real-time suggested responses and insights.” Because it integrates with the customer’s ticketing platform, such as Zendesk, human agents can access Agent Assist within the platform they already use. Agent Assist does “the heavy lifting of surfacing relevant information and drafting suggested answers so [agents] can save countless hours.”
Admin Dashboard
To Decagon, its AI agents "should not be a black box," and their customers should receive full visibility into their end users' common issues. The Admin Dashboard serves as a control center, aggregating insights across customer interactions and monitoring performance. Specifically, it “automatically reviews and tags [customer] conversations” to identify recurring themes within customer inquiries” and suggests additions that should be made to the AI agent’s knowledge base so that it can more effectively resolve inquiries that it may lack context on.
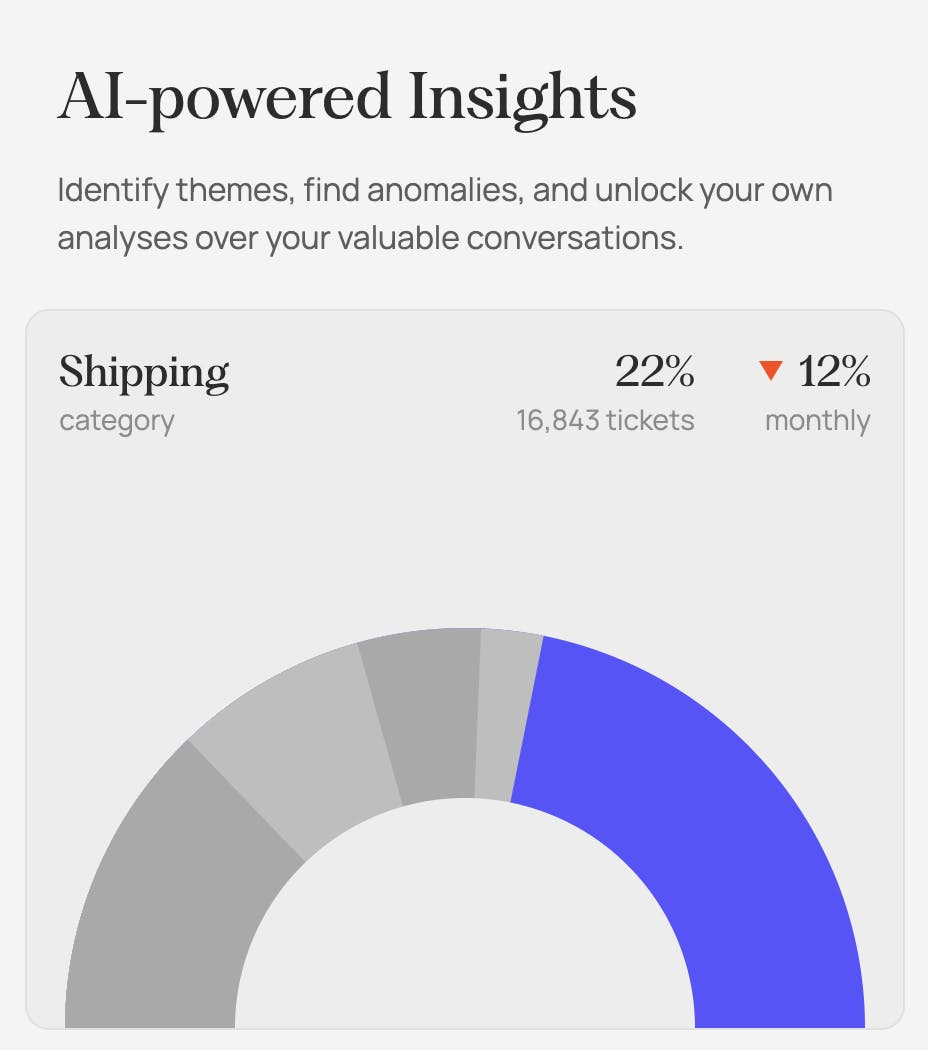
Source: Decagon
The AI agent can suggest knowledge base additions and proactively write new articles that fill knowledge gaps, which are then pulled into its knowledge base for use in future customer conversations. Further, Decagon customers can use the dashboard to quickly view the percentage of tickets resolved and average customer satisfaction score by month, along with gaining detailed voice of customer insights across different inquiry categories such as shipping, refunds, subscription questions, and more.
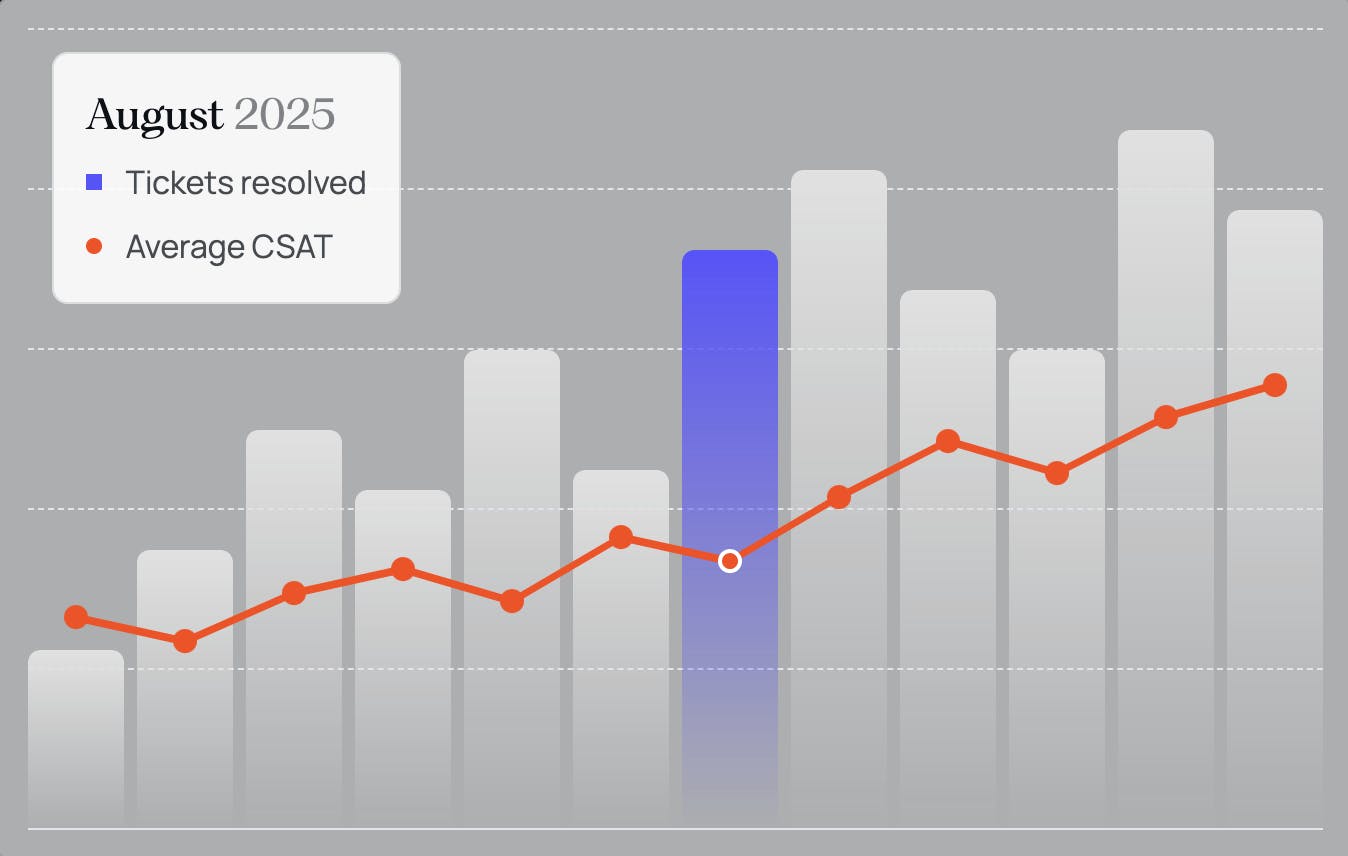
Source: Decagon
Because of the Admin Dashboard, customer experience leaders using Decagon “become uniquely proactive in their support philosophy” as they can immediately identify where customer points of confusion and frustration lie while quickly notifying human agents and updating the AI agent’s knowledge base so it can address these challenges. Sarah Vanden Broek, Senior Manager of Customer Experience Operations at ClassPass, highlighted the impact that this visibility has had on her organization:
"Though we already had a robust Voice of the Customer program and an understanding of customer inquiries we thought we could deflect, we saw 10x higher deflection at launch than we anticipated. Decagon has also allowed us to scale our Chat program to 24/7 while hitting critical CX metrics [for example, average customer satisfaction], at the same time."
Admin Dashboard also helps customer support teams bridge the gap with product teams, as direct visibility into customer pain points can easily be delivered to the product team to inform future product updates.
Quality Assurance Interface
Continuing its emphasis on providing customers with direct insights, the Quality Assurance Interface is where human support agents can see the documents and information that the AI agent uses to make decisions. Human agents can also provide direct feedback on the AI agent’s decision-making. This system “acts as [Decagon’s] continuous improvement mechanism for customer support,” learning from explicit feedback provided by human support agents to more effectively support customers in the future. Even non-technical employees can “make real-time adjustments to AI behavior,” which allows human agents to directly influence the agent’s development over time.
Decagon has emphasized that as of December 2024, “we’ve entered a fundamentally new era of AI where LLMs are capable of increasingly complex tasks, yet many **of today’s AI-powered products are still black boxes. They offer no insight into their inner processes like how different decisions are made.” This tool delivers visibility into how its agents make decisions and provides customers with direct input on how agents learn continuously over time.
Market
Customer
Decagon almost exclusively targets large enterprises. As of February 2025, its customer base includes companies like Notion, Bilt, Rippling, Substack, Eventbrite, Curology, and Vanta. While Zhang and Sreenivas were conducting their initial customer discovery interviews, they found that “enterprise customers felt deep pain with their customer support” and that existing chatbot solutions diminished customer trust.
Standard AI chatbots commonly struggle] with complex inquiries inherent in enterprises’ customer support operations and are often unable to “interpret nuanced expressions.” In addition to incumbent solutions’ ineffectiveness, Zhang indicated that many “bigger companies were hesitant to use AI in [customer experience] due to it being a black box and not having control over its guardrails or insight into how it’s making decisions.”
Because Decagon’s first core value is that “customers are everything,” its AI Agent Engine was purpose-built for the enterprise audience. Its AI agents are able to “capture complex business logic” that feels much less scripted and more so reflects human-level intelligence. The product components surrounding the AI agents, including the Admin Dashboard and QA Interface, provide customers with tooling that gives them direct visibility and oversight into their agents — removing the ‘black box’ that caused hesitancy in adopting customer support AI.
Evolving Role of Customer Support Team
As Decagon’s AI agents are autonomously resolving about 70% to 90% of customer inquiries, the company believes that customer support roles will shift from manually fielding inquiries toward “building and managing [AI] agents” and strategically building the support function. Tasks like monitoring the AI agents, analyzing their decision-making, providing feedback to help agents learn over time, and identifying knowledge base gaps will be core to human agents’ responsibilities.
When discussing this shift in the future of customer support roles, Zhang emphasized that this shift is “our core thesis for the company. That’s why our whole product is built around giving people tooling, visibility, explainability, and control over the agents.” Decagon’s focus on ‘removing the black box’ and delivering creative ways for non-technical people to construct custom guardrails, rules, and business logic around their agents is “probably #1 thing that has set us apart so far,” Zhang says.
Customer Case Studies
Substack: Substack was founded in 2017 to “help writers monetize their work independently, offering a way to bypass traditional media gatekeepers.” Its core product is a publishing platform that allows anyone to create and distribute a newsletter publication directly to their audience of both free and subscribed readers. As of December 2024, the company has scaled its subscription network to over 35 million active newsletter subscriptions and over 3 million paid subscriptions.
The company initially built its own in-house chatbot, yet this solution proved ineffective as Substack’s human support consistently fielded repetitive inquiries from customers, including subscription cancellations. Further, the in-house bot provided no analytics or insights on customer inquiry conversations and did not personalize its responses based on the type of customer it was interacting with (publisher, subscriber, or free user).
Substack adopted Decagon’s AI Agent Engine, which solved each of these issues. The AI agent has helped Substack “resolve more than 90% of user questions without human intervention, leading to consistently high [average customer satisfaction] and deflection rates.” As Decagon's AI agent is now managing repetitive customer inquiries, the support team can “now dedicate more time to helping top writers and creators with growth, marketing, and product-related inquiries” while remaining the same size.
Because Substack’s primary source of revenue is derived from a “commission fee charged to writers who monetize their content through paid subscriptions,” Decagon is directly impacting Substack’s revenue generation by providing human support staff with more time to help publishers scale their publications.
Rippling: Rippling is a cloud-based workforce management platform designed to eliminate the friction from running a business by unifying HR, IT, and finance operations on a single data platform. Founded in June 2016, the company rapidly scaled its ARR from $54 million in 2021 to $350 million in 2023. As of February 2025, Rippling has over 10K customers and over 400K total platform users.
Before using Decagon, the company used a decision-tree-based solution that required significant manual human attention and lacked the flexibility needed to support Rippling’s “ever-growing and diversified user base.” With over 12 core products spanning multiple functions (HR, IT, and finance) along with its user base holding various roles within small businesses and enterprises, Rippling is the ideal example of an enterprise with a customer support operation that is too complex for a traditional AI chatbot. Husam Najib, Vice President of Customer Support at Rippling, emphasized the challenges that the company was experiencing:
“Rippling has a very broad surface area with distinct products that require unique treatments — we brought this problem statement to Decagon and they delivered. We are able to tailor the experience and responses to customers to not only deliver strong deflection results, but also enhance the customer experience along the way.”
This level of complexity made it challenging for Rippling to provide its customers with an effective support experience. Customers could only self-serve their inquiries 38% of the time through the chat channel. Thus, improved deflection rate was a key objective for Rippling. Additionally, its diversified product portfolio and end-user base meant that its previous AI solution commonly directed customers to the wrong human support teams as it lacked a proper tagging and routing system. Rippling desired a more robust tagging and routing system to mitigate this.
After implementing Decagon’s AI agents, Rippling increased its chat inquiry resolution rate to 50% after connecting to “Rippling’s internal APIs to generate human-like responses that take into account the user’s specific situation.” For example, “if an admin is asking about an employee's healthcare enrollment status, Decagon can pull in that information from Rippling and answer that question directly with higher accuracy and no human intervention.”
Additionally, the Decagon team established 75+ customer inquiry tags and applied routing parameters that enable the AI agent to accurately and efficiently direct customers to the correct team of human subject-matter experts if it is not suited to resolve the inquiry. This resulted in an immediate 7% lift in customers being directed to the correct human support team.
Market Size
The AI agents market is projected to grow from $5.1 billion in 2024 to $47.1 billion in 2030, reflecting a 44.8% CAGR across this period. The term “AI Agent” refers to digital systems powered by generative AI “that can independently interact in a dynamic world” and take action on behalf of humans. One of the earliest and most prominent applications of LLMs and AI agents has been customer support. This is primarily due to the existing human support infrastructure already developed, and the measurable ROI that these solutions provide. What has specifically stimulated demand for AI agents within the customer support use case, as mentioned, is the dissatisfaction that enterprises experience with incumbent conversational AI chatbots in enterprises.
NVIDIA noted in November 2021 that “41% of organizations use AI-powered copilots for customer service.” Bain Capital Ventures partner Aaref Hilaly, who led Decagon’s Series B financing, emphasized the ineffectiveness of incumbent solutions:
“We already have virtual chatbots, but they don’t work well. Mainly, customers try to bypass them as quickly as possible to get to a human because their responses are canned and almost deliberately obtuse. They give you a menu of responses and if your issue falls outside of that narrow window, you’re out of luck until a real person can resolve your challenge. At best, it’s inefficient; at worst, hugely frustrating.”
As a result, the market for customer support AI agents is expanding as enterprises seek a replacement for existing chatbot products. While there is no documented market size for this space as of December 2024, the contact center intelligence market may be a good proxy. This market was valued at about $2.5 billion in 2023 and is projected to reach $4.6 billion by 2030, reflecting a 24.3% CAGR. Specifically, the “rising need for AI solutions in customer service is a major factor driving” market growth. This growth can be attributed to a few tailwinds:
Customer Expectations for Personalization
The first key driver of AI solutions in customer support is enhanced experience personalization for customers. Customer experience leaders’ top priority in a 2024 survey was “making customer experiences more personalized.” AI agents have been recognized as a technology that will aid in this goal, as “companies are going for AI agents that scale up personalized responses due to increasing complexities of customer interactions as well as the need for customized experiences.”
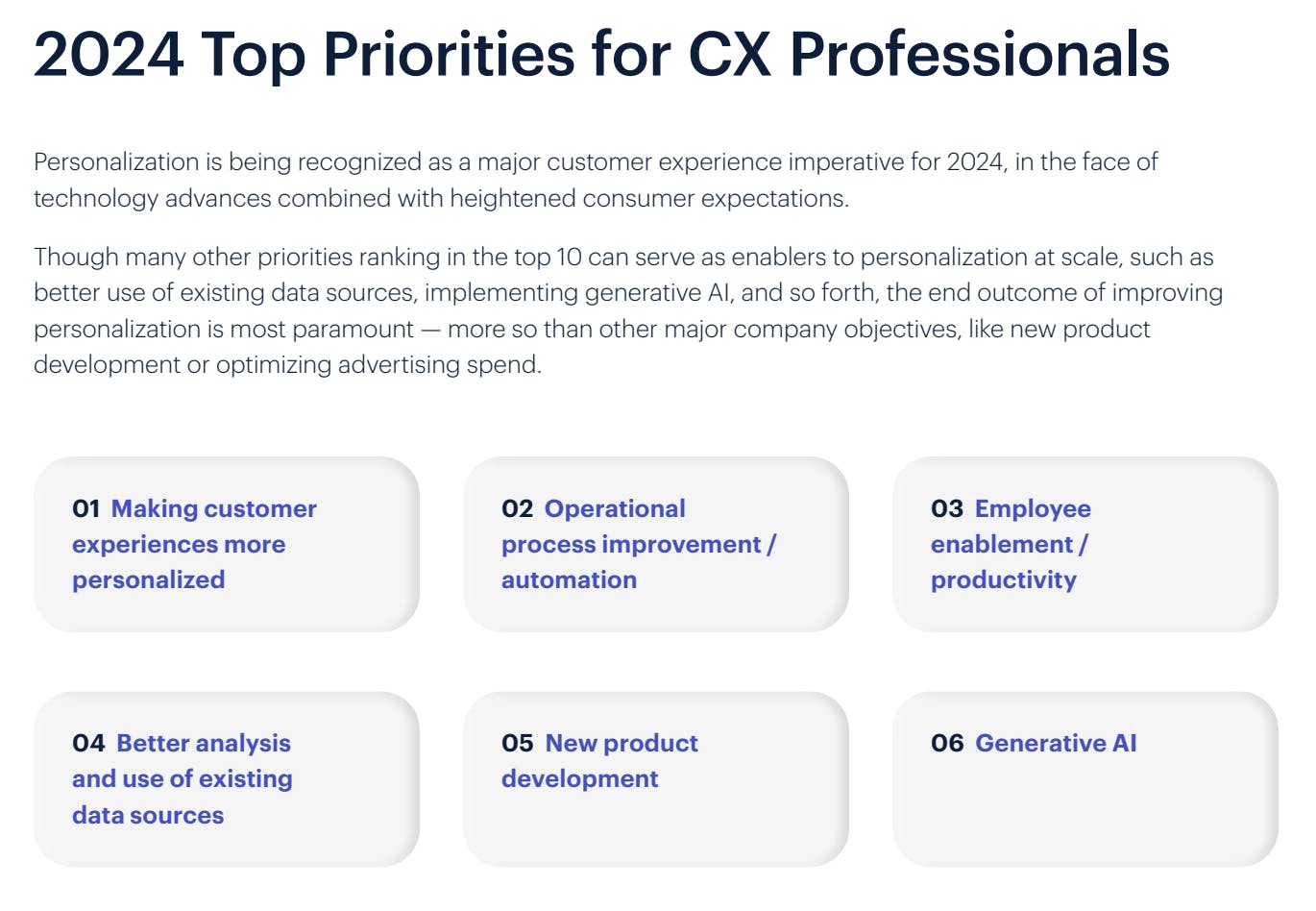
Source: Medallia
Customer experience leaders’ strong desire to elevate personalization can be attributed to the impact that delivering personalized experiences for customers has on their overall satisfaction. In the same 2024 survey, consumers who rated their experiences with a company as “very personalized” overall satisfaction was rated at 94%. For consumers who did not have a personalized experience, the overall satisfaction is reduced to 65%.
As AI customer support agents can “analyze customer history — including behavior and preferences,” they can deliver “personalized support that feels human.” Sreenivas emphasized the more personalized experience that AI customer support agents offer, stating that “for consumers, the impact will be transformative. Everyone will have access to hyper-personalized, on-demand support - even at 2 AM on Christmas morning.”
More Effective Use of Human Labor
As of August 2022, Gartner found that human labor expenses “can represent up to 95% of [total] contact center costs.” The 24/7 ‘always-on’ support that AI agents provide — autonomously resolving 70% to 90% of customer inquiries — means that enterprises can “cut contact center costs while increasing [the] scale” of their customer support operations. At the same time, customers can better utilize their most valuable resource: people.
For example, Curology used Decagon’s AI agents to “reduce customer support operation costs by 65%.” This was made possible as Decagon’s solution improved Curology’s ability to autonomously handle customer inquiries. Curology’s previous solution only resolved “5% of total support tickets.” Replacing it with Decagon’s AI agent elevated this to “80% of ticket volume.” As AI agents can “handle common questions and repetitive tasks,” enterprises are turning to them so that human support agents can develop more authentic relationships with customers and focus on initiatives that add to “overall revenue generation.”
Competition
Because of its extreme competitive saturation, Zhang and Sreenivas were initially encouraged not to enter this space. However, Zhang mentioned that while this may be the case on the surface, “we see more ‘fancy demos’ riding the AI hype than products that can truly win the trust of an enterprise company.” Large companies wanted a product that could truly handle complex, multi-step questions and provided direct visibility into how AI agents made decisions. It was indicated by Y Combinator in November 2024 that AI agents for customer support is a category that is “famously overcrowded,” but almost all of the companies in this space are making extremely simple, ineffective solutions that “can’t actually replace a real customer support team that does a lot of really complicated workflows.”
As of October 2024, “almost all of Decagon’s clients conducted what’s called a bake-off between its software and customer support tools from competitors like Salesforce — pitting one chatbot against another to see which one performed better and was able to resolve the most customer queries accurately.” Zhang indicated that “each time, Decagon’s AI-powered chatbot came out as a winner.”
Salesforce
Founded in 1999, Salesforce is a major provider of customer relationship management technology. Its cloud-based CRM was one of the first software products to be delivered via the cloud. The company went public in June 2004. As of February 2025, the company has a market capitalization of $327 billion.
As of May 2024, Salesforce held a 21.7% market share — the highest market share among players in the CRM market. This far exceeded other big tech players, including Microsoft (5.9%), Oracle (4.4%), and SAP (3.5%). It was the eleventh consecutive year the company had earned the top spot. Salesforce serves over 150K businesses globally, from enterprises to small businesses. Notable enterprise customers include American Express, Canvas, PayPal, Uber Eats, Unilever, Spotify, Toyota, and AWS.
In September 2024, the company released Agentforce — an added layer on the Salesforce platform that “delivers fully customizable autonomous AI agents that can connect to any enterprise data and take action across sales, service, marketing, and commerce.” The platform is natively integrated with the entire Salesforce product ecosystem, providing AI agents with extensive customer data that is used to deliver tailored customer experiences. Further, it is a horizontal solution that can be used in various industries like education, banking, and healthcare.
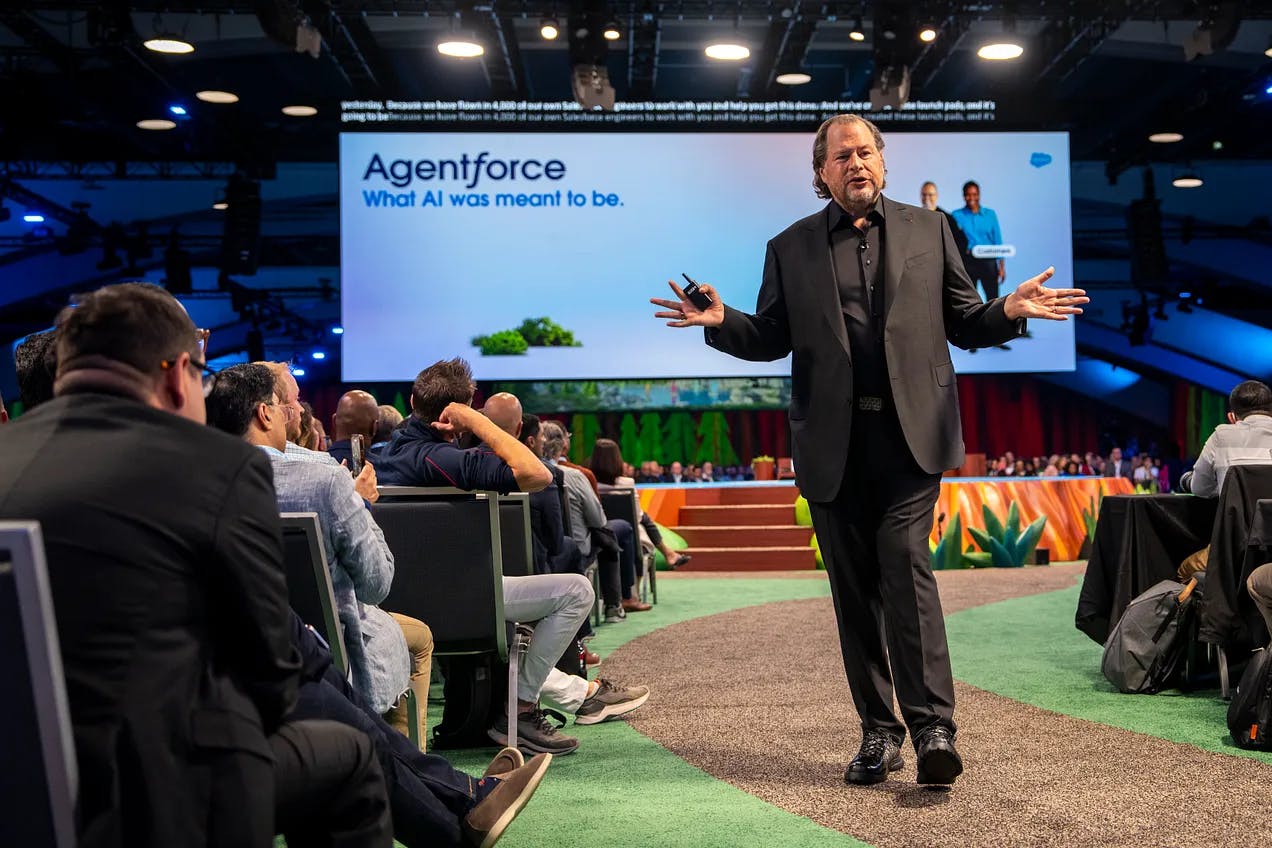
Source: Big Technology
Following the initial Agentforce release, Salesforce announced updates in December 2024 with Agentforce 2.0. While Decagon’s AI agents outperformed Agentforce’s agents before this release, the second iteration features “enhanced reasoning and data retrieval to deliver precise answers and orchestrate actions in response to complex, multi-step questions.” Because of this, Salesforce agents can handle a wider variety of user interactions than before, including those that “require deeper thought.” For example, agents can now answer deeply ambiguous and complex questions such as “What would be the right investment vehicle for my child’s college fund based on my current income and risk preferences?”
Most similar to Decagon is the Agentforce Service Agent. It is a pre-configured agent solution that is optimized for customer support. Like Decagon’s AI Agent Engine, this offering resolves repetitive customer inquiries like order shipment inquiries, enabling human service teams to focus on more complex customer interactions.
Like Decagon, Agentforce delivers usable agents out-of-box with pre-built job instructions, its Agent Builder enables users to easily fine-tune agents within any industry or use case. In natural language, users can give agents instructions and roles they want them to assume (such as AI sales representatives). Also similar to Decagon, the AI ‘black box’ is removed as users can simulate agent responses and see exactly how it makes decisions to provide feedback as needed.
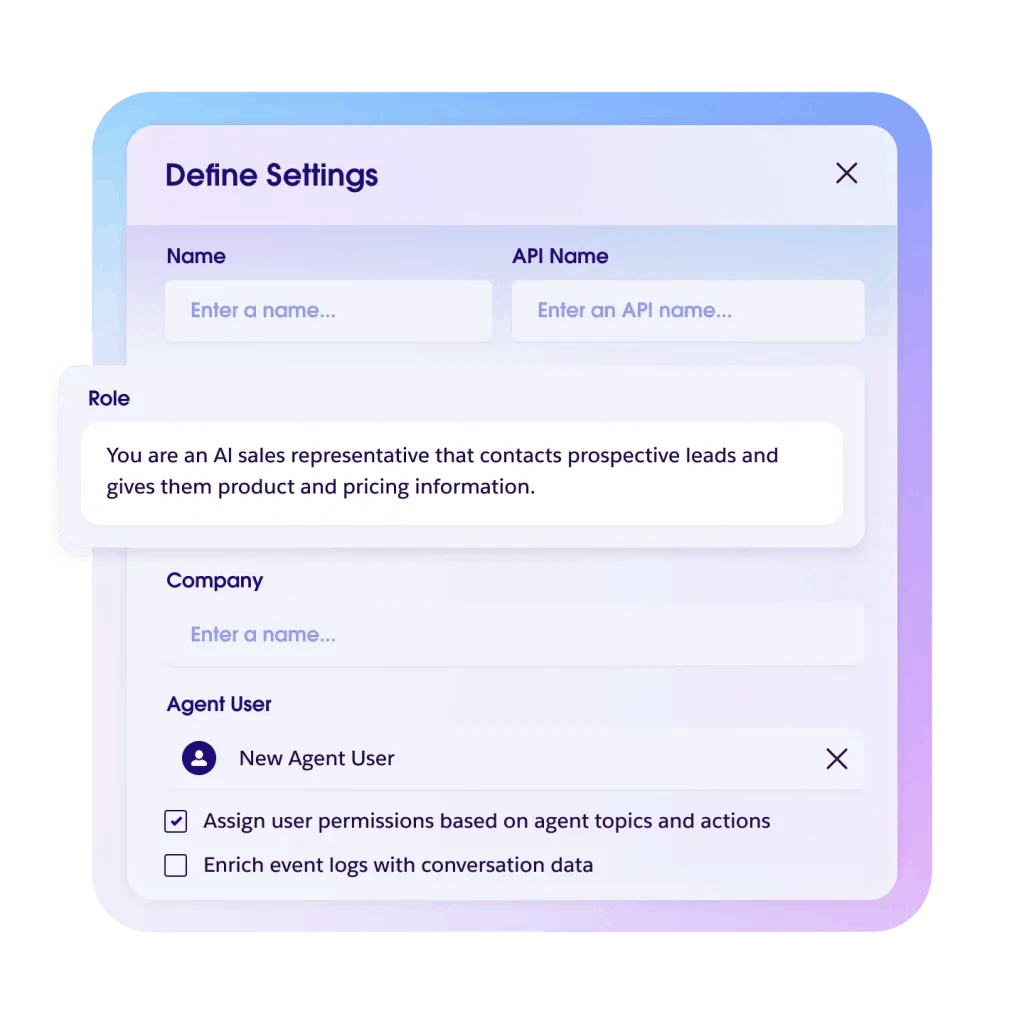
Source: Salesforce
Overall, Agentforce poses a competitive threat to Decagon due to the enhanced reasoning capabilities delivered in Agentforce 2.0, its versatility beyond customer support use cases, including sales and marketing, the simplicity of agent fine-tuning for specific domains and use cases, and Salesforce’s large enterprise customer base that already relies on Salesforce’s product ecosystem.
Ada
Ada was founded in 2016 and offers an “AI-powered platform designed to automate customer service interactions to improve customer satisfaction and reduce the workload on human customer service professionals.” In May 2021, Ada raised $130 million in Series C financing at a $1.2 billion valuation. The round was led by Spark Capital, with participation from new investor Tiger Global Management and existing investors, including Accel and Bessemer Venture Partners. The fresh capital brought Ada’s total funding to $200 million. As of February 2025, Ada has powered over 4 billion automated customer interactions and engaged with over 400 million users globally. The company’s customer base includes notable enterprises such as Monday.com, Pinterest, Square, Afterpay, Intuit Mailchimp, Verizon, and Yeti as of February 2025.
In November 2023, Ada launched its AI Agent, powered by its proprietary Reasoning Engine. Like Decagon, its Reasoning Engine uses a “sophisticated ensemble” of LLMs, “each specifically selected to optimize performance for specific tasks.” The solution is “powering experiences that are meeting — and in some cases exceeding — human capability, with each AI Agent doing the work of 10+ human agents for the cost of less than one.” As of May 2024, the company’s AI Agent was resolving over 70% of customer inquiries without involving human support.
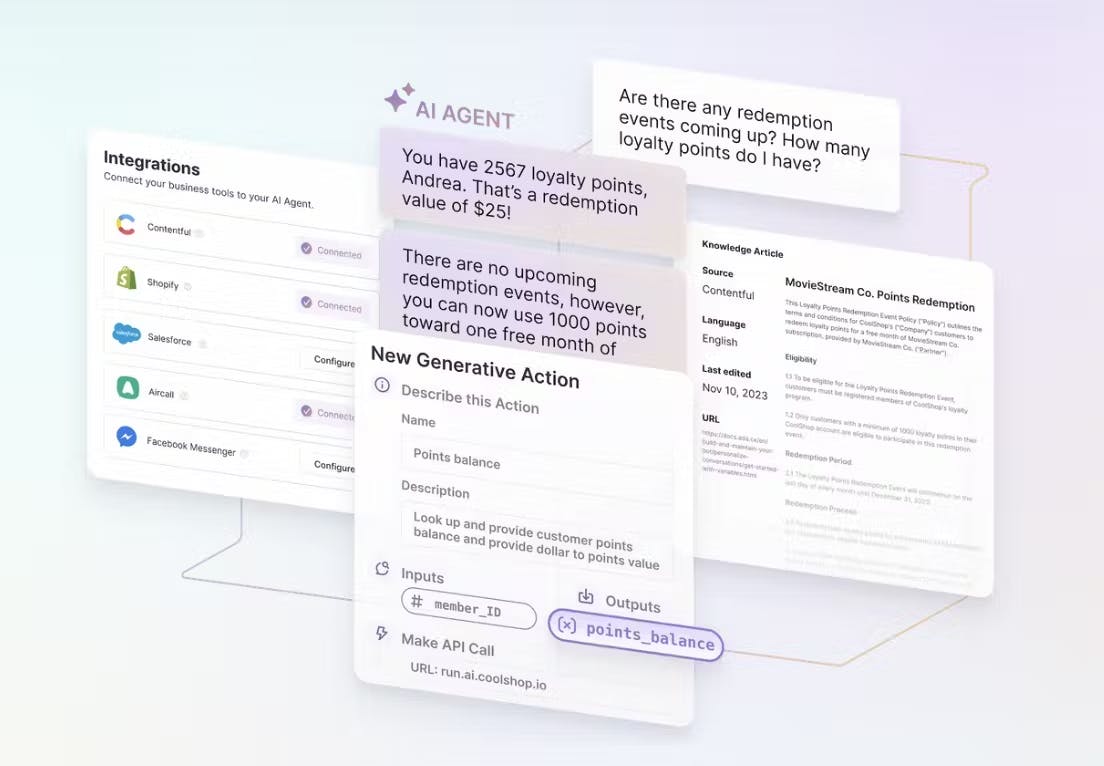
Source: Ada
Many overlaps exist between its AI Agent and Decagon’s AI Agent Engine. First, as mentioned, they both use unique combinations of LLMs in their Reasoning Engine, which enables Ada to solve highly complex, multi-step customer inquiries. Additionally, both companies’ agents take feedback and learn from it over time.
Third, in May 2024, Ada released new coaching tools that enable users to “understand what’s going on under the hood [of the Reasoning Engine] so they can see how agents make decisions and provide feedback where needed. This is very similar to Decagon’s Quality Assurance Interface which allows users to see exactly how agents make their decisions. Fourth, Ada’s AI Agent also aggregates data, which can sent to customers’ downstream business intelligence tools using the Data Export API. his contrasts with Decagon, as Decagon’s customer conversation insights surface directly within its product’s Admin Dashboard.
Intercom
Intercom was founded in 2011. The company was founded out of the “desire to make internet business personal. People wanted to connect with businesses, to receive more than spammy emails or vague, cookie-cutter answers irrelevant to their needs.” Its core product is a unified AI system for customer service. In March 2018, the company raised a $125 million Series D round led by Kleiner Perkins at a post-money valuation of $1.3 billion. Google Ventures also participated in the round. In total, Intercom has raised $240 million.
Intercom reportedly primarily serves small businesses and startups as well as mid-market companies. The company’s “original growth strategy focused on publishing content for software founders,” which caused many of its early adopters to be Y Combinator-backed startups. As of February 2025, startups receive a 90% discount on Intercom’s AI-first customer service platform. However, Intercom also serves enterprise customers, including Atlassian, Amazon, and Microsoft.
Within Intercom’s customer communication platform, support teams can manage and automatically respond to customer interactions across channels, including live chat, email, and in-app messaging from within a single platform. The company’s AI-powered customer support platform features its AI agent, Fin 2, which was released in October 2024 and is the successor to Fin, released in March 2023. Like Decagon and many other agentic products, Fin 2 can learn about the customer’s product by connecting to internal and external knowledge sources.
There are a few enhancements with Fin 2 over its predecessor. First, it can combine knowledge from multiple sources for hyper-personalized answers. It can categorize customer interactions and respond in specific ways that the user determines. For example, suppose the customer is inquiring about cancellations. In that case, the agent categorizes this as a cancellation inquiry and can direct the customer to the human team that is most well-suited to handle cancellation inquiries should the agent not resolve the request. Fin 2 can also take action on end users’ behalf, like “changing a customer’s shipping address or adjusting their subscription [tier].”
Also, similarly to Decagon, Intercom customers have complete visibility across their human and AI customer interactions. They can view analytics dashboards for key metrics and specific inquiry topics needing additional attention.
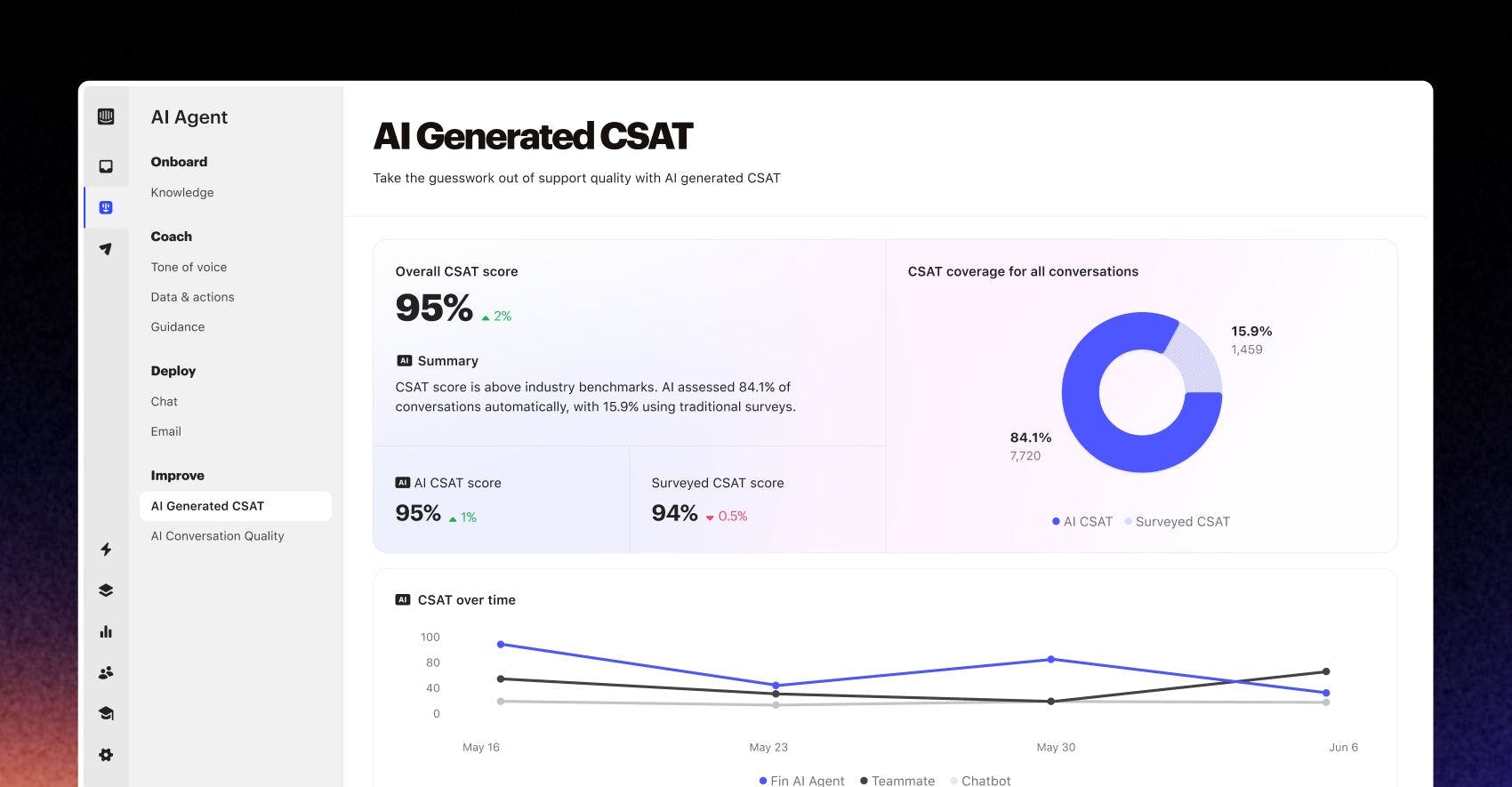
Source: Intercom
Despite these product enhancements, customers commonly achieve a 50% inquiry resolution rate, much lower than Decagon’s AI Agent Engine. This reduced resolution rate indicates Fin 2 is less effective at resolving complex, multi-step enterprise inquiries than Decagon and Ada. Intercom predominantly targets a simpler, smaller customer profile — small businesses and startups.
Maven AGI
Maven AGI was founded in July 2023 by executives from HubSpot, Google, and Stripe. Its core product, Agent Maven, is a conversational AI agent designed specifically for customer support. In May 2024, the company raised a $28 million Series A round and emerged from stealth mode. M13 led the round with participation from Lux Capital, MIT's E14 Fund, and "executives from OpenAI, Google, HubSpot, and Stripe."
Like Decagon, Maven AGI released a complete, integrated product suite surrounding its core AI agent, Agent Maven. First, Agent Maven can resolve up to 93% of customer inquiries autonomously and can take action on behalf of the customer across 50+ native platform integrations including Salesforce, Zendesk, ADP, and HubSpot. For example, TripAdvisor has adopted Agent Maven and “has seen best-in-class 90%+ resolution of support tickets while simultaneously cutting costs by 81%.” Other early adopters of Maven include HubSpot, ClickUp, Rho, and “fast-growing SMBs.” It can be deployed across text, email, web, app, and voice channels. Further, it asks “intelligent follow-up questions” as Decagon’s agent does.
Also similar to Decagon’s Agent Assist, which surfaces the most relevant insights for human agents as they respond to customer inquiries, Maven AGI has built Maven Copilot, which has the same function while also “teaching Agent Maven how to handle similar cases in the future.”
Maven AGI customers can also use Agent Designer, like Decagon’s AI Engine, to train their agents on structured and unstructured knowledge and set custom actions that the agent will employ autonomously to solve customer problems. Decagon also offers this tooling for customers as they can add custom guardrails and their business logic to their Decagon agents.
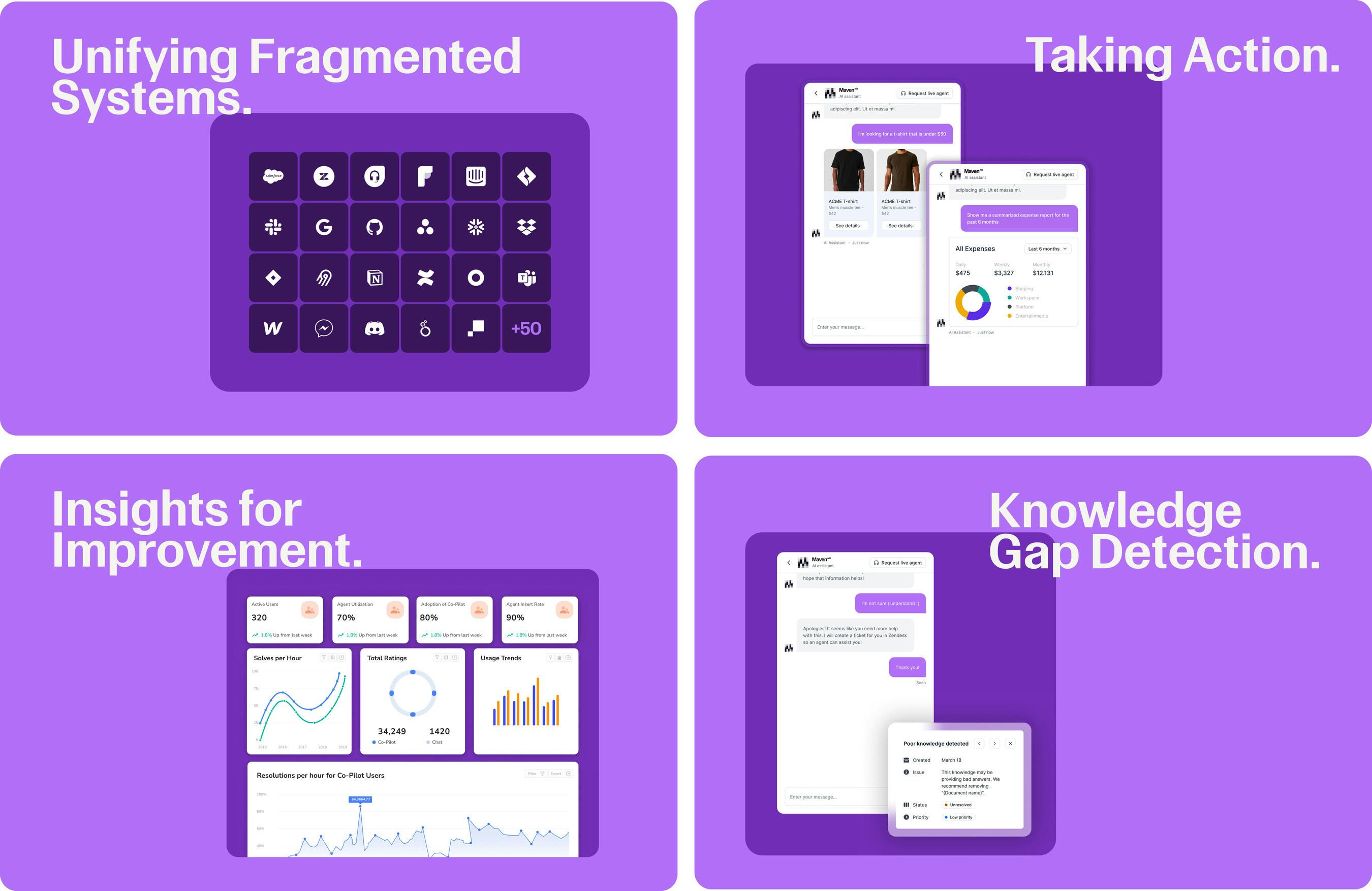
Source: PR Web
Lastly, similar to Decagon’s Admin Dashboard in which users can review insights across customer conversations and provide feedback to the agent, Maven’s Agent Ops products enable customers to “see customer sentiment, AI and human agent performance, ROI” and can also be used for human agents to “catch and resolve knowledge gaps.”
Overall, Maven AGI offers a product ecosystem very similar to Decagon’s, with an AI agent that has achieved similarly strong inquiry resolution rates. Decagon is only available in chat channels like Slack, email, web, and mobile app channels, while Maven AGI also offers voice agent capabilities.
Ema
Ema was founded in 2023 but emerged from stealth in March 2024. Contrary to Decagon’s vertical use case focus in customer support, Ema’s mission is to transform every business “with universal AI employees.” Ema, an acronym for “Enterprise Machine Assistant,” offers an AI agent that has numerous applications across customer support, employee experience, sales & marketing, and legal & compliance.
Central to the product’s functionality is the Generative Workflow Engine (GWE), which can be thought of as the “brain” powering the agents. It coordinates “the activities of specialized agents much like how the prefrontal cortex manages various brain regions.” This mirrors the human brain’s ability to complete complex challenges. The company has also built its proprietary EmaFusion model, which contains over 2 trillion parameters across “100+ public LLMs and domain-specific custom models to maximize accuracy at the lowest possible cost.”
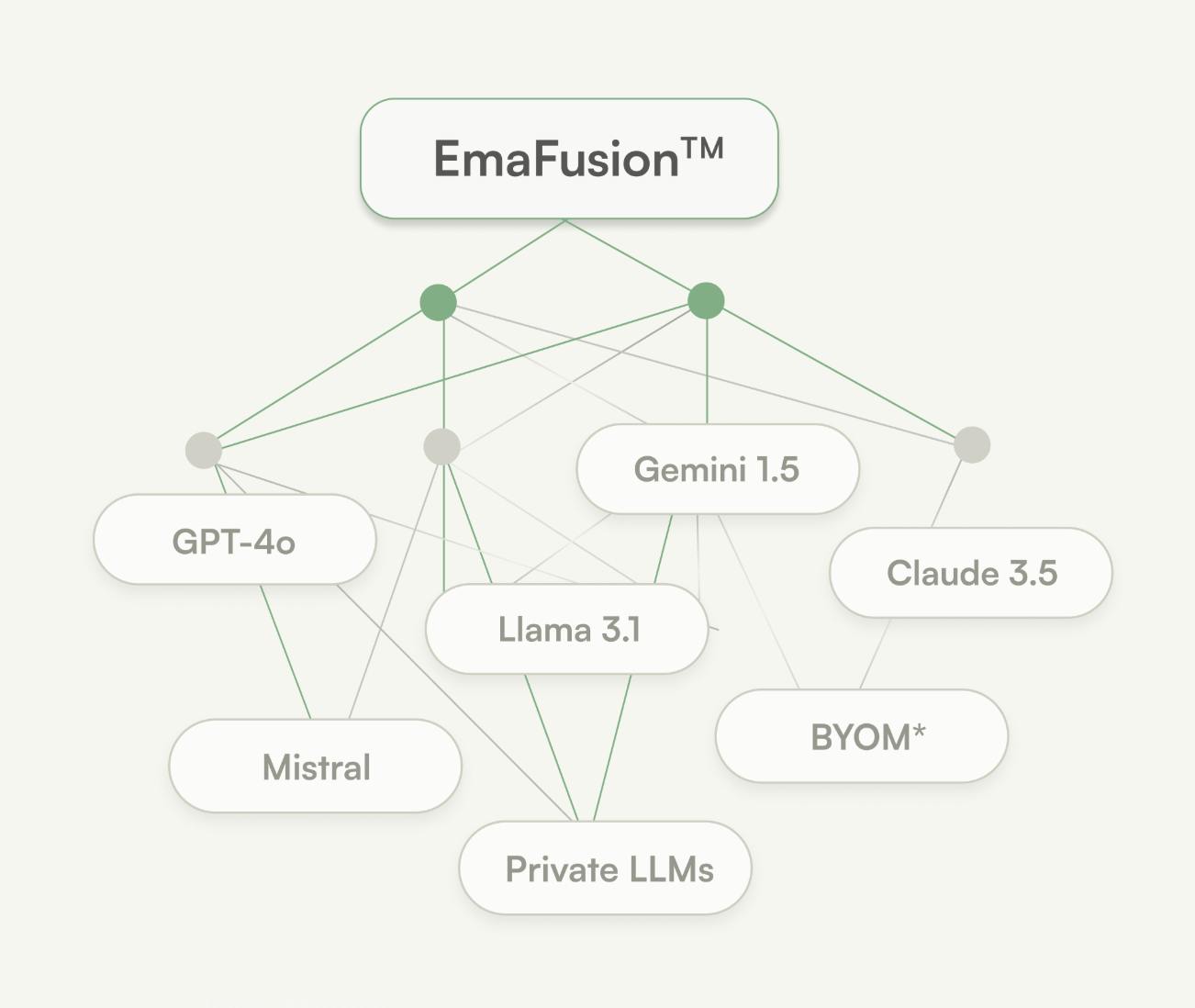
Source: Ema
While users have a pre-built library of AI employee “personas,” these templates can be easily fine-tuned to meet the customer’s specific requirements. For example, customers can fine-tune customer service specialists, data engineers, sales assistants, compliance analysts, and proposal managers with Ema.
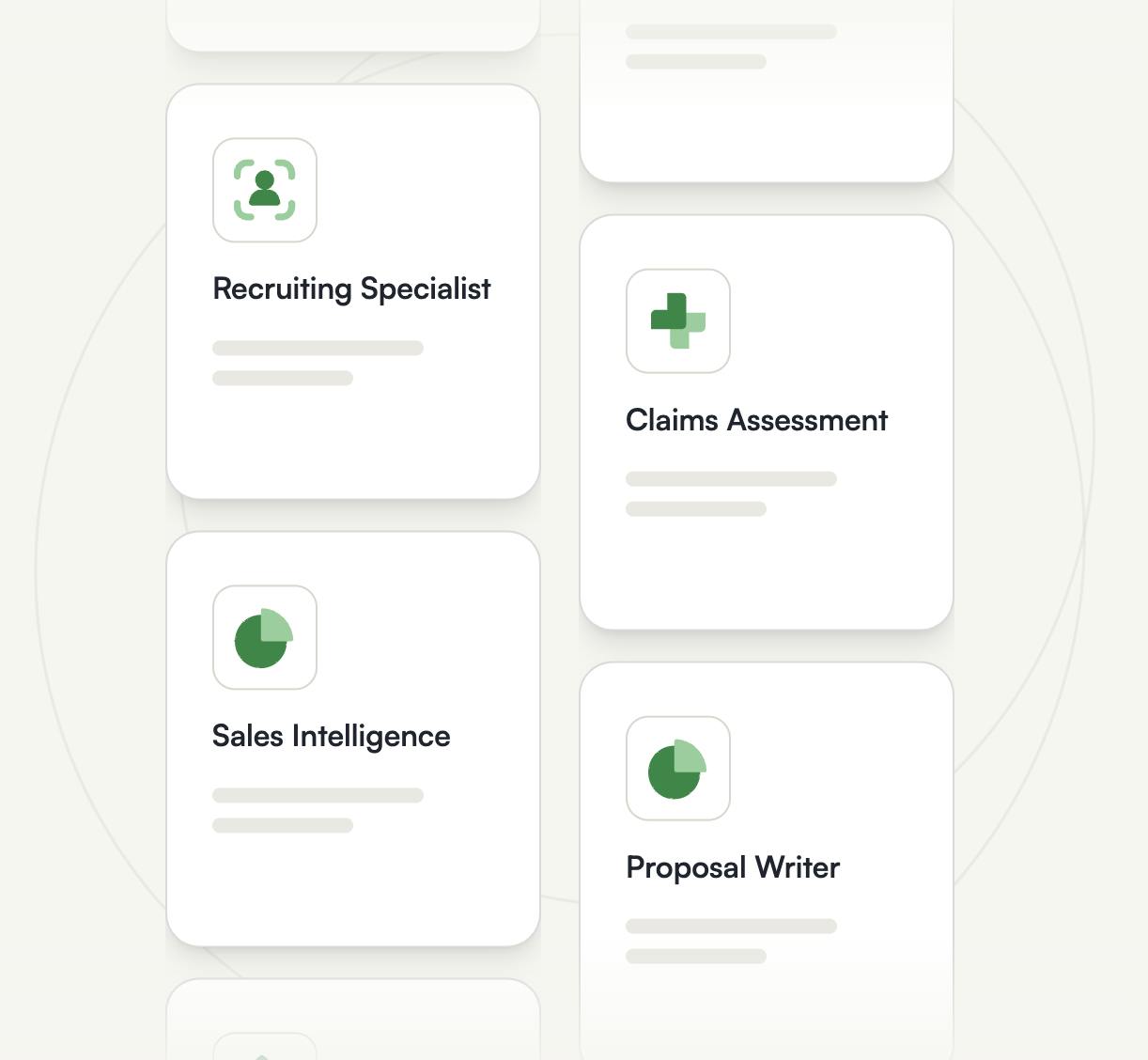
Source: Ema
As it emerged from stealth in March 2024, the company announced its initial $25 million financing co-led by Accel, Section 32, and Prosus Ventures. Notable angel investors include Jerry Yang, Sheryl Sandberg, Dustin Moskovitz, Divesh Makan, and David Baszucki. Similar to Decagon, Ema announced its $36 million Series A just a few months after its initial financing, in July 2024. Its total capital raised increased to $61 million in a round that was co-led by existing investors Accel and Section 32.
What has given investors confidence in the nascent company’s ability to provide highly performant AI employees across not just one, but many applications is the pedigree of the founding team. CEO Surojit Chatterjee was formerly Chief Product Officer at Coinbase prior to its IPO and was also VP of Product at Google in its mobile ads and shopping segments. Engineering Lead Souvik Sen was formerly VP of Engineering at Okta and, before this, was an ML Engineering Lead at Google focused on privacy and data. Between them, they hold 77 patents as of March 2024.
As of July 2024, Chatterjee noted that Ema’s “customer base “has tripled since it emerged from stealth in March 2024 — across sectors such as finance, legal, healthcare, e-commerce, and insurance.” Overall, the strength of Ema’s founding team has given investors confidence in their ability to provide a quality enterprise AI agent employee across numerous functional areas (including customer support). If they are able to succeed, this may be a notable threat to Decagon’s business as enterprises may seek to consolidate their tech stack across fewer vendors.
Sierra
Founded in 2023, Sierra was founded by Bret Taylor and Clay Bavor to deliver human-like, empathetic AI agents for customer support that complete actions on behalf of customers. As of February 2025, Taylor serves as Chairman of the Board at OpenAI and previously led Salesforce as co-CEO alongside Marc Benioff. He also led the Board at Twitter when it was acquired by Elon Musk and has widely been credited with leading the team that co-created Google Maps. Bavor spent 18 years at Google, leading VR initiatives and serving as VP of Google Labs.
In October 2024, it was announced that Sierra had raised $175 million at a $4.5 billion valuation. The Series A financing was led by Greenoaks Capital with participation from ICONIQ and Thrive Capital. The Series A followed the company’s $110 million seed round led by Sequoia Capital and Benchmark at a near $1 billion valuation. As a result, the company has $285 million in total capital.
Sierra’s conversational AI platform allows enterprises to create autonomous, personalized AI agents that are purpose-built for customer support. Its Agent OS platform enables customers to easily customize and deploy agents that possess a fine-tuned personality and tone of voice. Also, agents can be instructed to “help customers with even their most complex issues, whether making an exchange or updating a subscription.”
Similarly to Decagon’s Admin Dashboard, Sierra tracks, tags, and measures each customer conversation so customer experience teams can uncover insights and make adjustments. However, one differentiation from Decagon is Sierra’s hyper-realistic voice agent. It displays the “nuances of human communication” and rapid response times while employing the specified personality and tone of voice that the customer selects.
As of February 2025, enterprise customers, including Sonos, Casper, ADT, and Weight Watchers, have adopted Sierra’s conversational AI agent platform. Resolution rates for these customers seem to fall around 70% as of February 2025. While Decagon’s AI Agent Engine has displayed higher resolution rates of 90%, Sierra AI’s use of hyper-realistic voice makes it a channel that could help Decagon maintain differentiation.
Business Model
Because AI agents automate a portion of humans’ work, the number of humans required to do the same amount of work is reduced. This means that the standard seat-based SaaS model doesn’t translate well to agent-based products as the value incurred by AI agent solutions does not scale alongside the number of people using it. Instead, customers incur value from agentic products based on specific outcomes or product usage. Just like a human worker, as more work is done by the AI agent, more value is generated.
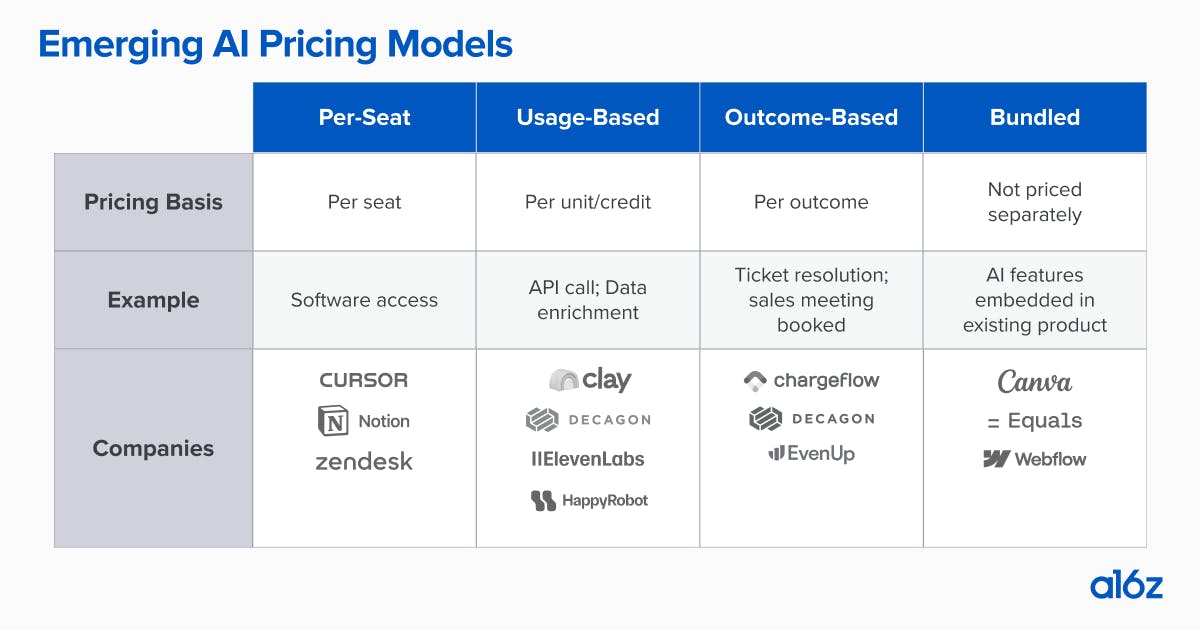
Source: a16z
Decagon is no exception and as of December 2024, offers two pricing options. First, the per-conversation model charges a fixed rate for each conversation that end users conduct with Decagon’s AI agents. Second, the per-resolution model charges a higher fixed unit rate — only for conversations in which the customer’s inquiry is autonomously resolved. If the inquiry is elevated to a human agent, the customer does not pay for the interaction. For both models, standard volume discounts apply.
As of December 2024, the “majority of [Decagon’s] customers gravitate toward” the per-conversation pricing model. The primary reason for this is that “costs scale directly with usage.” As the number of interactions the AI agent has with customers increases, the cost scales linearly. Customers like the predictability of this model.
Traction
As of February 2025, Decagon serves a wide variety of “forward-thinking” enterprises spanning B2B and B2C. B2C companies like Eventbrite, Curology, Bilt, and ClassPass have adopted Decagon, while enterprise software companies like Vanta, Duolingo, Webflow, and Rippling are using Decagon’s AI agents on the B2B side. Decagon’s AI agent platform appeals to enterprise customers due to its ability to accurately resolve complex customer inquiries without “sacrificing quality or speed,” leading to cost savings and a “white-glove experience” for end users.
Additionally, enterprises value the suite of tooling they receive across all customer interactions. This enables them to review granular insights across all customer interactions, see how the agent is making decisions, and a no-code platform to customize the guardrails and business logic of the agent while giving direct feedback so it can improve continuously.
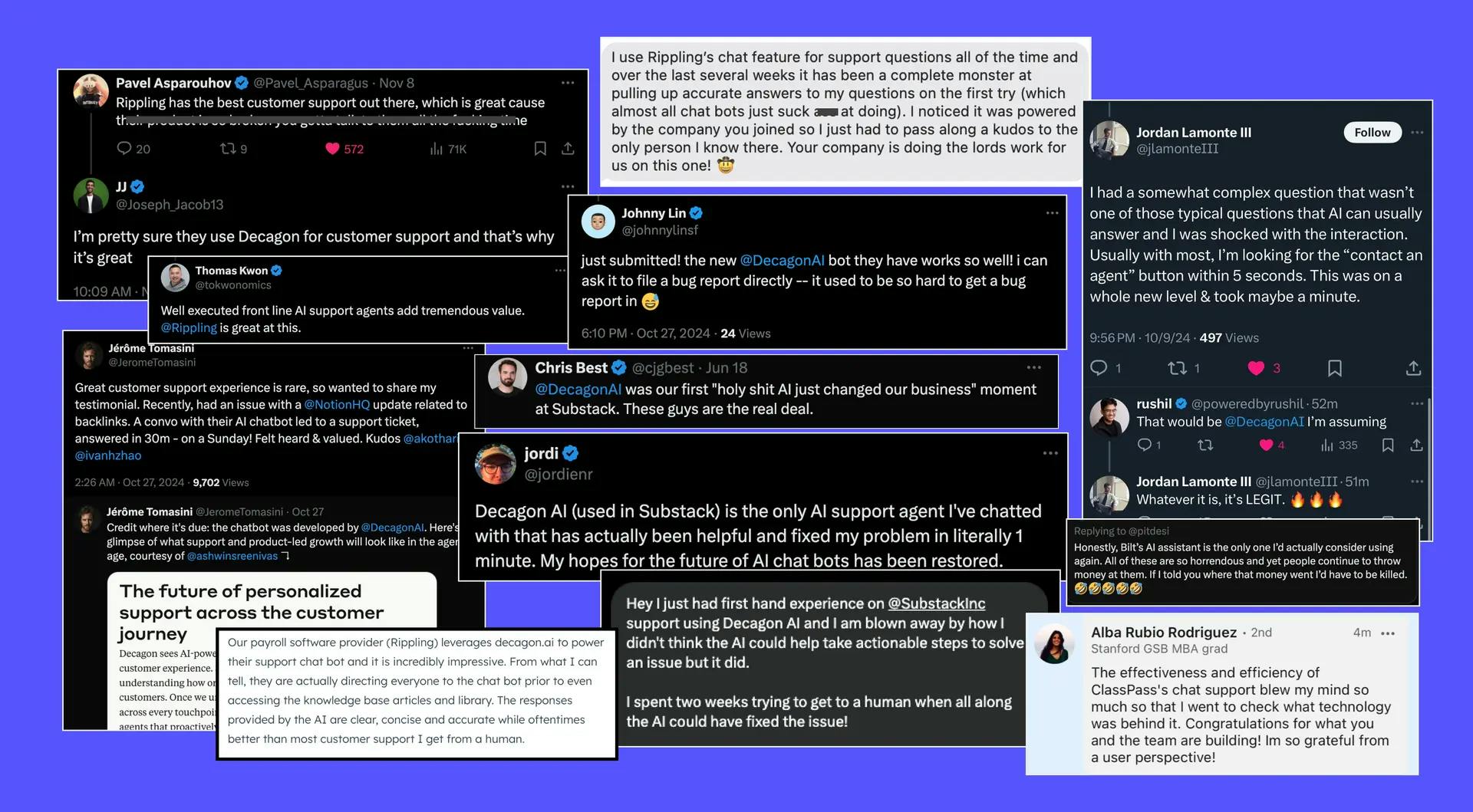
Source: Decagon
This early adoption has helped Decagon scale revenue with a limited team. Zhang stated that as of June 2024, “we reached seven-figures ARR with two people while building everything from scratch.” As of February 2025, the company has 55 employees. Sreenivas noted that when evaluating potential candidates, “Decagon looks for proof of exceptionalism. Either a candidate has built something really cool themselves or has worked at a company we admire.” Because Decagon has achieved product-market fit and is focused on revenue scale, thirteen employees are aligned to sales and go-to-market as of February 2025.
Valuation
In October 2024, Decagon announced that it had raised a $65 million Series B financing led by Bain Capital Ventures (BCV), bringing its total amount raised to $100 million. BCV Partner Aaref Hilaly led the round and joined the Decagon Board. Other investors in the round included Accel, Elad Gil, A*, BOND Capital, and ACME Capital. While a valuation has not been publicly disclosed as of February 2025, it was noted that Decagon quadrupled its valuation in the few months between its Series B and June 2024 Series A.
Just a few months prior to the Series B, in June 2024, Decagon had emerged from stealth. The company announced that it had raised $35 million spread across a $30 million Series A and a $5 million seed round. The Series A was led by Accel with participation from Elad Gil, A*, and numerous high-profile angel investors, including Aaron Levie (CEO of Box), Howie Liu (CEO of Airtable), Matt MacInnis (COO of Rippling), Jack Altman (co-founder and former CEO of Lattice).
Lead investor Ivan Zhou, who represented Accel on the deal and joined the Decagon Board, has a personal connection to the founding team. Prior to joining Accel, he founded Mayhem, a “social gaming platform” that provided “AI insights and analytics for gamers.” It was acquired by Niantic in January 2021, upon which Zhou joined the company as Director of Product Management and led augmented reality experiences across the Niantic product ecosystem. He also supported Niantic Ventures and led the acquisition of Zhang’s first company, Lowkey, in December 2021.
The seed round was led by Andreessen Horowitz who had invested at company inception after also backing Lowkey before it was acquired by Niantic. Investment Partner Kimberly Tan represented the firm and joined Decagon’s Board after having introduced the pair at a 2023 founder retreat in Utah.
Key Opportunities
Expansion Beyond Customer Support
In November 2024, Decagon Product Lead Bihan Jiang noted that the company envisions its AI Agent Engine as “the endgame for all AI agent systems — customer support is just the beginning.” This comes as AI agents are being used for multiple use cases across customer-facing and internal employee domains. For example, on the customer-facing side, Decagon could expand its offerings into sales prospecting and tailored outreach. Agent startups like Artisan, Outreach, Unify, and Clay offer AI products to automate end-to-end sales processes, from prospect research to tailored outbound automation. Additionally, sales is a natural use case for Salesforce’s Agentforce product as it is natively integrated with the company’s CRM platform.
On the internal employee side, Decagon’s existing solution could translate well as an internal employee copilot that quickly and accurately retrieves information from across an enterprise’s knowledge base while also taking action on behalf of employees. The Agent Assist feature already fulfills this role for customer support agents — meaning it could provide the same value for other internal functions as well.
As of February 2025, Decagon’s customer base includes enterprises spanning D2C physical goods companies like Curology, B2C software companies like Substack and Duolingo, and B2B enterprise software vendors like Rippling and Vanta. Because all three of these customer profiles have gained value from using Decagon, the broad compatibility of Decagon’s offering is a key opportunity for continued revenue expansion.
New AI Modality
Apart from chat, website, and mobile app, Decagon could expand its product to include voice functionality to support phone calls with end customers. Zhang indicated that voice is “still in its early stages and is a technology that we’re super excited about,” and that the technology isn’t quite ready for enterprise-grade usage. Although Decagon competitors, including Sierra and Cognigy, feature conversational voice agents, Zhang stated that “you’ll be able to see a lot of demos now where the voice latency feels really nice, but that’s when you have, for example, a single prompt [and not many complex, multi-step questions].”
This may work for small businesses, but when you have highly complex, multi-component inquiries that enterprises often face, voice agents must be able to deliver accurate answers with minimal time gaps between responses. Should Decagon elect to buy voice technology from another vendor and integrate it with its existing AI gent Engine infrastructure, Cartesia is building Sonic: “the fastest, ultra-realistic generative voice AI,” with customer support as a primary use case. Overall, adding voice AI would enable Decagon to add phone calls as a new channel through which customers could interact with the AI agent.
Personal Customer Concierge
In his October 2024 Decagon investment spotlight, Aaref Hilaly noted the opportunity for Decagon this way:
“Imagine that each customer has an agent that acts as a personalized concierge, bringing them into the storefront and showing them products tailored to their past history. This super-powered agent would know them by name at first contact, make helpful suggestions and proactively solve their problems. Every store would be able to offer Four Seasons-level service that would engender loyalty on a new scale.”
The elevated personalization that Decagon provides end users aligns with customer experience leaders’ top priority of providing tailored, personalized customer experiences. This is an imperative for leaders as personalization plays a significant role in customer satisfaction.
Apart from Decagon providing more personalization compared to traditional chatbots, there could be an opportunity for this “personalized concierge,” as Hilaly notes, to ecommerce. Given that 81% of “customers prefer companies that offer a personalized experience” as of April 2024, online retailers are seeking innovative ways to enhance shopper experience in online channels. Decagon developing a ‘personal shopping concierge’ that functions much like an in-person sales associate, who learns from past conversations with a specific customer over time, could deliver this enhanced customer experience.
For example, the AI agent could learn the customer’s style preferences, shopping history, personality, and even price sensitivity and could provide item recommendations that drive increased basket sizes. Given that Decagon has already worked with notable B2C online retailers like Curology, providing a personal online shopping concierge could be an effective way to expand its usage footprint with end customers while enhancing stickiness with retailers.
Key Risks
Crowded Market
Although it was indicated in November 2024 that Decagon believes it can apply its core AI Agent Engine to other use cases beyond customer support, competitors, including Salesforce and Ema, offer horizontal use case solutions spanning sales, marketing, compliance, and HR use cases. This may be a threat to Decagon as enterprises may elect to consolidate their agent stack across one or a few vendors due to data security concerns.
More specifically, the Generative AI customer support category is extremely popular as technologies within this space enable enterprises to reduce labor costs — which comprises 95% of total contact center spend as of August 2022. As of June 2024, Zhang was “well aware of how stiff the competition is in the market for AI-powered customer support, which spans not only tech giants like Google and Amazon but [many] startups” as well. Decagon’s comprehensive AI Agent Engine — which “removes the black box” around AI agent decision-making and can support highly complex customer inquiries while giving customers greater input and visibility on AI agent improvement over time — is also similarly offered by competitors.
For example, Maven AGI offers an integrated product suite that surrounds its core AI agent product — which autonomously resolves up to 93% of inquiries — and enables customers to see how agents make decisions while giving direct feedback so they can improve future performance. Other startups, like Sierra, offer voice modality which Decagon lacks as of February 2025. Large tech companies like Salesforce, Microsoft, and Google have each released agent products that integrate with their respective product ecosystems as of February 2025. Overall, while Decagon has supposedly scaled revenue quickly, continual product innovation across new AI modalities and use cases could help the company maintain this growth over time.
Summary
Enterprises have highly complex customer support operations with often diverse product offerings and end-user bases. To reduce the burden of doing mundane, repetitive tasks on their human support agents, enterprises adopted conversational AI chatbot technology. However, large companies “felt deep pain with their customer support,” as incumbent chatbot solutions were unable to solve the deeply nuanced, multi-step inquiries that customers often pose which “eroded customer trust.”
In a February 2023 study, nearly 75% of respondents reported that chatbots “aren’t able to handle complex questions and are often unable to provide accurate answers.” Further, a December 2022 study found over 80% of applicants had reported that chatbots “increased their frustration levels,” while 63% indicated that their chatbot interaction did not resolve their inquiry.
Decagon provides an AI agent purpose-built for enterprise customer support that displays human-like reasoning capability to provide accurate responses to nuanced, multi-step customer inquiries. Surrounding the AI agent itself is robust tooling that unlocks precise visibility and explainability into how agents are making their decisions while enabling all employees, regardless of technical background, to construct custom rules, guardrails, and business logic around the agents. As of February 2025, the company serves enterprise B2B and B2C customers, including Rippling, Substack, Vanta, Bilt, Curology, and Duolingo. While Decagon achieved product-market fit quickly — having reached a seven-figure ARR with a two-person team as of June 2024 — the key question is whether it can sustain this growth within a deeply fragmented market.